Leveraging Established Machine Learning Methodology to Tackle Viral Infections in Emergency Departments: A Retrospective Analysis using Hemocytometric Data to detect SARS-CoV-2
Research Square (Research Square)(2023)
摘要
During the COVID-19 pandemic, the healthcare system faced significant strain. This study aimed to achieve two objectives: developing a machine learning model using only haemocytometric data to accurately identify COVID-19 positive patients in Emergency Departments (EDs) and empowering non-technical healthcare professionals to assess model performance and make informed decisions about implementing machine learning models in healthcare settings. This study utilized data from suspected COVID-19 admissions at Zuyderland Medical Center and employed principal component analysis, agglomerative hierarchical clustering, followed by Random Forest to build and evaluate the prediction model. Model validation was conducted using a separate dataset. The resulting model, based on 14 haemocytometric parameters, achieved an overall weighted accuracy of 0.88, with specificity, sensitivity, positive predictive values, and negative predictive values of 0.93, 0.78, 0.86, and 0.89, respectively. Concentrations of highly fluorescent lymphocytes, basophils, and eosinophils had the most significant impact on the model's output. This study demonstrates the potential of a well-trained machine learning model to predict COVID-19 infection within one hour using easily accessible laboratory data. Implementing this model in Emergency Departments could provide valuable support and alleviate staff workload.
更多查看译文
关键词
tackle viral infections,established machine learning methodology,emergency departments,hemocytometric data,sars-cov
AI 理解论文
溯源树
样例
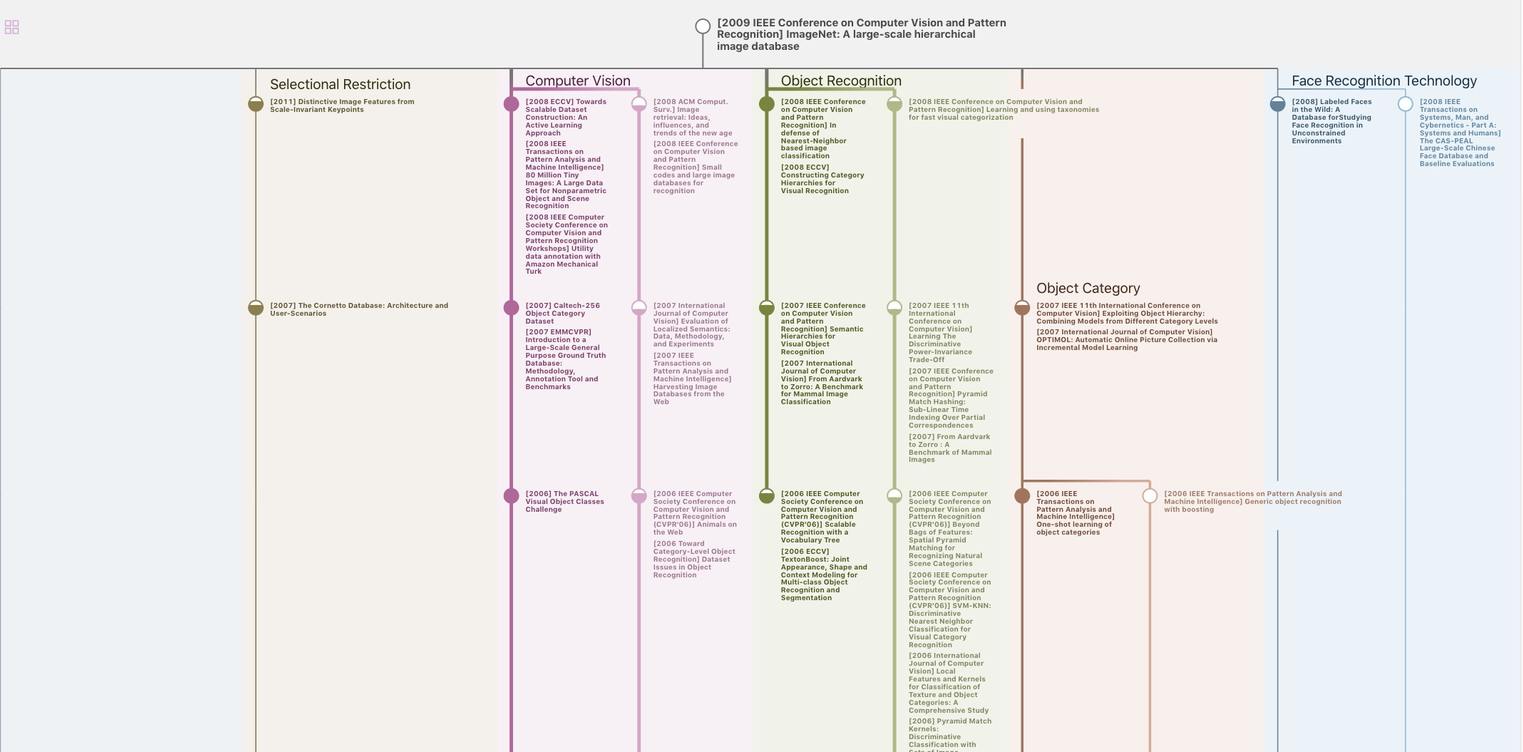
生成溯源树,研究论文发展脉络
Chat Paper
正在生成论文摘要