Analysis of follow-up data in large biobank cohorts: a review of methodology
bioRxiv (Cold Spring Harbor Laboratory)(2023)
摘要
Abstract Survival analysis in clinical trials has been extensively researched, but its applicability to large omics-based biobanks requires further investigation. This study addresses two important issues in time-to-event data analysis in biobank settings. First, there is the need to pay attention to left-truncation of some outcomes that would prevent individuals joining the biobank, if the event of interest occurs before possible recruitment time. To avoid biases due to left-truncation, it has been suggested to use age as time scale in the analysis, whereas an individual is considered at risk only after recruitment to the biobank. Second, one needs to address the computational burden due to relative slowness of the conventional algorithm to maximize the partial likelihood function for the Cox model. This study examines the impact of the choice of timescale on the bias and power, while varying effect size and censoring rate in the range realistically seen in a typical biobank setting. Moreover, the study explores a computationally fast two-step martingale residual (MR) based approach for Cox modeling in high-dimensional omics data. The findings indicate that the choice of timescale has minimal impact on accuracy for small hazard ratios (HR), but for larger HRs, accounting for left-truncation is crucial to reduce bias. When maximizing power for association discovery, using participant age as the time scale (whereas individual is considered to be at risk immediately after birth) yields the highest power. The two-step MR approach is recommended for genome-wide association studies due to acceptable precision and retained power for small effect sizes. However, for predictions and polygenic risk score calculations, effect sizes should be recalculated using the conventional Cox proportional hazards model while accounting for left-truncation. The conclusions are based on simulations and illustrated with survival data from the Estonian Biobank cohort.
更多查看译文
关键词
large biobank cohorts,data
AI 理解论文
溯源树
样例
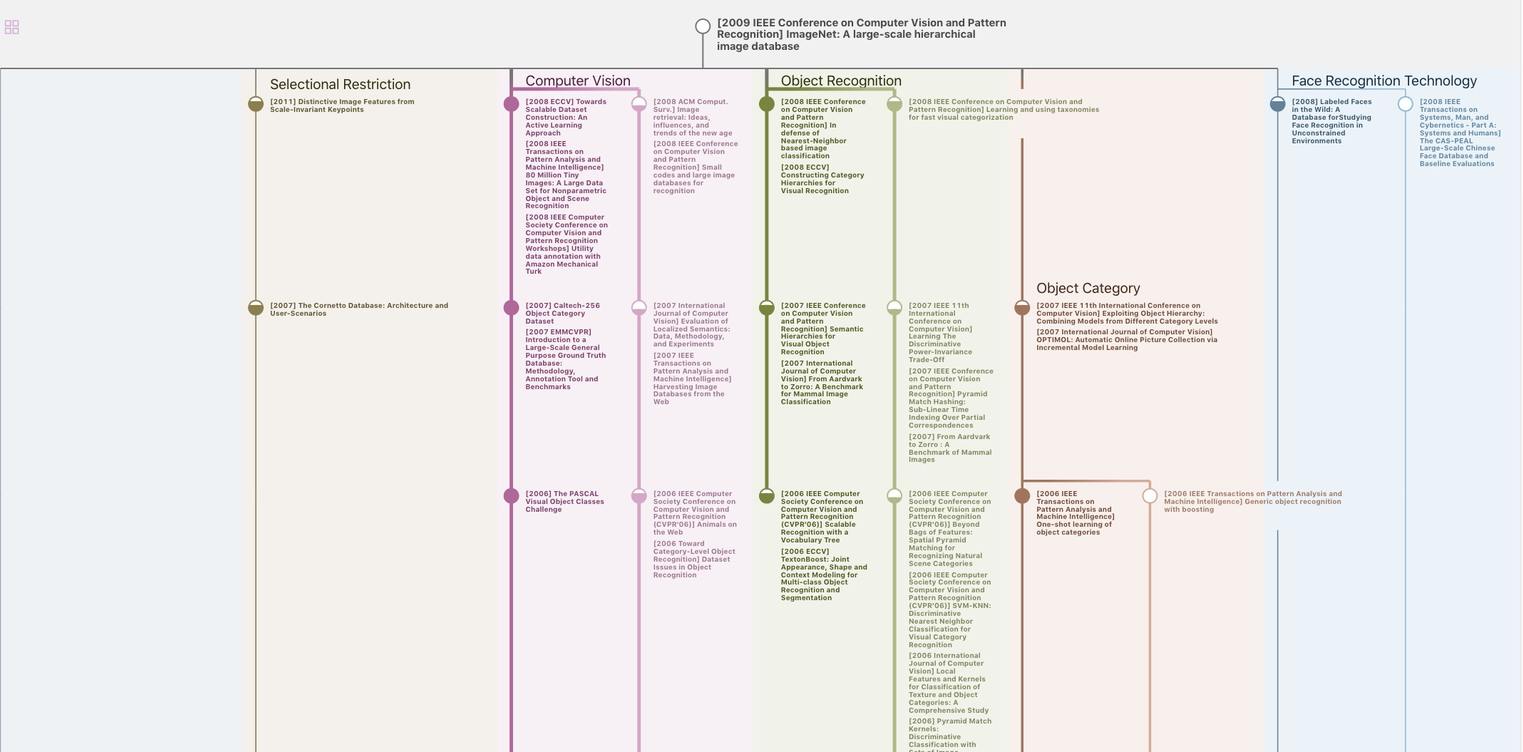
生成溯源树,研究论文发展脉络
Chat Paper
正在生成论文摘要