A Neural Database for Answering Aggregate Queries on Incomplete Relational Data
IEEE Transactions on Knowledge and Data Engineering(2023)
摘要
Real-world datasets are often incomplete due to data collection cost, privacy considerations or as a side effect of data integration/preparation. We focus on answering aggregate queries on such datasets, where data incompleteness causes the answers to be inaccurate. To address this problem, assuming typical relational data, existing work generates synthetic data to complete the database, a challenging task, especially in the presence of bias in observed data. Instead, we propose a paradigm shift by learning to directly estimate query answers, circumventing the difficult data generation step. Our approach, dubbed NeuroComplete, learns to answer queries in three steps. First, NeuroComplete generates a set of queries for which accurate answers can be computed given the incomplete dataset. Next, it embeds queries in a feature space, through which each query is effectively represented with the portion of the database that contributes to the query answer. Finally, it trains a neural network in a supervised learning fashion: both query features (input) and correct answers (labels) are known. The learned model generates accurate answers to new queries at test time, exploiting the generalizability of the learned model in the embedding space. Extensive experimental results on real datasets show up to 4 times for AVG queries and 10 times for COUNT queries error reduction compared with the state-of-the-art.
更多查看译文
关键词
Relational Database,Missing Data,Analytical Queries,Machine Learning
AI 理解论文
溯源树
样例
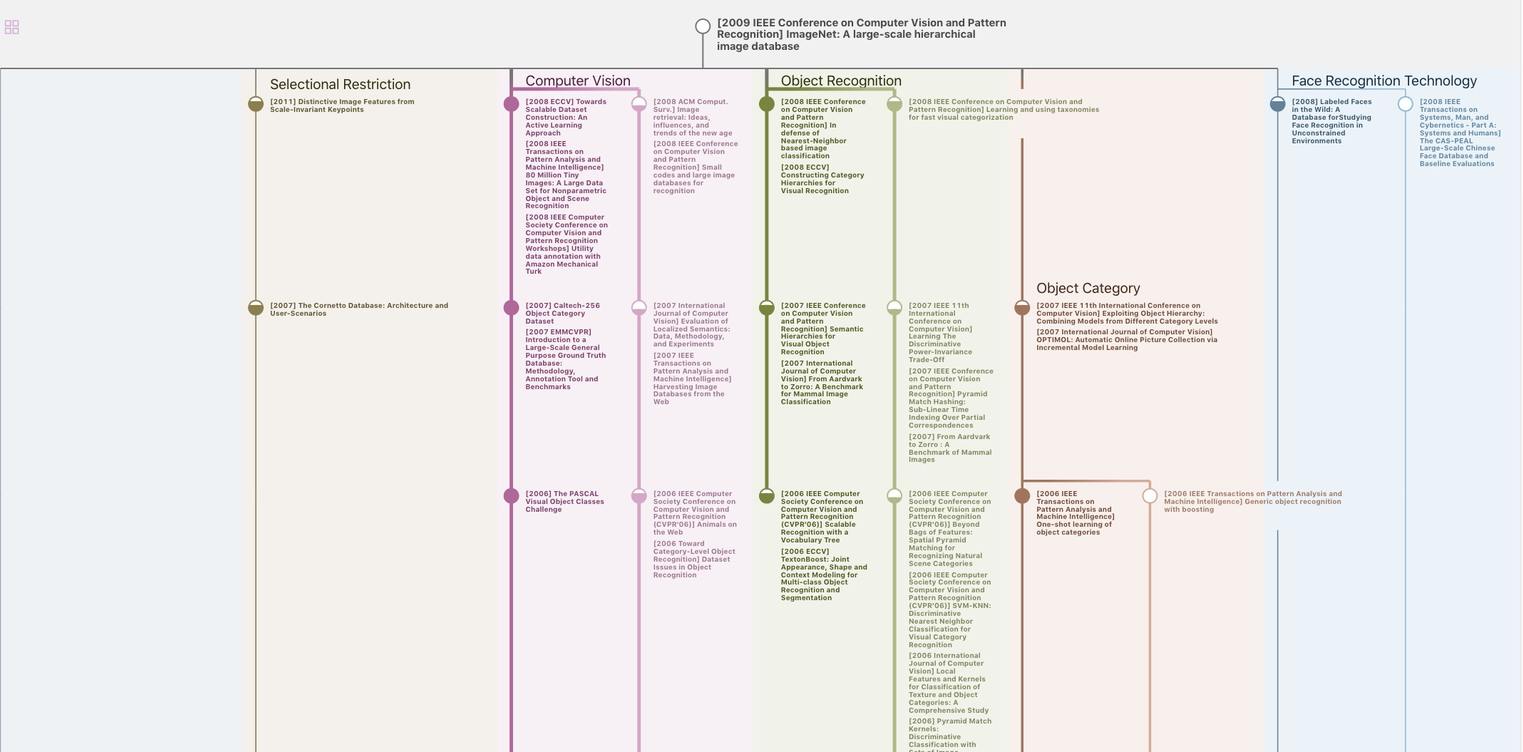
生成溯源树,研究论文发展脉络
Chat Paper
正在生成论文摘要