Dynamic Incentive Pricing on Charging Stations for Real-Time Congestion Management in Distribution Network: An Adaptive Model-Based Safe Deep Reinforcement Learning Method
IEEE TRANSACTIONS ON SUSTAINABLE ENERGY(2024)
摘要
This paper concerns the pricing strategy for real-time distribution network congestion management, aiming at maximizing the total social welfare while eliminating congestion. The difficulty lies in dealing with the complex time-varying relationship between price and charging demand in the integrated power system and transportation network, and ensuring the safe application of the proposed method with limited real historical data. To address these challenges, we first model the EV user charging decision-making process to indirectly reflect the unquantifiable price and demand relationship, and then formulate the pricing problem as a bi-level model with a constrained Markov Decision Process (CMDP). After that, we propose a model-based safe DRL framework and develop an adaptive model-based safe deep reinforcement learning (AMSDRL) algorithm to solve the CMDP problem. AMSDRL learns the environment transition model and uses a strict and adaptive cost constraint to offset potential modeling errors. Compared to the state-of-the-art safe DRL methods, AMSDRL can be deployed with security guarantees by training on limited historical data, which is more practical for applications. The numerical results on modified IEEE 33-bus and 118-bus systems and a transportation network with real-world EV data demonstrate the effectiveness of the proposed method.
更多查看译文
关键词
Congestion management,constrained Markov decision process,EV charging pricing,power and transportation system,model-based safe deep reinforcement learning
AI 理解论文
溯源树
样例
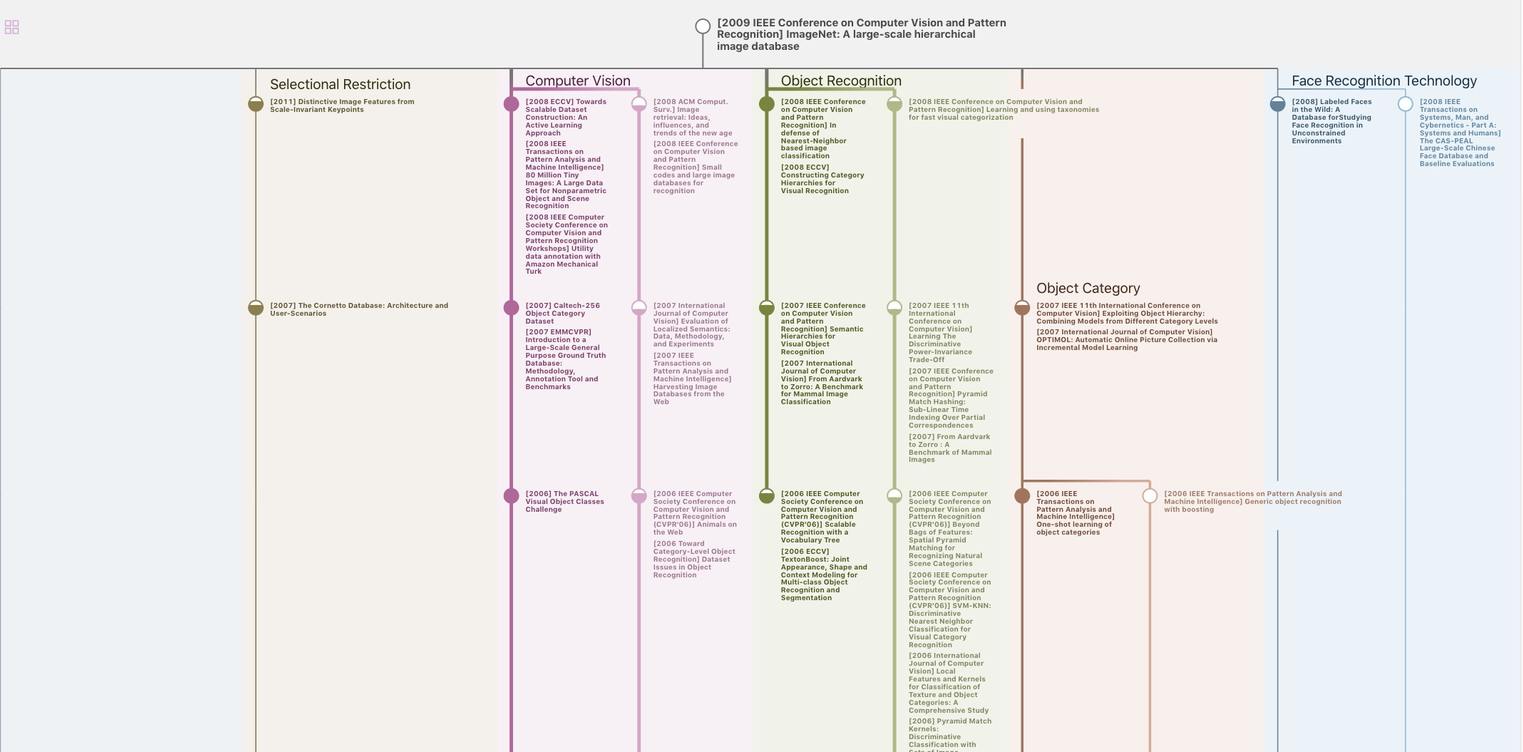
生成溯源树,研究论文发展脉络
Chat Paper
正在生成论文摘要