Less is More: Selective Layer Finetuning with SubTuning
arXiv (Cornell University)(2023)
摘要
Finetuning a pretrained model has become a standard approach for training neural networks on novel tasks, resulting in fast convergence and improved performance. In this work, we study an alternative finetuning method, where instead of finetuning all the weights of the network, we only train a carefully chosen subset of layers, keeping the rest of the weights frozen at their initial (pretrained) values. We demonstrate that \emph{subset finetuning} (or SubTuning) often achieves accuracy comparable to full finetuning of the model, and even surpasses the performance of full finetuning when training data is scarce. Therefore, SubTuning allows deploying new tasks at minimal computational cost, while enjoying the benefits of finetuning the entire model. This yields a simple and effective method for multi-task learning, where different tasks do not interfere with one another, and yet share most of the resources at inference time. We demonstrate the efficiency of SubTuning across multiple tasks, using different network architectures and pretraining methods.
更多查看译文
关键词
selective layer,subtuning
AI 理解论文
溯源树
样例
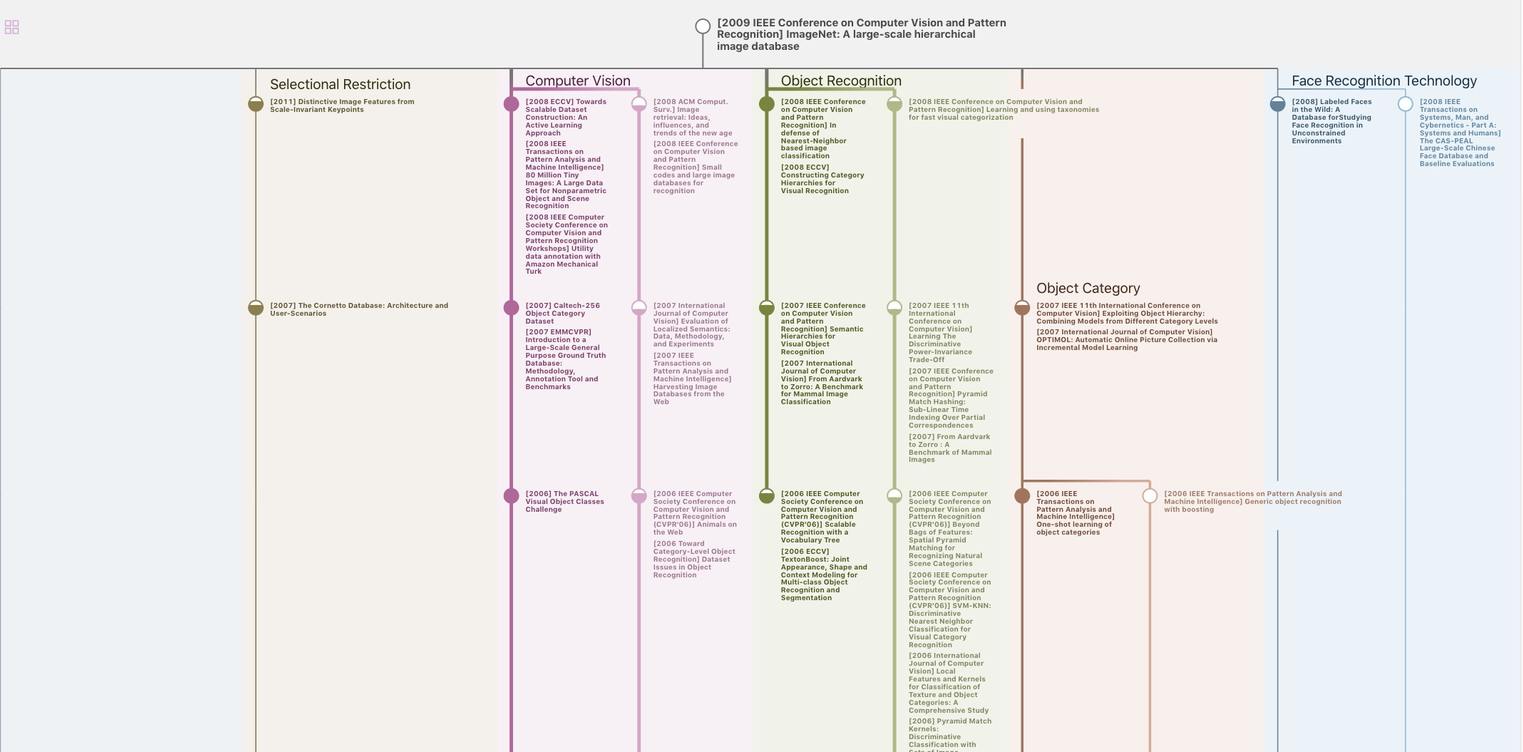
生成溯源树,研究论文发展脉络
Chat Paper
正在生成论文摘要