A Fault Detection Method Based on the Dynamic k-Nearest Neighbor Model and Dual Control Chart
IEEE TRANSACTIONS ON AUTOMATION SCIENCE AND ENGINEERING(2023)
摘要
The incipient fault detection of a complex industrial process is a challenging problem for traditional dynamic detection methods. Traditional dynamic detection methods usually decouple the correlations among the variables and dynamic correlations simultaneously, which makes the two types of correlations mixed and may lead to performance deteriorationin long-sequence dynamic detection. Some incipient faults maynot change the amplitudes of process variables but change thelong-sequence dynamic features. Based on theT(2)statistic andmatrix multiplication transformation ((TS)-S-2-MMT), traditionaldynamic detection methods can detect many faults effectively.However, theT(2)S-MMT can not effectively detect some incipientfaults due to the above two types of correlations mixed. In orderto overcome the shortcomings ofT(2)S-MMT and improve thedetection ability of some incipient faults, this paper proposes afault detection method based on the dynamic k-nearest neighbormodel and Dual Control Chart (DKNN-DCC), which can improvethe incipient fault detection performance by using long-sequencedynamic detection. The proposed method is verified by theTennessee Eastman (TE) process and the continuously stirredtank reactor (CSTR) process. The experimental results show theeffectiveness of the proposed method in incipient fault detectioncompared with traditional dynamic detection methods.
更多查看译文
关键词
Fault detection,Correlation,Kernel,Principal component analysis,Control charts,Fluctuations,Mathematical models,DCC,DKNN,DKNN-DCC,fault detection
AI 理解论文
溯源树
样例
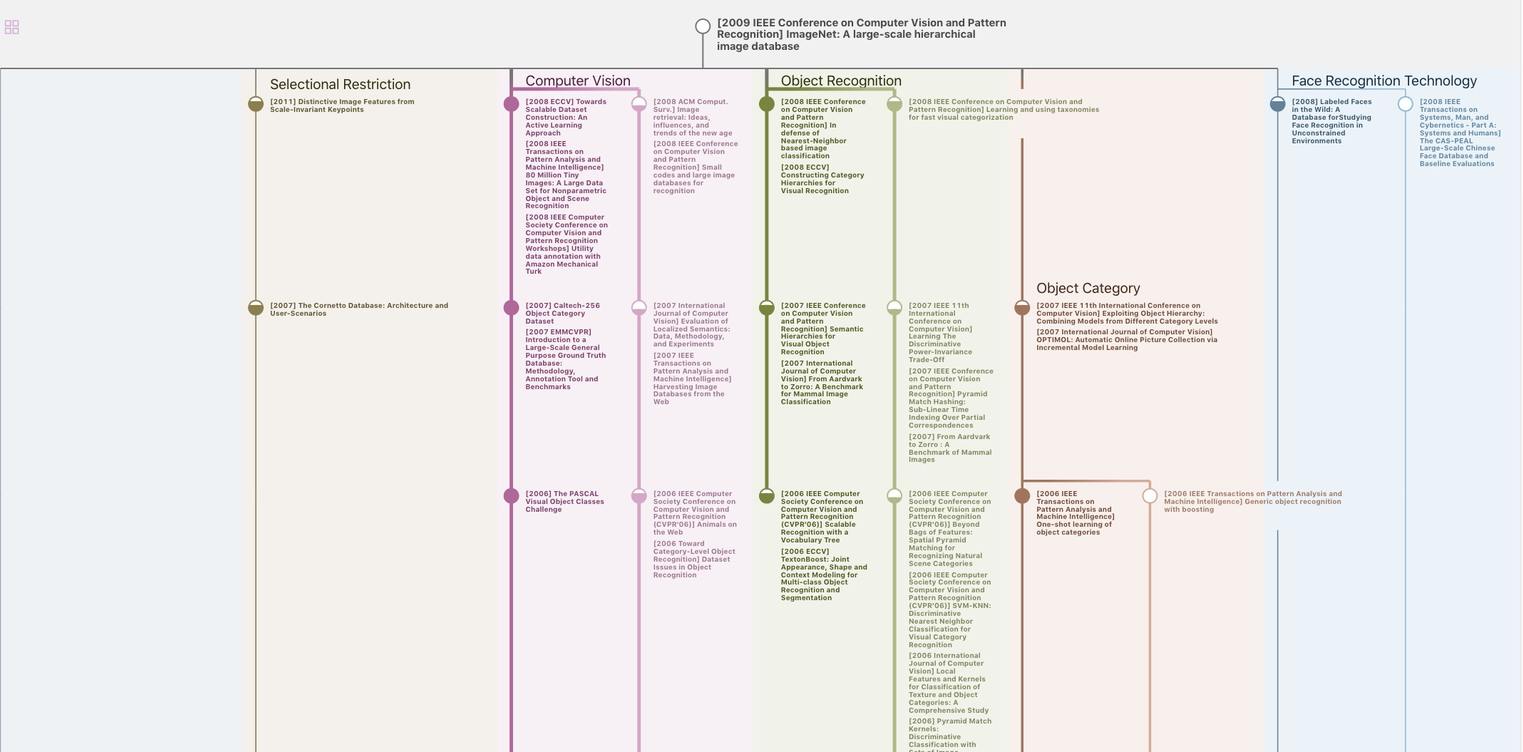
生成溯源树,研究论文发展脉络
Chat Paper
正在生成论文摘要