Metrics for Evaluating Machine Learning Models Prediction Accuracy and Uncertainty
Computer-aided chemical engineering(2023)
摘要
Models with stochastic outputs are commonly employed to make decisions for design and operation of chemical processes. The accuracy and precision of the stochastic outputs, i.e., model predictions, should be compared to the observation based on the design and operation requirements. The area metric measures the overall mismatch between prediction and observation but does provide precision and accuracy information separately. In this paper, we proposed a new metric called uncertainty width that decomposes area metrics into precision and bias components. This new metric enables the consideration of precision and accuracy simultaneously for model evaluation. We applied this metric to evaluate and compare the performance of three models fitted using three liquid entrainment fraction measurement datasets with different flow orientations, using Gaussian Process (GP) and Bayesian Neural Network (BNN). The results reveal that the overall prediction accuracy of GP model (GPM) is better than that of BNN model. The overestimation of the GPM prediction precision causes the high average area metric.
更多查看译文
关键词
accuracy,machine learning,prediction,uncertainty
AI 理解论文
溯源树
样例
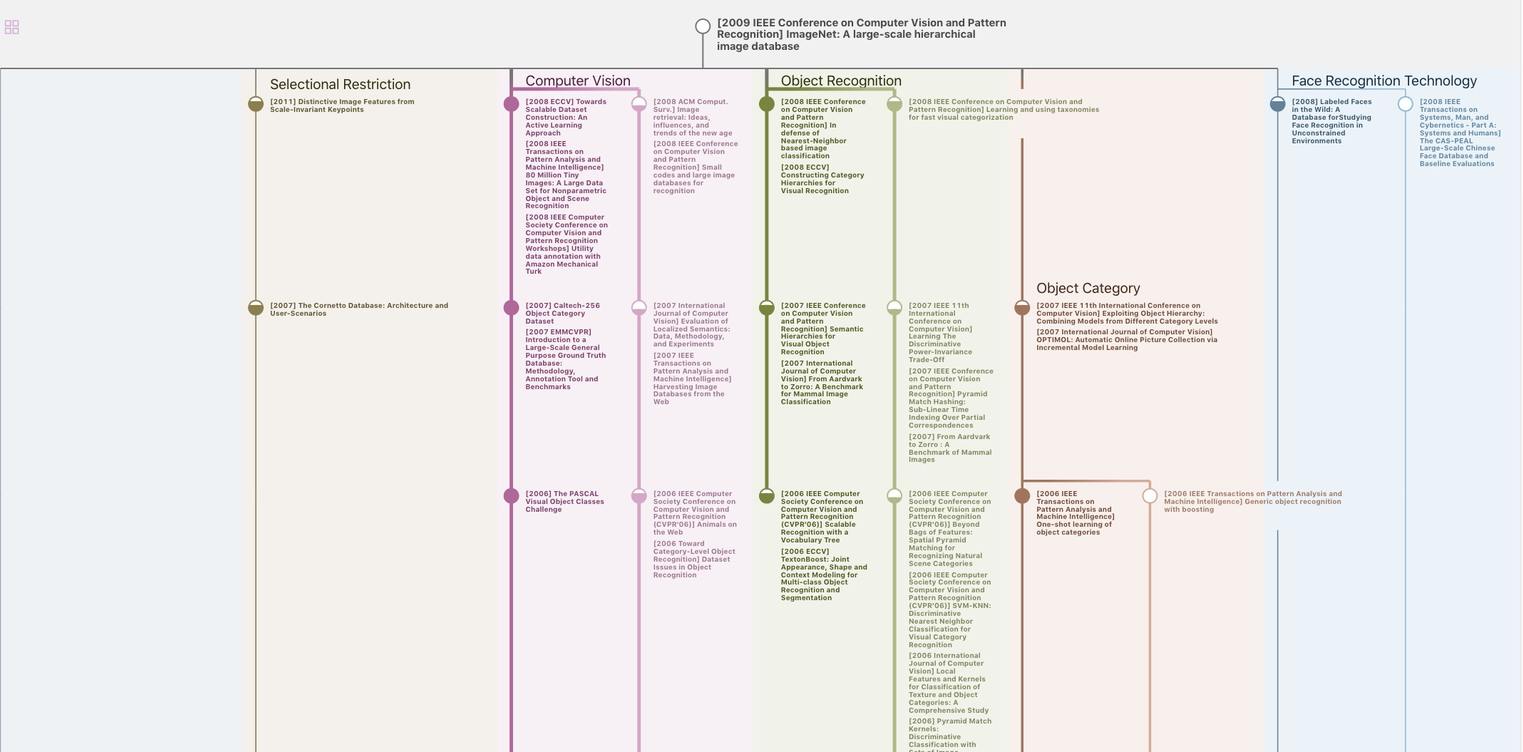
生成溯源树,研究论文发展脉络
Chat Paper
正在生成论文摘要