DSA-Net: An Attention-Guided Network for Real-Time Defect Detection of Transmission Line Dampers Applied to UAV Inspections
IEEE TRANSACTIONS ON INSTRUMENTATION AND MEASUREMENT(2024)
摘要
As a crucial component in power transmission lines, structural defects in dampers can significantly reduce their ability to suppress wire vibrations, posing a serious threat to the transmission line infrastructure. Most existing methods for damper defect detection are based on deep learning. However, these methods face challenges when applied to unmanned aerial vehicle (UAV)-captured images of dampers, as they often appear in complex backgrounds and contain small and densely distributed objects. This research aims to improve the accuracy and speed of damper defect recognition by proposing an attention-guided damper defect detection network called DSA-Net, which includes components such as damper attention (DA), Stile path aggregation network (Stile PAN), and ASFFs. First, to accurately extract the features of the damper while reducing background interference, a novel attention mechanism called DA is introduced, based on the shape of the damper and bidirectional stripe convolution. This mechanism allows the network to focus on key regions in the image without incurring expensive computational costs. Second, the Stile PAN feature fusion structure is employed to integrate shallow-level information of small targets. Finally, a single-layer ASFFs structure is utilized to autonomously learn information about small and densely distributed targets from the detection feature maps output by Stile PAN. On the transmission line damper (TLD) dataset, DSA-Net achieves an mAP0.5 of 0.935, an mAP0.5: 0.95 of 0.789, and an inference speed of 7.2 ms.
更多查看译文
关键词
Attention mechanism,damper,transmission line,unmanned aerial vehicle (UAV),visible light image
AI 理解论文
溯源树
样例
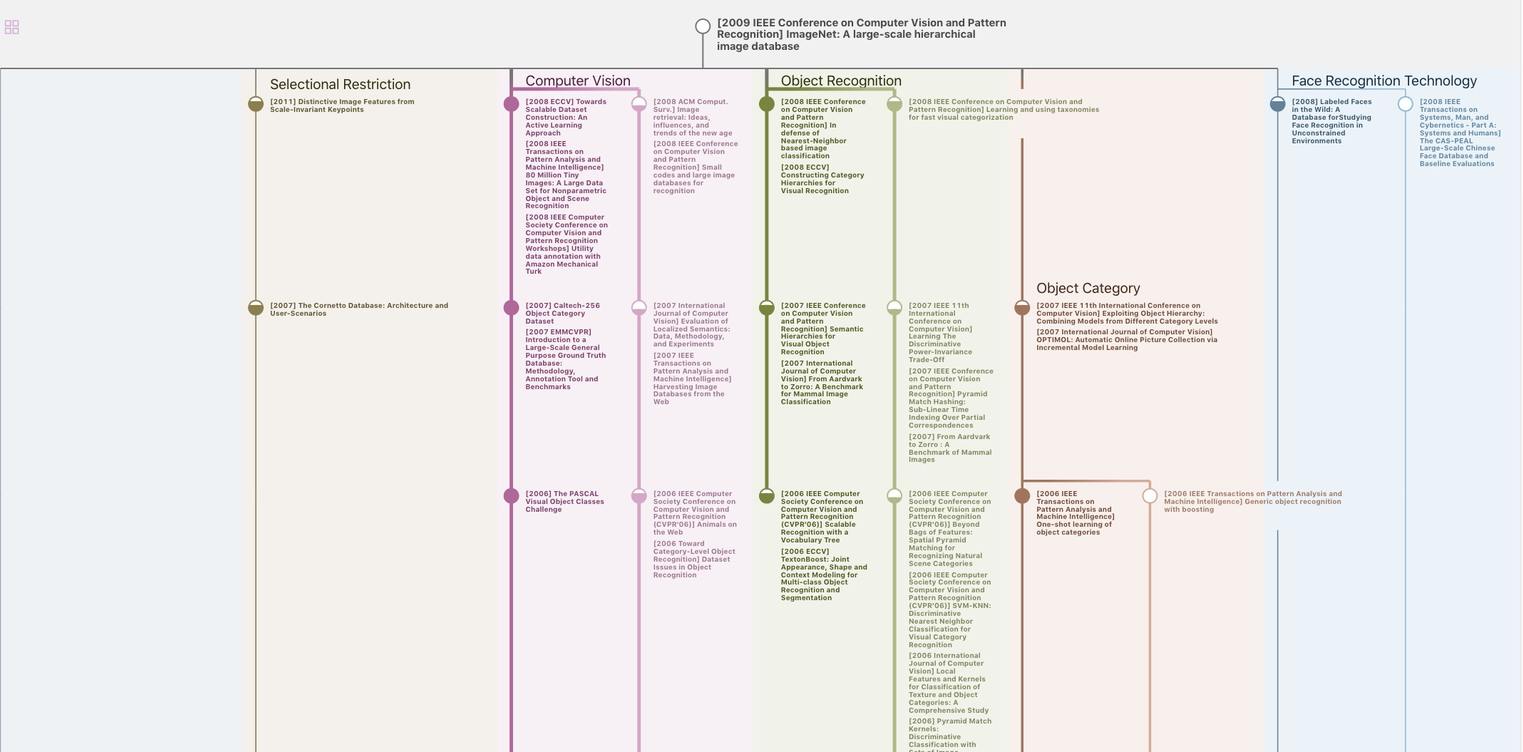
生成溯源树,研究论文发展脉络
Chat Paper
正在生成论文摘要