Fuzzy Twin Support Vector Machines With Distribution Inputs
IEEE TRANSACTIONS ON FUZZY SYSTEMS(2024)
摘要
The fuzzy twin support vector machine (FTSVM) is a powerful and effective classifier due to the use of nonparallel hyperplanes and fuzzy membership functions. This article extends the FTSVM model to uncertain objects with probability distributions and proposes a distribution-input FTSVM (DFTSVM) model. Unlike the classical FTSVM, the DFTSVM model adopts an insensitive pinball loss function that can suppress feature noise. It also defines a fuzzy membership function of uncertain objects in terms of the Wasserstein distance and k-nearest neighbors. We use properties of Gaussian distributions to transform the original DFTSVM into a tractable model. When covariance matrices of uncertain objects are positive definite, two optimization problems in DFTSVM are smooth and convex, and the quasi-Newton algorithm is employed to solve the DFTSVM model. We also analyze the DFTSVM model to demonstrate its noise insensitivity and weighted scatter minimization. The fuzzy decision rule we define is independent of Gaussian assumptions, and a kernel version of DFTSVM via a reduced-set strategy is achieved. In the experimental part, we demonstrate the effectiveness of DFTSVM when handling uncertain objects. Moreover, we illustrate how the DFTSVM model is modified to deal with large-scale datasets by constructing probability density functions of samples and how to model deep features as distribution inputs for image datasets.
更多查看译文
关键词
Support vector machines,Data models,Optimization,Kernel,Covariance matrices,Computational modeling,Probability distribution,Data classification,fuzzy decision rules,fuzzy membership,fuzzy twin support vector machine (FTSVM),kernel functions
AI 理解论文
溯源树
样例
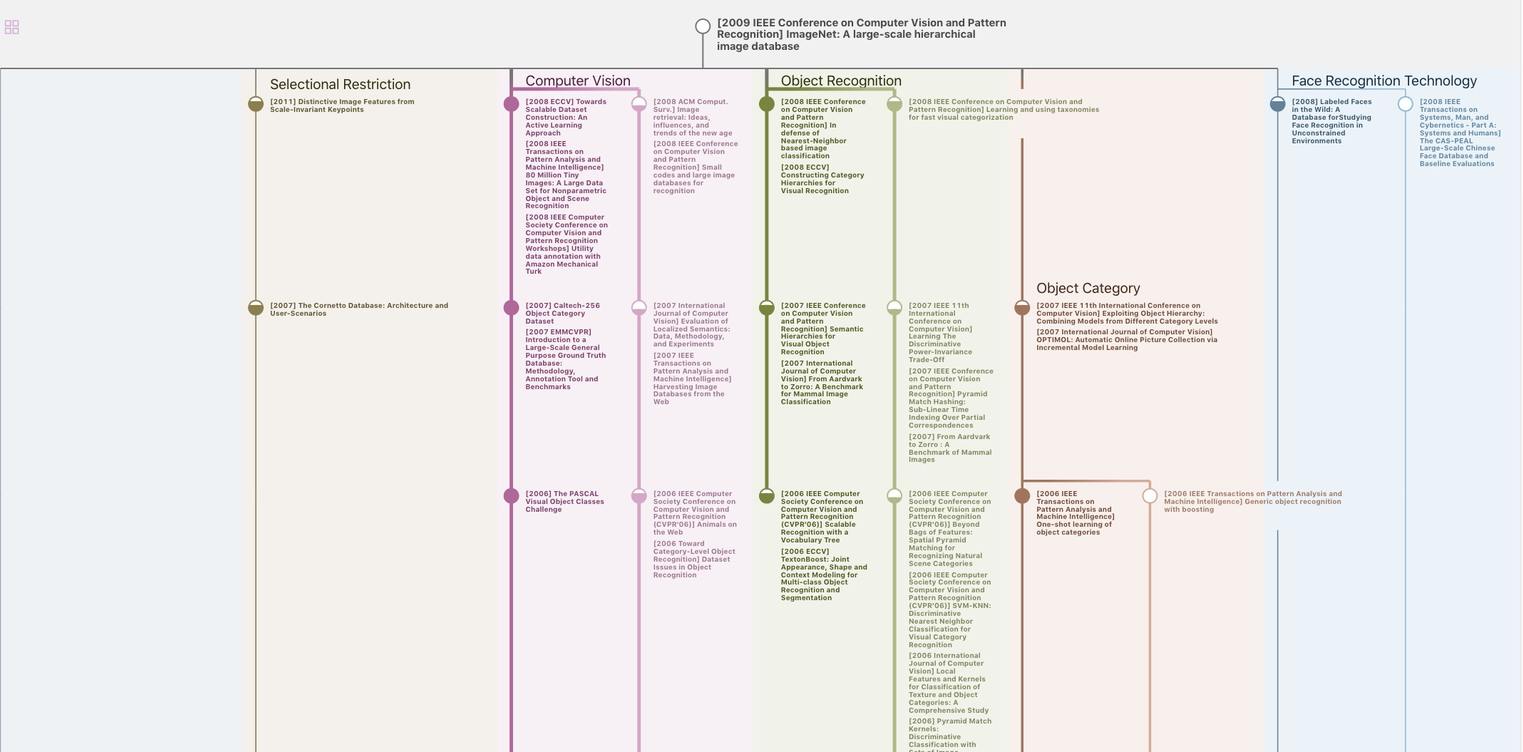
生成溯源树,研究论文发展脉络
Chat Paper
正在生成论文摘要