Hi-RCA: A Hierarchy Anomaly Diagnosis Framework Based on Causality and Correlation Analysis
APPLIED SCIENCES-BASEL(2023)
摘要
Microservice architecture has been widely adopted by large-scale applications. Due to the huge amount of data and complex microservice dependency, it also poses new challenges in ensuring reliable performance and maintenance. Existing approaches still suffer from limitations of anomaly data, over-simplification of metric relationships, and lack of diagnosing interpretability. To solve these issues, this paper builds a hierarchy root cause diagnosis framework, named Hi-RCA. We propose a global perspective to characterize different abnormal symptoms, which focuses on changes in metrics' causation and correlation. We decompose the diagnosis task into two phases: anomalous microservice location and anomalous reason diagnosis. In the first phase, we use Kalman filtering to quantify microservice abnormality based on the estimation error. In the second phase, we use causation analysis to identify anomalous metrics and generate anomaly knowledge graphs; by correlation analysis, we construct an anomaly propagation graph and explain the anomaly symptoms via graph comparison. Our experimental evaluation on an open dataset shows that Hi-RCA can effectively locate root causes with 90% mean average precision, outperforming state-of-the-art methods.
更多查看译文
关键词
microservice architecture,intervention recognition,root cause location
AI 理解论文
溯源树
样例
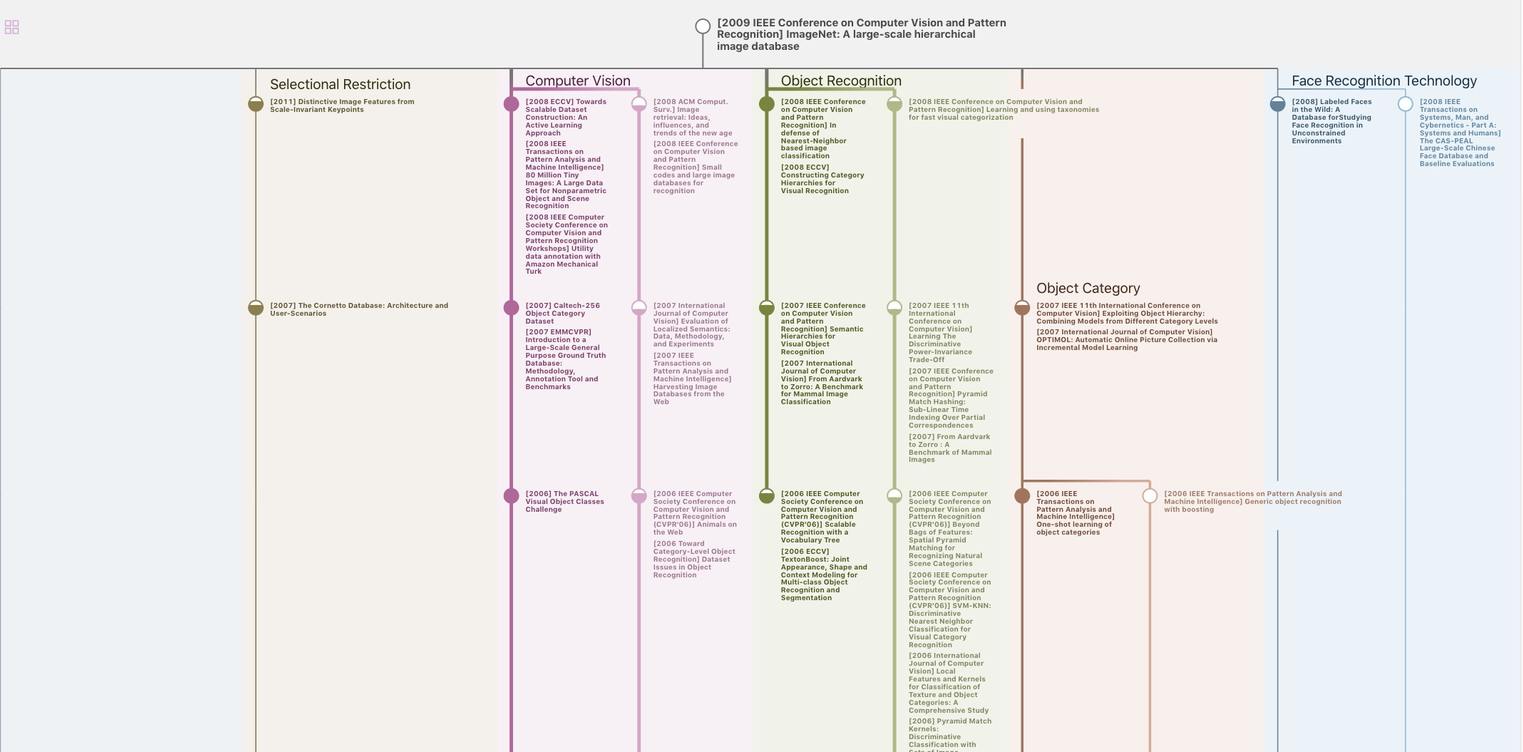
生成溯源树,研究论文发展脉络
Chat Paper
正在生成论文摘要