using the XGBoost classification algorithm and model interpretability techniques
Journal of Credit Risk(2023)
摘要
Sovereign debt has traditionally been regarded a safe asset class. However, the picture has shifted in the last decade, with a significant increase in the number of sovereign defaults. The drivers of this increase are complex and as yet unexplored, but it is clear that factors other than traditional macroeconomic events are significantly underpinning these economies. Therefore, understanding how the drivers of sovereign credit risk have evolved beyond economic and fiscal issues, as well as the importance of additional drivers in sovereign credit risk assessment, is critical. This paper explains the strength of the effect of spillover effects from private sector risks on sovereign debt risk, as well as the impact of rising sustainability risks on sovereign credit risk and their underlying relationship, using the XGBoost classification algorithm and model interpretability techniques, namely Shapley additive explanations (SHAP) and local interpretable model-agnostic explanations (LIME). First, we discover that private sector risks contribute significantly to sovereign credit risk and that these factors must be considered alongside macroeconomic variables when modeling sovereign credit risk. Further, we find evidence that sustainability risks are financially material to sovereign creditworthiness. The relationship between sustainability risks and sovereign credit risk is complex, follows a nonmonotonic relationship and is highly dependent on country specificities, necessitating the integration of sustainability risk factors while accounting for interactions with the economic state of a country.
更多查看译文
关键词
xgboost classification algorithm,model interpretability techniques
AI 理解论文
溯源树
样例
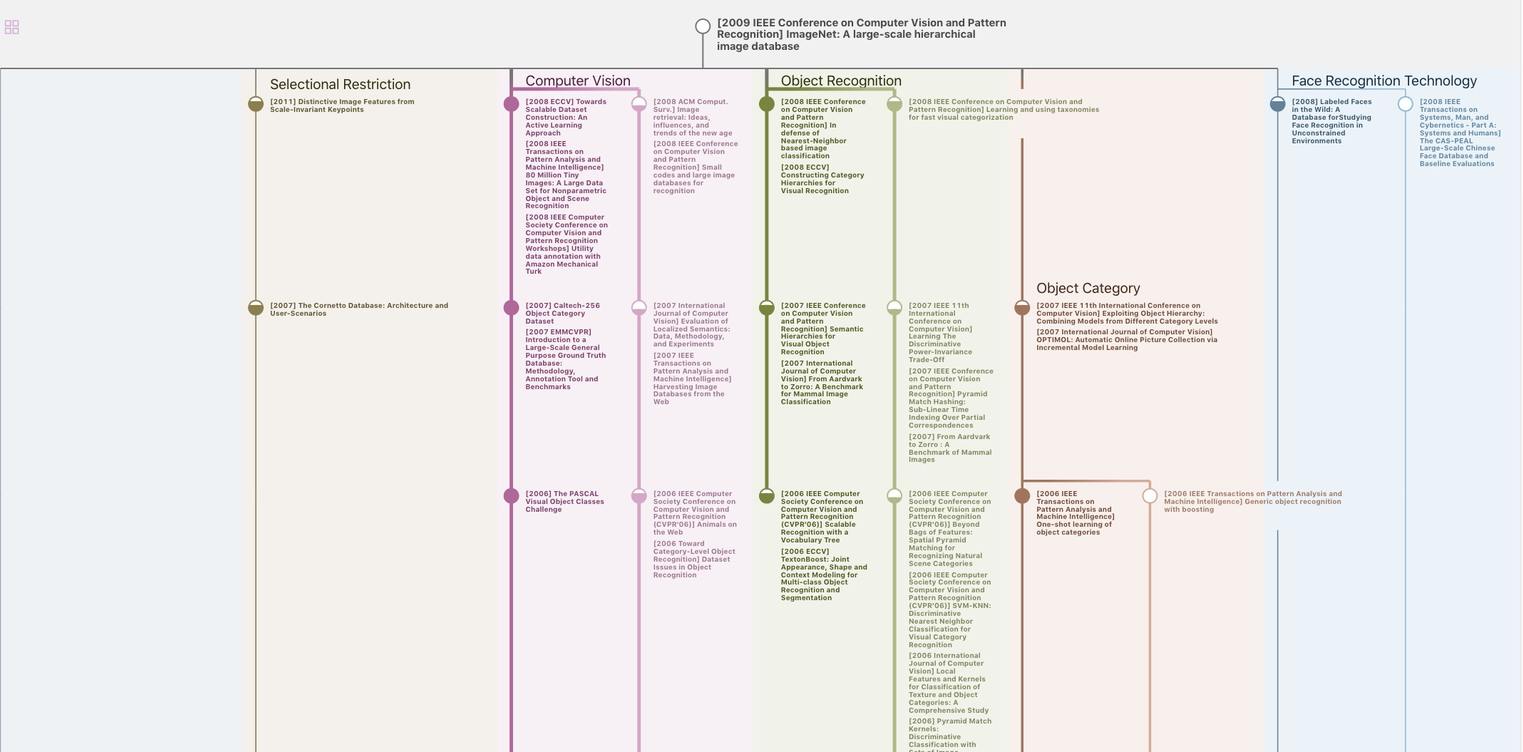
生成溯源树,研究论文发展脉络
Chat Paper
正在生成论文摘要