Self-supervised Re-identification for Online Joint Multi-object Tracking
Research Square (Research Square)(2023)
摘要
Abstract Recently, the bottleneck of multi-object tracking is shifting from detection performance to association performance. However, research on association algorithms requires a large number of identity labels, which are more expensive than detection labels. To circumvent the need for identity labels, we propose a Self-supervised Re-ID module for online joint Multi-Object Tracking (SR-MOT). Specifically, we design an appearance discriminator to judge identities with only the readily detection hypotheses and then associate the same identity to the final trajectory. To train the discriminator without using identity labels, we construct negative pairs by the detections appear in the same video frame as they definitely belong to different identities, the positive pairs are naturally constructed by several useful data augmentation methods. In addition, our proposed method balances the conflicting tasks of detection and re-ID by using different output features and we dynamically adjust the loss weights of detection and re-ID based on the information content of the loss distribution to promote balance between the two tasks from the feature level and optimization methods.In our evaluation on the MOT Challenge benchmark, we show that our SR-MOT performs comparably to supervised methods and is significantly superior to other unsupervised methods. Our proposed method provides a practical solution for multi-object tracking without the need for identity labels, making it more accessible for real-world applications.
更多查看译文
关键词
tracking,self-supervised,re-identification,multi-object
AI 理解论文
溯源树
样例
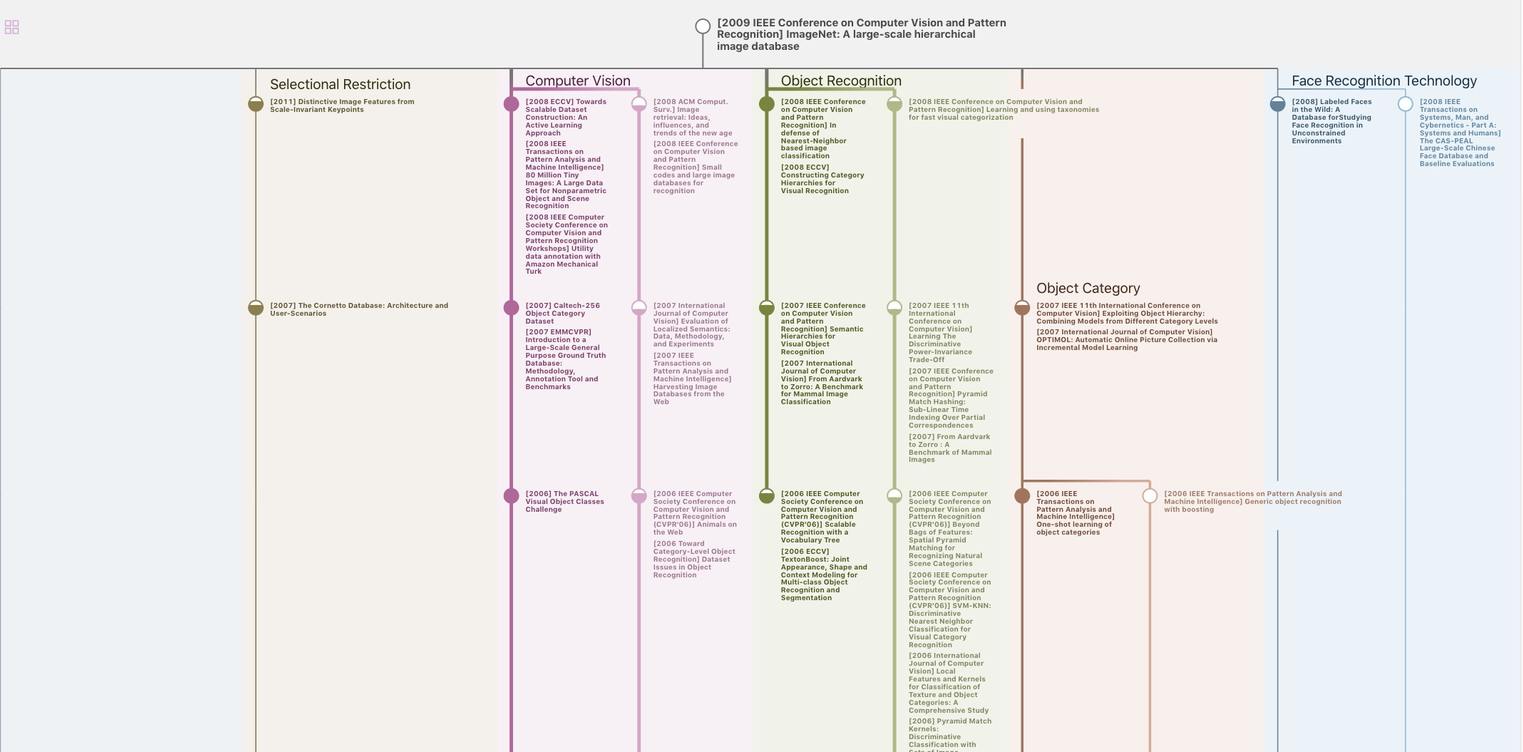
生成溯源树,研究论文发展脉络
Chat Paper
正在生成论文摘要