DeHi: A Decoupled Hierarchical Architecture for Unaligned Ground-to-Aerial Geo-Localization
IEEE TRANSACTIONS ON CIRCUITS AND SYSTEMS FOR VIDEO TECHNOLOGY(2024)
摘要
Ground-to-aerial (G2A) geo-localization remains extremely challenging due to the drastic appearance and geometry differences between ground and aerial views, especially when their relative orientation is unknown. In this paper, we focus on the challenging problem of unaligned G2A geo-localization, where the query ground-level image is not perfectly orientation-aligned with respect to reference aerial imagery. We cast this problem as a metric embedding task and propose a decoupled hierarchical (DeHi) architecture to progressively learn meaningful multi-grained features. Specifically, DeHi first leverages CNN to extract high-level semantic features, and then introduces a novel orthogonally factorized transformer model consisting of part-level and global transformer encoders to learn part-level and global feature descriptors sequentially. For the purpose of enhancing representation power, cross-level connections are introduced to enrich part-level and global descriptors by CNN features, and the pooled part-level descriptor is combined with the global descriptor to construct the final query representation. Furthermore, such a decoupled hierarchical architecture allows for incorporating multi-level deep supervision. We introduce two part-level losses combined with one cross-level loss to complement the widely used global retrieval loss. Extensive experiments on standard benchmark datasets show significant boosting in recall rates compared with the previous state-of-the-art. Remarkably, DeHi improves the recall rate @top-1 from 78.59% to 82.38% (+3.79%) and from 72.91% to 77.94% (+5.03%) on CVUSA and CVACT datasets, respectively, under random orientation misalignments. Besides, DeHi maintains competitive inference efficiency with less parameters compared to existing transformer-based methods.
更多查看译文
关键词
Transformers,Task analysis,Computer architecture,Feature extraction,Convolutional neural networks,Computational modeling,Sun,Unaligned cross-view geo-localization,decoupled hierarchical architecture,factorized transformer model,multi-level deep supervision
AI 理解论文
溯源树
样例
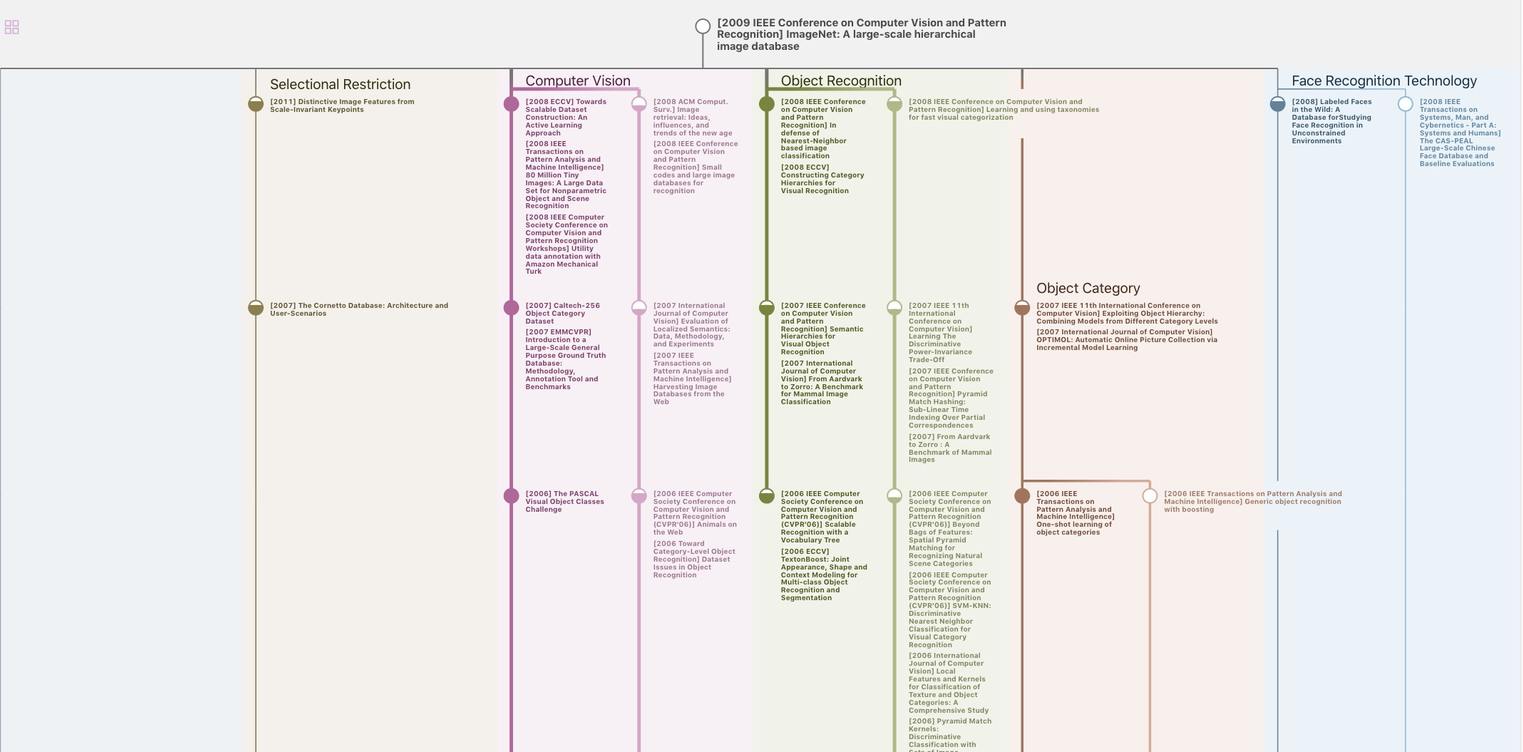
生成溯源树,研究论文发展脉络
Chat Paper
正在生成论文摘要