Reservoir-computing based associative memory for complex dynamical attractors
Research Square (Research Square)(2023)
摘要
Abstract Traditional neural-network models of associative memories were for storing and retrieving static patterns. We develop reservoir-computing based memories for complex dynamical attractors, under two common recalling scenarios in neuropsychology: location-addressable with an index channel and context-addressable without such a channel. We demonstrate that, for location-addressable retrieval, a single reservoir computing machine can memorize a large number of periodic and chaotic attractors, each retrievable with a specific index value. We articulate control strategies to achieve successful switching among the attractors. An algebraic scaling law between the number of stored attractors and the reservoir-network size is uncovered. For context-addressable retrieval, we exploit multistability with cue signals, where the stored attractors coexist in the high-dimensional phase space of the reservoir network. As the length of the cue signal increases through a critical value, a high success rate can be achieved. The work provides foundational insights into developing long-term memories for complex dynamical patterns.
更多查看译文
关键词
associative memory,reservoir-computing
AI 理解论文
溯源树
样例
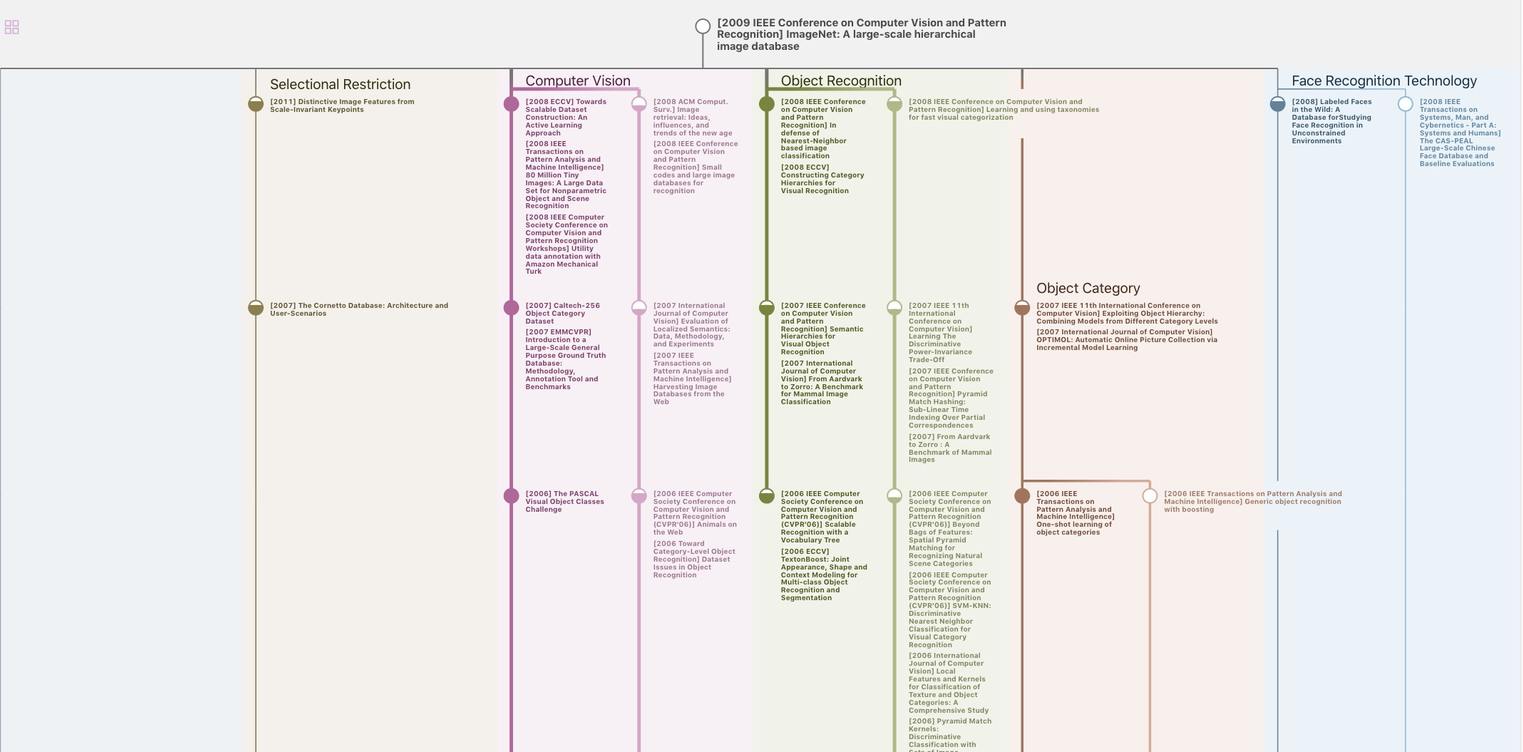
生成溯源树,研究论文发展脉络
Chat Paper
正在生成论文摘要