Deep Pixel-Wise Textures for Construction Waste Classification
IEEE TRANSACTIONS ON AUTOMATION SCIENCE AND ENGINEERING(2023)
摘要
Construction waste classification (CWC) is a crucial and challenging task. However, this promising CWC system has not yet been fully implemented worldwide. The similar appearance of construction waste makes it difficult to be classified. We believe that if the characteristics of the appearance between different materials can not be fully extracted and effectively propagated, any further improvements of CWC models will be futile. To this end, this paper develops a texture and pixel gradient convolutional network (TPCNet) to capture material features. We find that the texture features as the additional input features can provide intrinsic fine-grained patterns. First, we propose the early-stage fusion strategy, where natural texture features and RGB are concatenated together as input. Second, we propose a texture and pixel gradient convolution (TPC) module to consider subtle texture information. TPC focuses on the texture guidance and introduces it to the modern CNNs, which enjoy the best of both worlds. We extend TPC to GTPC using a lossless synthetic large kernel (SLK) to achieve global contextual dependencies and seamlessly incorporate channel-wise adaptability. Moreover, we propose a two-stage feature pyramid network (TFPN) to fuse richer and denser spatial multi-scale contextual information. We also construct a large-scale real-world dataset and a production line to demonstrate the effectiveness of our model.Note to Practitioners-This paper is motivated by the problem in real-world construction waste classification task. The construction waste have similar 2D appearance makes them hard to be classified. Current models often blindly feed raw RGB images into CNNs instead of considering specific features, such as textured material surface textures. This paper proposes the texture guidance mechanism and introduces it to the training of CNN. Furthermore, we propose a lossless synthetic large kernel (SLK) to achieve global texture information. By introducing texture information as a basis for classification, our model can effectively classify similarly shaped construction waste and improve segmentation performance, thus providing the robot with more accurate location information.
更多查看译文
关键词
Construction waste classification,texture and pixel gradient convolution,texture-aware,feature enhancement,synthetic large kernel
AI 理解论文
溯源树
样例
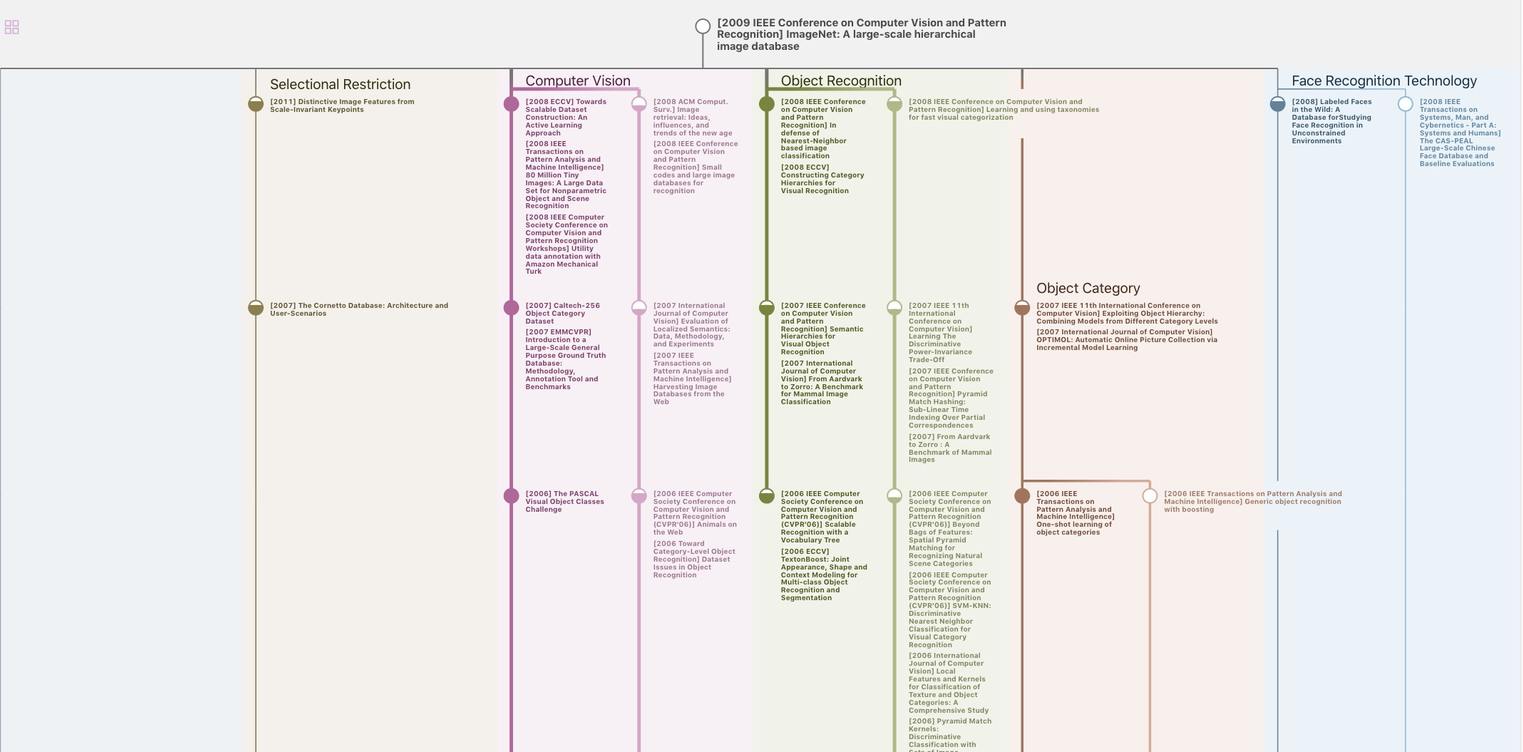
生成溯源树,研究论文发展脉络
Chat Paper
正在生成论文摘要