A Bayesian Semi-parametric Modelling Approach for Area Level Small Area Studies
Calcutta Statistical Association Bulletin(2023)
摘要
We present a new semiparametric extension of the Fay-Herriot model, termed the agnostic Fay-Herriot model (AGFH), in which the sampling-level model is expressed in terms of an unknown general function [Formula: see text]. Thus, the AGFH model can express any distribution in the sampling model since the choice of [Formula: see text] is extremely broad. We propose a Bayesian modelling scheme for AGFH where the unknown function [Formula: see text] is assigned a Gaussian Process prior. Using a Metropolis within Gibbs sampling Markov Chain Monte Carlo scheme, we study the performance of the AGFH model, along with that of a hierarchical Bayesian extension of the Fay-Herriot model. Our analysis shows that the AGFH is an excellent modelling alternative when the sampling distribution is non-Normal, especially in the case where the sampling distribution is bounded. It is also the best choice when the sampling variance is high. However, the hierarchical Bayesian framework and the traditional empirical Bayesian framework can be good modelling alternatives when the signal-to-noise ratio is high, and there are computational constraints. AMS subject classification: 62D05; 62F15
更多查看译文
关键词
area,bayesian,modelling,semi-parametric
AI 理解论文
溯源树
样例
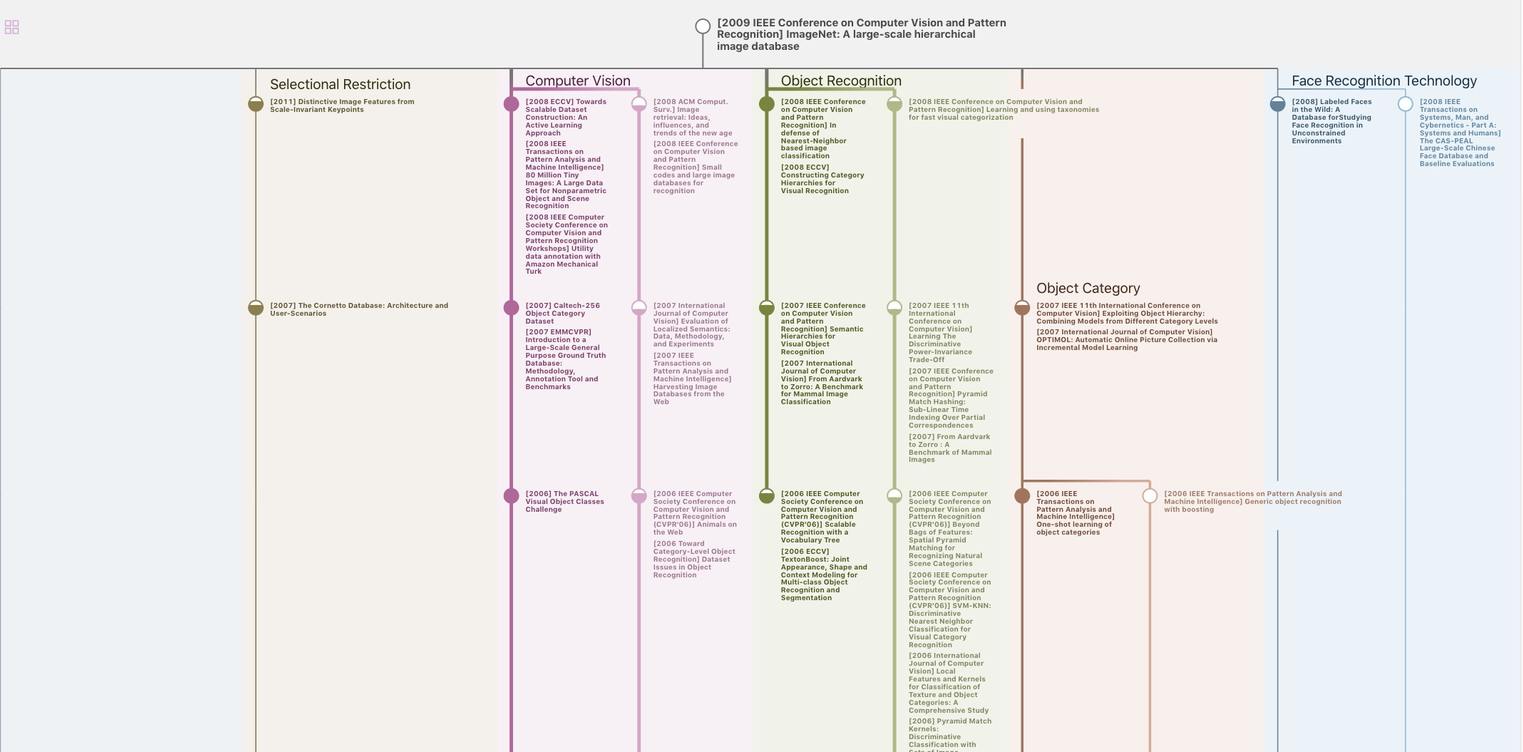
生成溯源树,研究论文发展脉络
Chat Paper
正在生成论文摘要