SSPNet: Predicting Visual Saliency Shifts.
IEEE Trans. Multim.(2024)
摘要
When images undergo quality degradation caused by editing, compression or transmission, their saliency tends to shift away from its original position. Saliency shifts indicate visual behaviour change and therefore contain vital information regarding perception of visual content and its distortions. Given a pristine image and its distorted format, we want to be able to detect saliency shifts induced by distortions. The resulting saliency shift map (SSM) can be used to identify the region and degree of visual distraction caused by distortions, and consequently to perceptually optimise image coding or enhancement algorithms. To this end, we first create a largest-of-its-kind eye-tracking database, comprising 60 pristine images and their associated 540 distorted formats viewed by 96 subjects. We then propose a computational model to predict the saliency shift map (SSM), utilising transformers and convolutional neural networks. Experimental results demonstrate that the proposed model is highly effective in detecting distortion-induced saliency shifts in natural images.
更多查看译文
关键词
Saliency,saliency shift,eye-tracking,transformer,convolutional neural networks
AI 理解论文
溯源树
样例
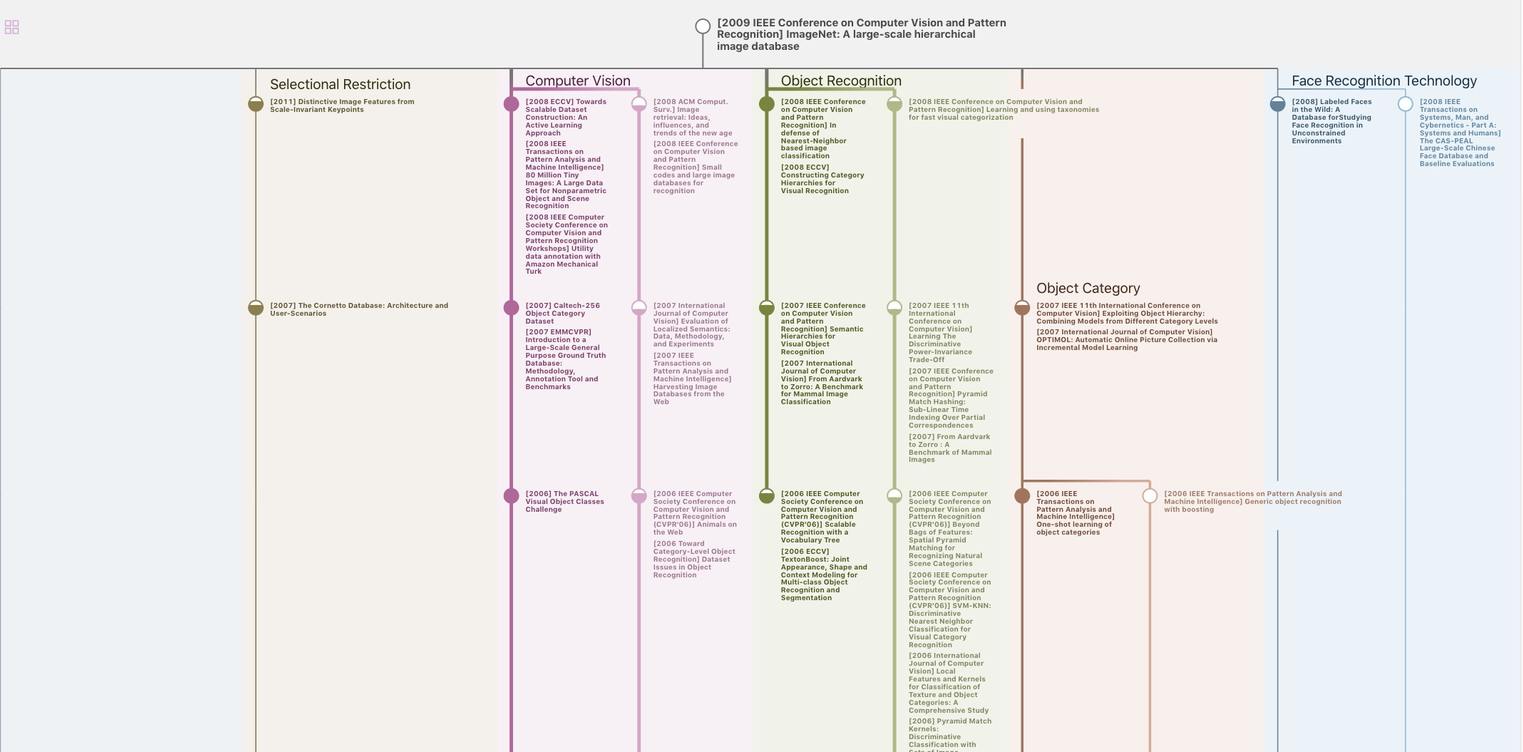
生成溯源树,研究论文发展脉络
Chat Paper
正在生成论文摘要