An Efficient Incentive Mechanism for Federated Learning in Vehicular Networks
IEEE Network(2023)
摘要
As a novel machine learning technique, the promising performance of Federated Learning (FL) paradigms has been well recognized. However, the incentive issue for this framework is still under-explored. Many existing incentive mechanisms are computationally complex and result in increased communication overhead, making them unsuitable for Vehicular Networks. To address this issue, we propose a simple yet efficient incentive mechanism that identifies agents with high-quality resources and motivates them to behave actively in the learning process, such that the final aggregated model is accurate, with a reduced network overhead. Especially, the specific requirements of end-users and the uniqueness of raw data are considered. Our evaluations on both synthetic and real-world datasets demonstrate that the clustering accuracy can be slightly improved (by around 2%), while the network overhead can be decreased by up to 70%, and the convergence time can be reduced by up to 9%.
更多查看译文
关键词
Federated Learning,Distributed algorithm,Clustering,Incentive Mechanism
AI 理解论文
溯源树
样例
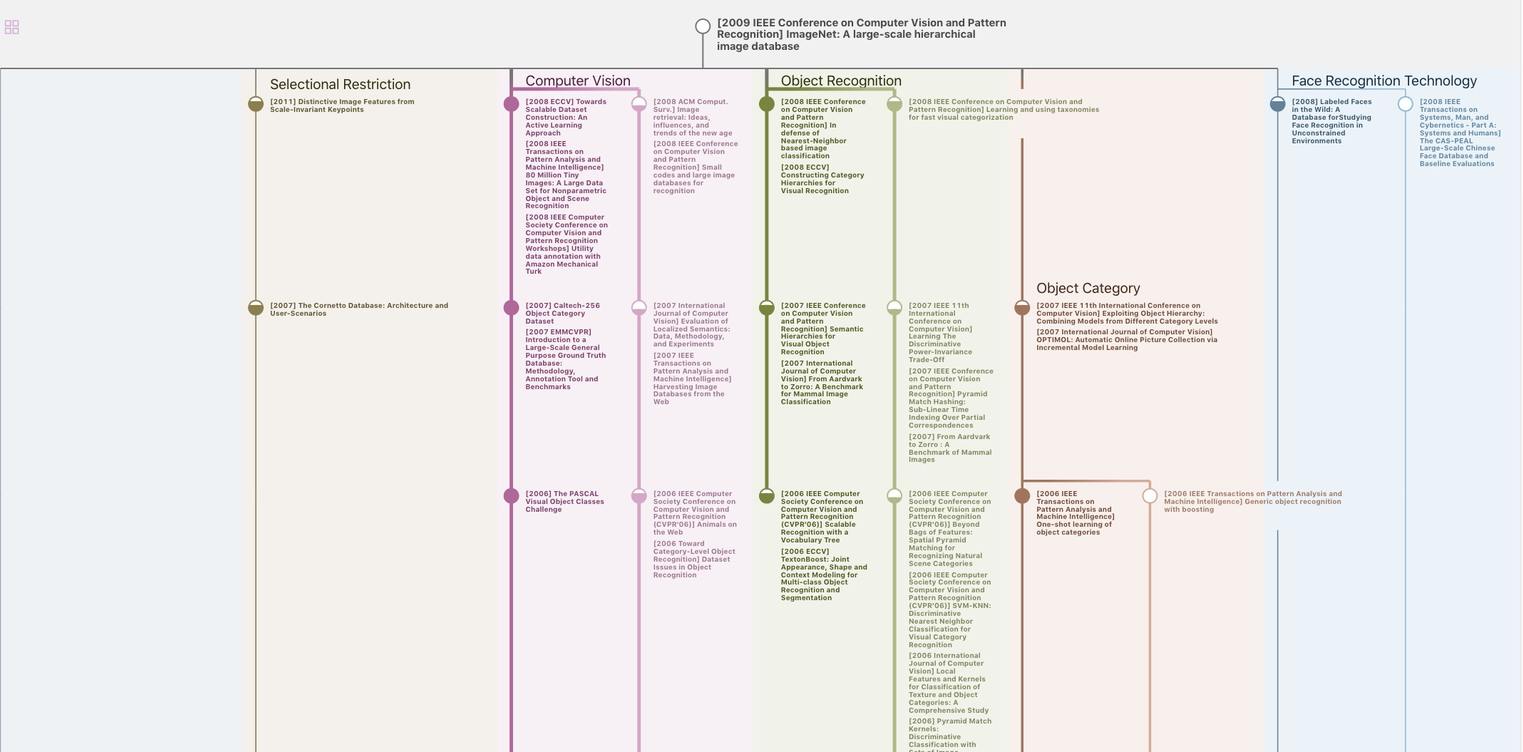
生成溯源树,研究论文发展脉络
Chat Paper
正在生成论文摘要