An artificial intelligence’s interpretation of complex high-resolution in situ transmission electron microscopy data
Matter(2023)
摘要
Complicated nano- and atomic-scale processes with sub-angstrom spatial resolution and millisecond time resolution visualized by in situ transmission electron microscopy (TEM) are often highly dynamical and time consuming to analyze and interpret. Here, we report how variational autoencoders (VAEs) can provide an artificial intelligence’s interpretation of high-resolution in situ TEM data by condensing and deconvoluting complicated atomic-scale dynamics into a latent space with reduced dimensionality. We designed a VAE model with high latent dimensions capable of deconvoluting information from complex high-resolution TEM data. We demonstrate how this model, with high latent dimensions trained on atomically resolved TEM images of lead sulfide (PbS) nanocrystals, is able to capture movements and perturbations of periodic lattices in both simulated and real in situ TEM data. The VAE model shows the capability of detecting and deconvoluting dynamical nanoscale physical processes, such as the rotation of crystal lattices and intra-particle ripening during the annealing of semiconductor nanocrystals.
更多查看译文
AI 理解论文
溯源树
样例
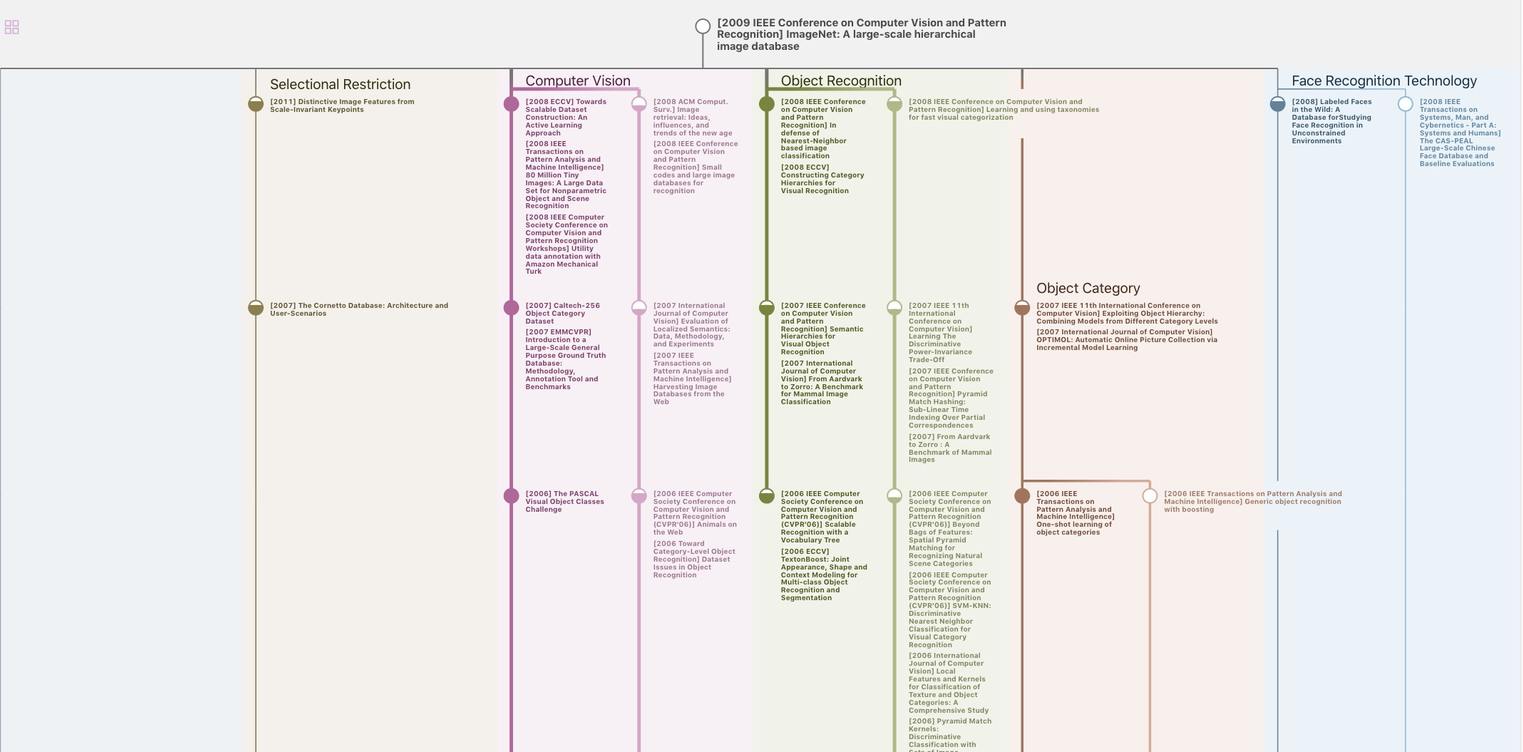
生成溯源树,研究论文发展脉络
Chat Paper
正在生成论文摘要