Testing Deep Learning Recommender Systems Models on Synthetic GAN-Generated Datasets
INTERNATIONAL JOURNAL OF INTERACTIVE MULTIMEDIA AND ARTIFICIAL INTELLIGENCE(2023)
摘要
The published method Generative Adversarial Networks for Recommender Systems (GANRS) allows generating data sets for collaborative filtering recommendation systems. The GANRS source code is available along with a representative set of generated datasets. We have tested the GANRS method by creating multiple synthetic datasets from three different real datasets taken as a source. Experiments include variations in the number of users in the synthetic datasets, as well as a different number of samples. We have also selected six state-of-the-art collaborative filtering deep learning models to test both their comparative performance and the GANRS method. The results show a consistent behavior of the generated datasets compared to the source ones; particularly, in the obtained values and trends of the precision and recall quality measures. The tested deep learning models have also performed as expected on all synthetic datasets, making it possible to compare the results with those obtained from the real source data. Future work is proposed, including different cold start scenarios, unbalanced data, and demographic fairness.
更多查看译文
关键词
Collaborative Filtering,Deep Learning,GANRS,Generated Datasets,Recommender Systems,Synthetic Datasets
AI 理解论文
溯源树
样例
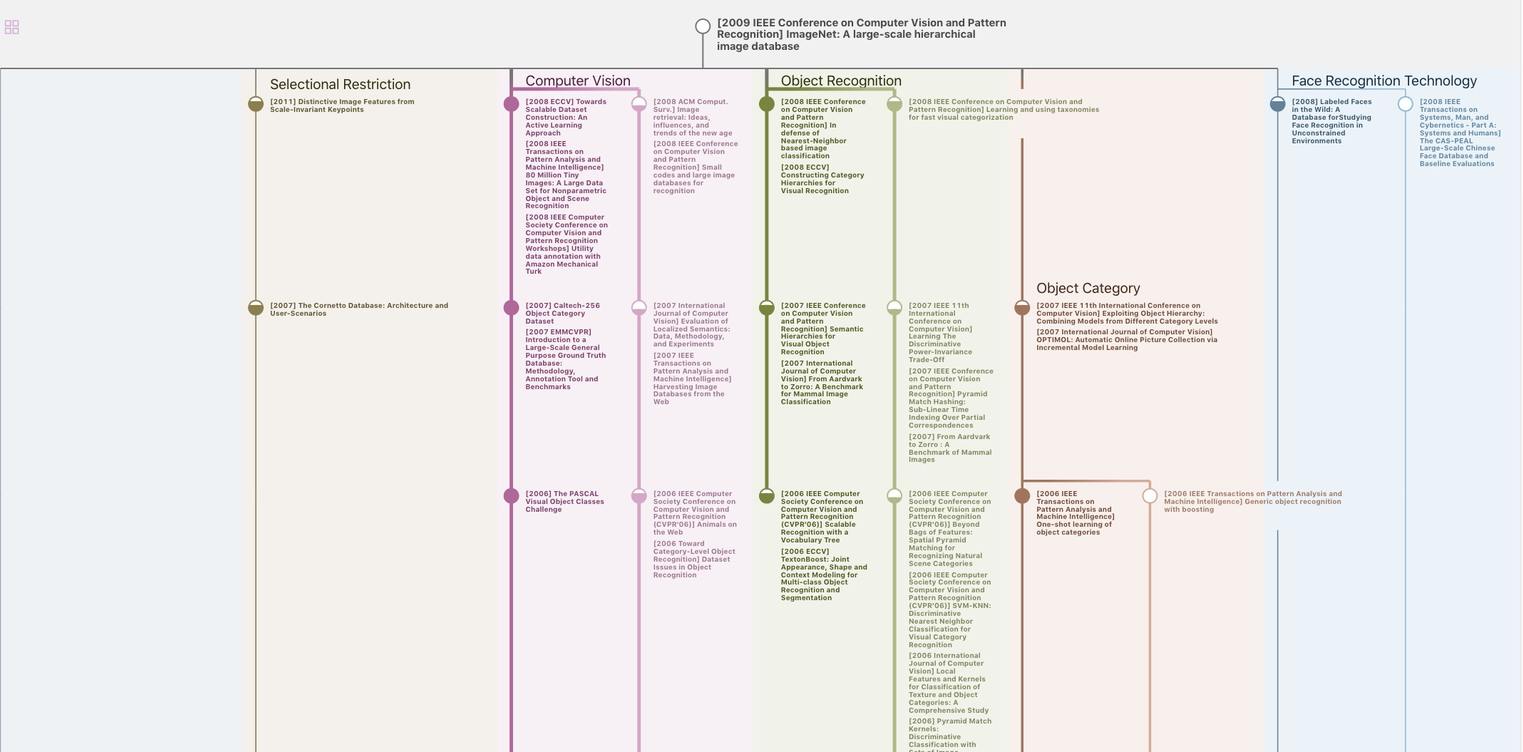
生成溯源树,研究论文发展脉络
Chat Paper
正在生成论文摘要