An adaptive approach for online monitoring of large-scale data streams
IISE TRANSACTIONS(2023)
摘要
In this article, we propose an adaptive top-r method to monitor large-scale data streams where the change may affect a set of unknown data streams at some unknown time. Motivated by parallel and distributed computing, we propose to develop global monitoring schemes by parallel running local detection procedures and then use the Benjamin-Hochberg false discovery rate control procedure to estimate the number of changed data streams adaptively. Our approach is illustrated in two concrete examples: one is a homogeneous case when all data streams are independent and identically distributed with the same known pre-change and post-change distributions. The other is when all data are normally distributed, and the mean shifts are unknown and can be positive or negative. Theoretically, we show that when the pre-change and post-change distributions are completely specified, our proposed method can estimate the number of changed data streams for both the pre-change and post-change status. Moreover, we perform simulations and two case studies to show its detection efficiency.
更多查看译文
关键词
False discovery rate,CUSUM,quickest change detection,process control
AI 理解论文
溯源树
样例
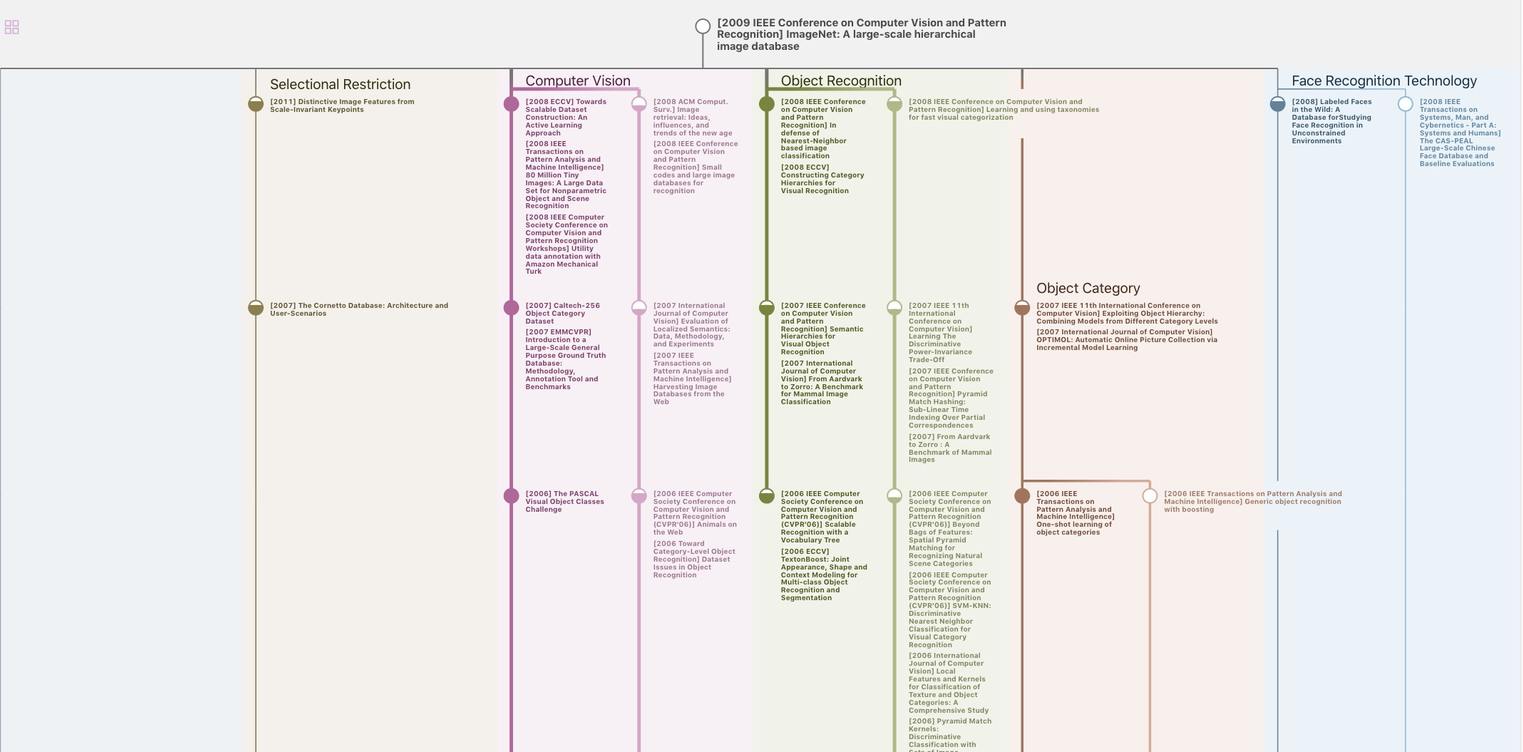
生成溯源树,研究论文发展脉络
Chat Paper
正在生成论文摘要