MALIBO: Meta-learning for Likelihood-free Bayesian Optimization
arXiv (Cornell University)(2023)
摘要
Bayesian optimization (BO) is a popular method to optimize costly black-box functions. While traditional BO optimizes each new target task from scratch, meta-learning has emerged as a way to leverage knowledge from related tasks to optimize new tasks faster. However, existing meta-learning BO methods rely on surrogate models that suffer from scalability issues and are sensitive to observations with different scales and noise types across tasks. Moreover, they often overlook the uncertainty associated with task similarity. This leads to unreliable task adaptation when only limited observations are obtained or when the new tasks differ significantly from the related tasks. To address these limitations, we propose a novel meta-learning BO approach that bypasses the surrogate model and directly learns the utility of queries across tasks. Our method explicitly models task uncertainty and includes an auxiliary model to enable robust adaptation to new tasks. Extensive experiments show that our method demonstrates strong anytime performance and outperforms state-of-the-art meta-learning BO methods in various benchmarks.
更多查看译文
关键词
optimization,malibo,meta-learning,likelihood-free
AI 理解论文
溯源树
样例
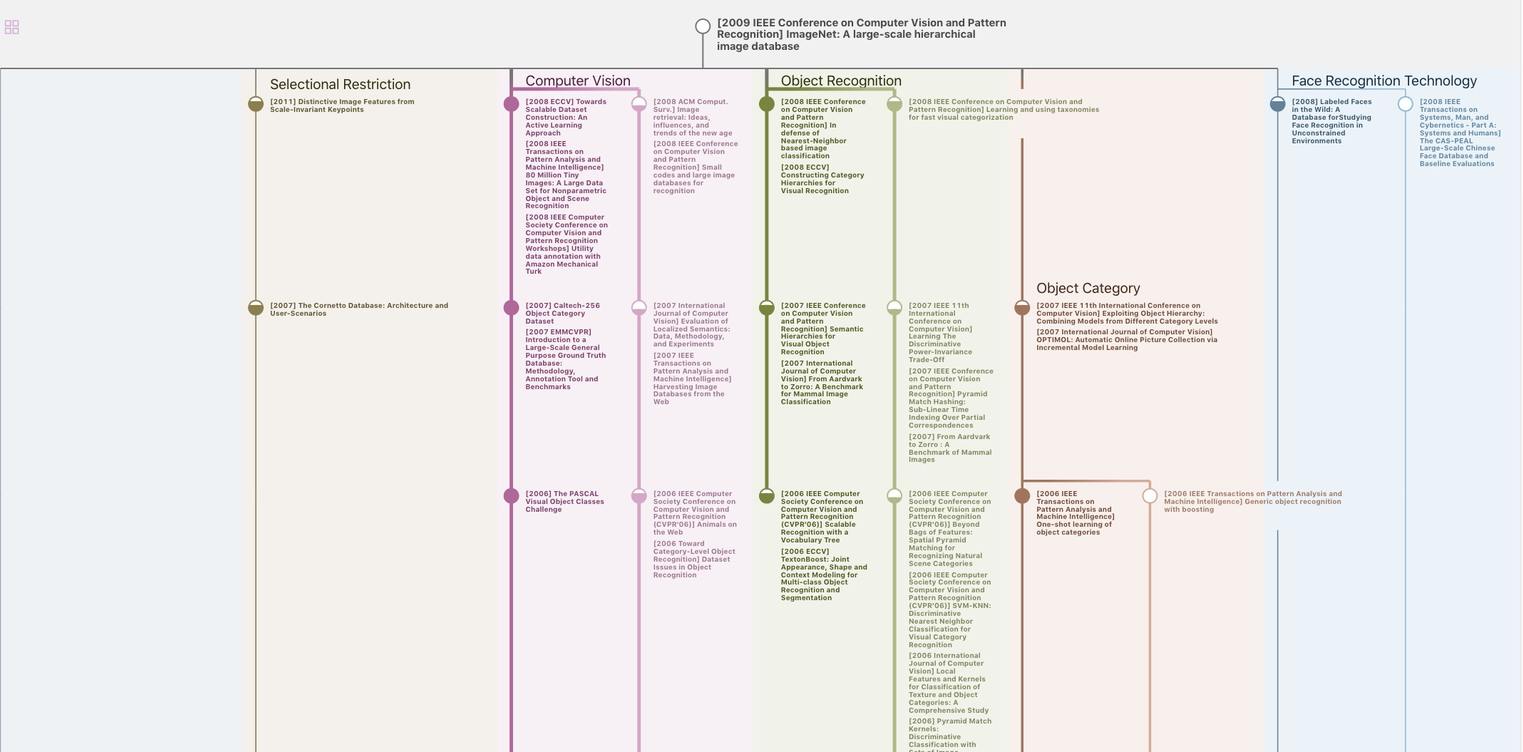
生成溯源树,研究论文发展脉络
Chat Paper
正在生成论文摘要