A Novel and Efficient Point Cloud Registration by using Coarse-to-Fine Strategy Integrating PointNet
INTERNATIONAL JOURNAL OF ADVANCED COMPUTER SCIENCE AND APPLICATIONS(2023)
摘要
The registration of the point cloud plays a critical and fundamental role in the computer vision domain. Although quite good registration results have been obtained by using the global, local, and learning-based registration strategies, there are still many problems to solve. For example, the local methods that are based on geometric features are very sensitive to attitude deviation, the global shapes-based methods are easy to result in inconsistency when the distribution differences are obvious and the learning-based registration methods have highly relied on the huge label data. A novel and effective registration method for the point cloud data integrating the coarse-to-fine strategy and the improved PointNet network is proposed to overcome the abovementioned drawbacks and improve registration accuracy. The improved Random Sample Consensus (RANSAC) algorithm is developed to effectively deal with the initial attitude deviation problem in the coarse registration procedure and the improved Lucas and Kanade (LK) algorithm is proposed based on the classical PointNet framework to reduce the errors of the refine registration, and the whole registration procedure is implemented under a trainable recurrent deep learning architecture. Compared with the state-of-the-art point cloud registration methods, experimental results fully prove that the proposed method can effectively handle the significant attitude deviation and partial overlap problem and achieves stronger robustness and higher accuracy.
更多查看译文
关键词
Point cloud registration,PointNet,coarse-fine registration,random sample consensus (RANSAC) algorithm,Lucas and Kanade (LK) algorithm
AI 理解论文
溯源树
样例
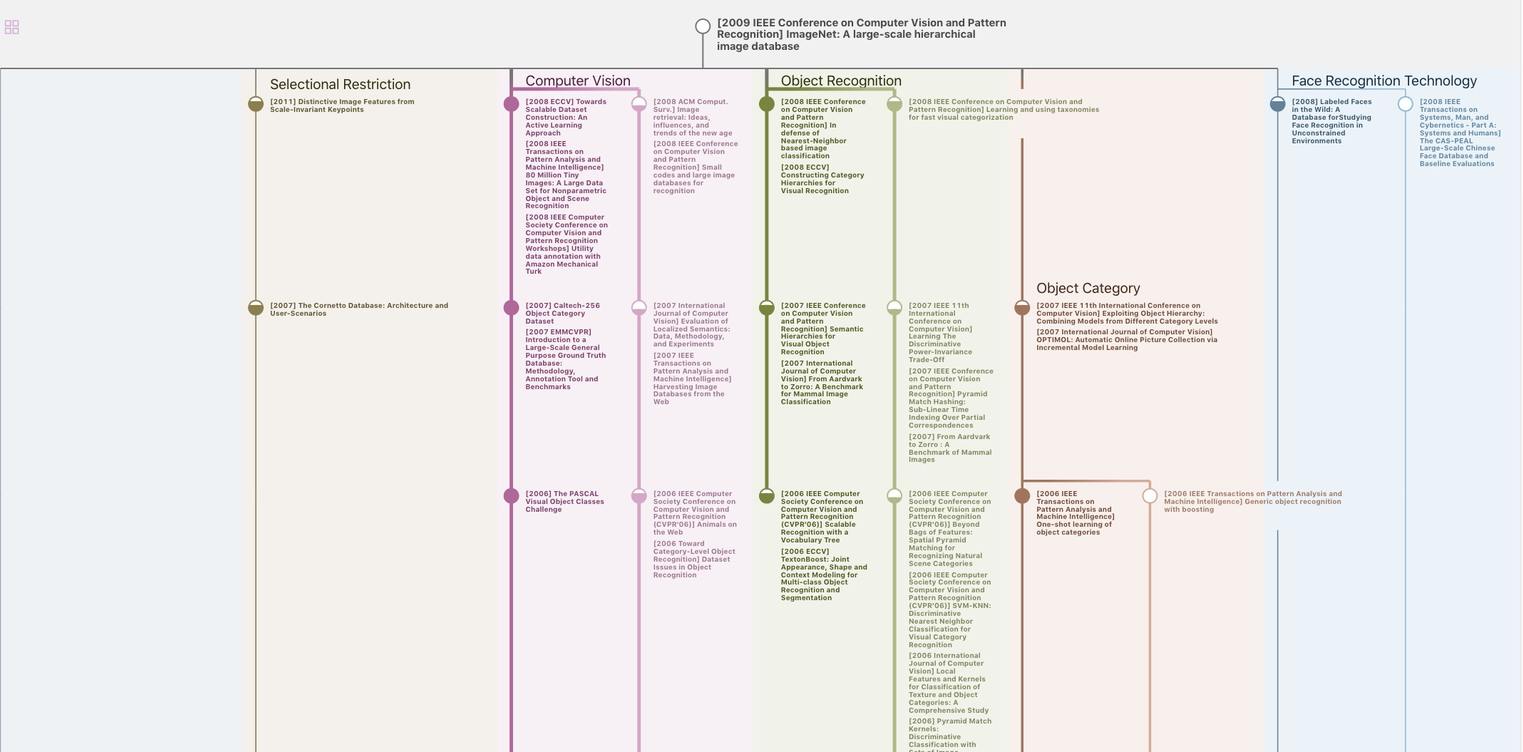
生成溯源树,研究论文发展脉络
Chat Paper
正在生成论文摘要