A Block-Structured Optimization Approach for Data Sensing and Computing in Vehicle-Assisted Edge Computing Networks
IEEE SENSORS JOURNAL(2024)
摘要
With the rapid development of Internet-of-things (IoT) applications and multiaccess edge computing (MEC) technology, massive amounts of sensing data can be collected and transmitted to MEC servers for rapid processing. On the other hand, as the number of IoT devices grows, the MEC server cannot perform tremendous computing tasks because of its limited computation capacity. This article introduces a vehicle-assisted MEC framework that leverages vehicles to provide computational services for IoT devices and overcome this challenge. The problem of latency minimization was formulated by optimizing the sensing data rate, offloading decisions, and resource allocation while considering energy consumption constraints. Nevertheless, achieving the global optimal solution in polynomial time is challenging because the formulated problem is mixed-integer nonlinear and nonconvex. This article provides an efficient algorithm that adopts the block coordinate descent technique to decompose the original problem into four subproblems. These subproblems can be solved using Lagrangian relaxation and the block successive upper bound minimization (BSUM) method. The superiority of the proposed approach in reducing latency compared with baseline schemes is evident from the simulation results.
更多查看译文
关键词
Computation offloading,data sensing,edge computing,resource allocation,vehicular network
AI 理解论文
溯源树
样例
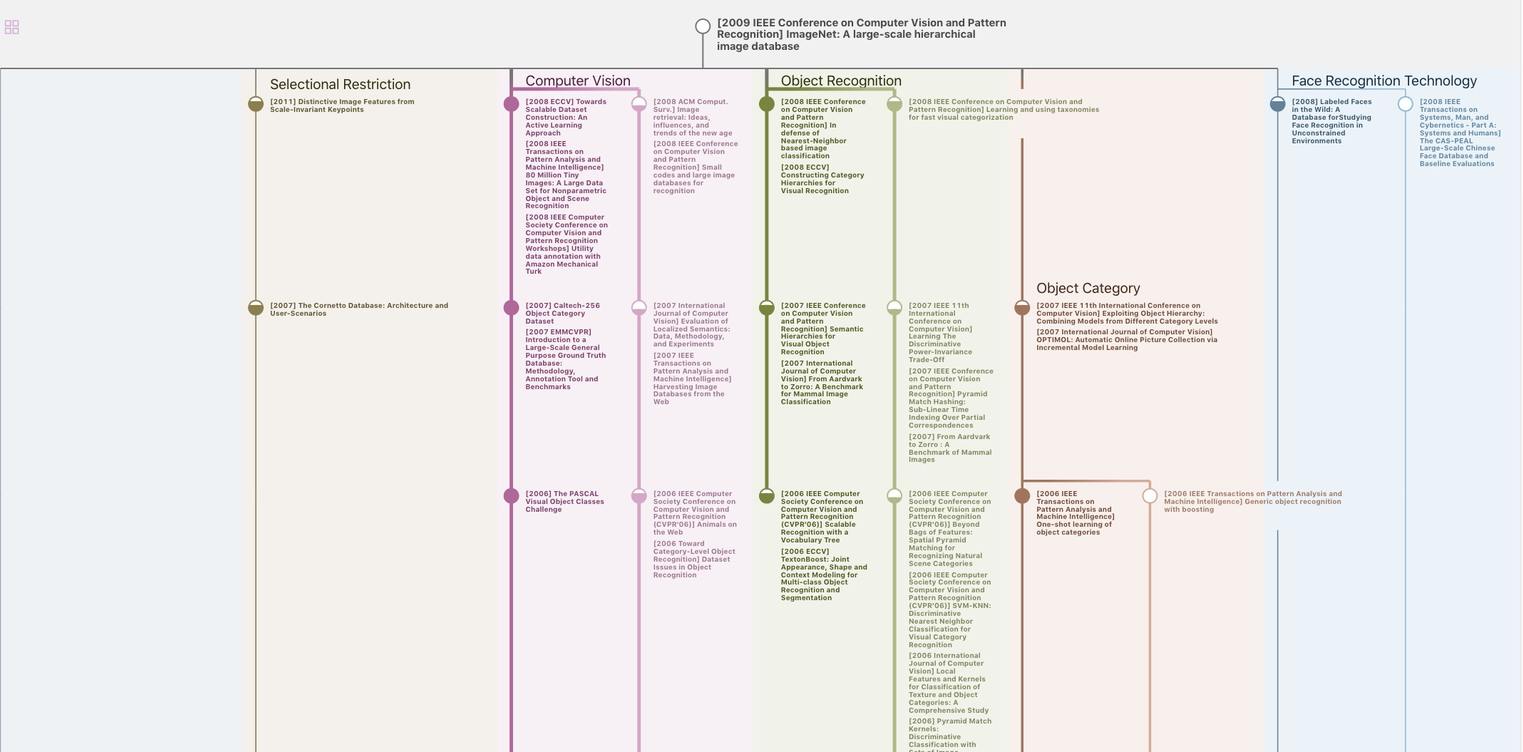
生成溯源树,研究论文发展脉络
Chat Paper
正在生成论文摘要