Implicit neural representations for unsupervised super-resolution and denoising of 4D flow MRI
arXiv (Cornell University)(2023)
摘要
4D flow MRI is a non-invasive imaging method that can measure blood flow velocities over time. However, the velocity fields detected by this technique have limitations due to low resolution and measurement noise. Coordinate-based neural networks have been researched to improve accuracy, with SIRENs being suitable for super-resolution tasks. Our study investigates SIRENs for time-varying 3-directional velocity fields measured in the aorta by 4D flow MRI, achieving denoising and super-resolution. We trained our method on voxel coordinates and benchmarked our approach using synthetic measurements and a real 4D flow MRI scan. Our optimized SIREN architecture outperformed state-of-the-art techniques, producing denoised and super-resolved velocity fields from clinical data. Our approach is quick to execute and straightforward to implement for novel cases, achieving 4D super-resolution.
更多查看译文
关键词
denoising,implicit neural representations,flow,super-resolution
AI 理解论文
溯源树
样例
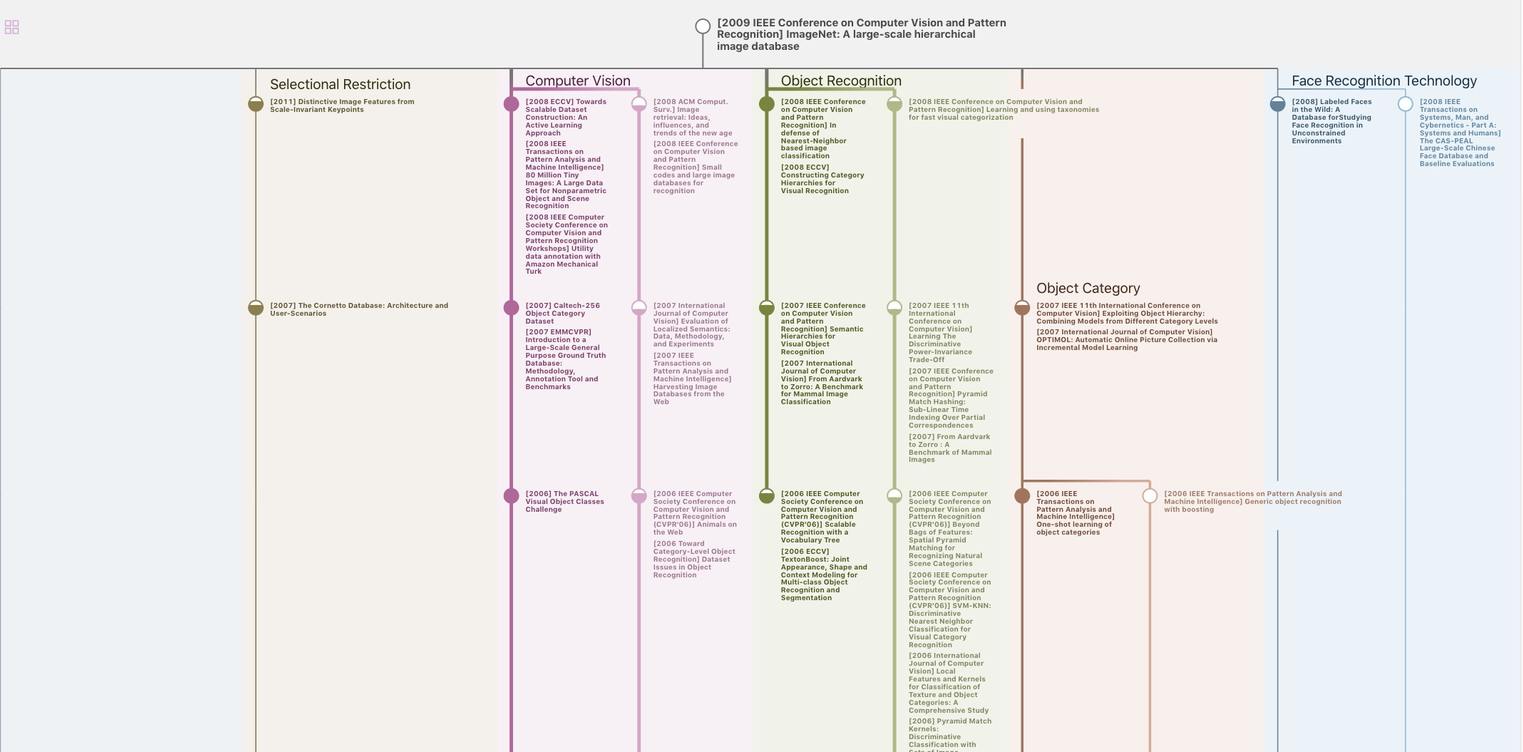
生成溯源树,研究论文发展脉络
Chat Paper
正在生成论文摘要