SpecTr: Spectral Transformer for Microscopic Hyperspectral Pathology Image Segmentation
IEEE Transactions on Circuits and Systems for Video Technology(2023)
摘要
Hyperspectral imaging (HSI) unlocks the huge potential to a wide variety of applications relying on high-precision pathology image segmentation, such as computational pathology. It can acquire biochemical properties even invisible to naked eyes from histological specimens. Since 1) spectra contain discriminative and continuous patterns for differentiating tissues/cells, and 2) the discriminability of spectra relies on both fine-grained relations in the high-resolution spectrum and coarse relations in the low-resolution spectrum, the key to achieving high-precision hyperspectral pathology image segmentation is to felicitously model the intra- and inter-scale context especially for spectra. In this paper, we propose a spectral transformer (SpecTr) for hyperspectral pathology image segmentation, which first captures global context for intra-scale spectral features, and subsequently extract coarse and fine-grained discriminative spectral information from inter-scale features, respectively. To learn intra-scale spectral context, we propose a Spectral Attentive Module (SAM). Unlike the existing Transformer model that is designed for modalities such as natural images, our proposed SAM is efficient in capturing sparse and pivotal spectral context while avoiding the heterogeneous underlying distributions and noises of different bands. Besides, to reduce the computational complexity of the HSI segmentation model, we further propose a global-local attention module to effectively learn a condensed spectral feature. Experiments show that HSIs can become a more powerful image modality for understanding microscopic pathology images than RGB images, and the proposed SpecTr outperforms other competing methods for hyperspectral pathology image segmentation, with an improvement of 3% compared with the popular 3D-nnUNet and other transformer-based methods. Our code is available at https://github.com/DeepMed-Lab-ECNU/SpecTr.
更多查看译文
关键词
Hyperspectral pathology image,Medical image segmentation,Transformer,Optical imaging,Microscopy
AI 理解论文
溯源树
样例
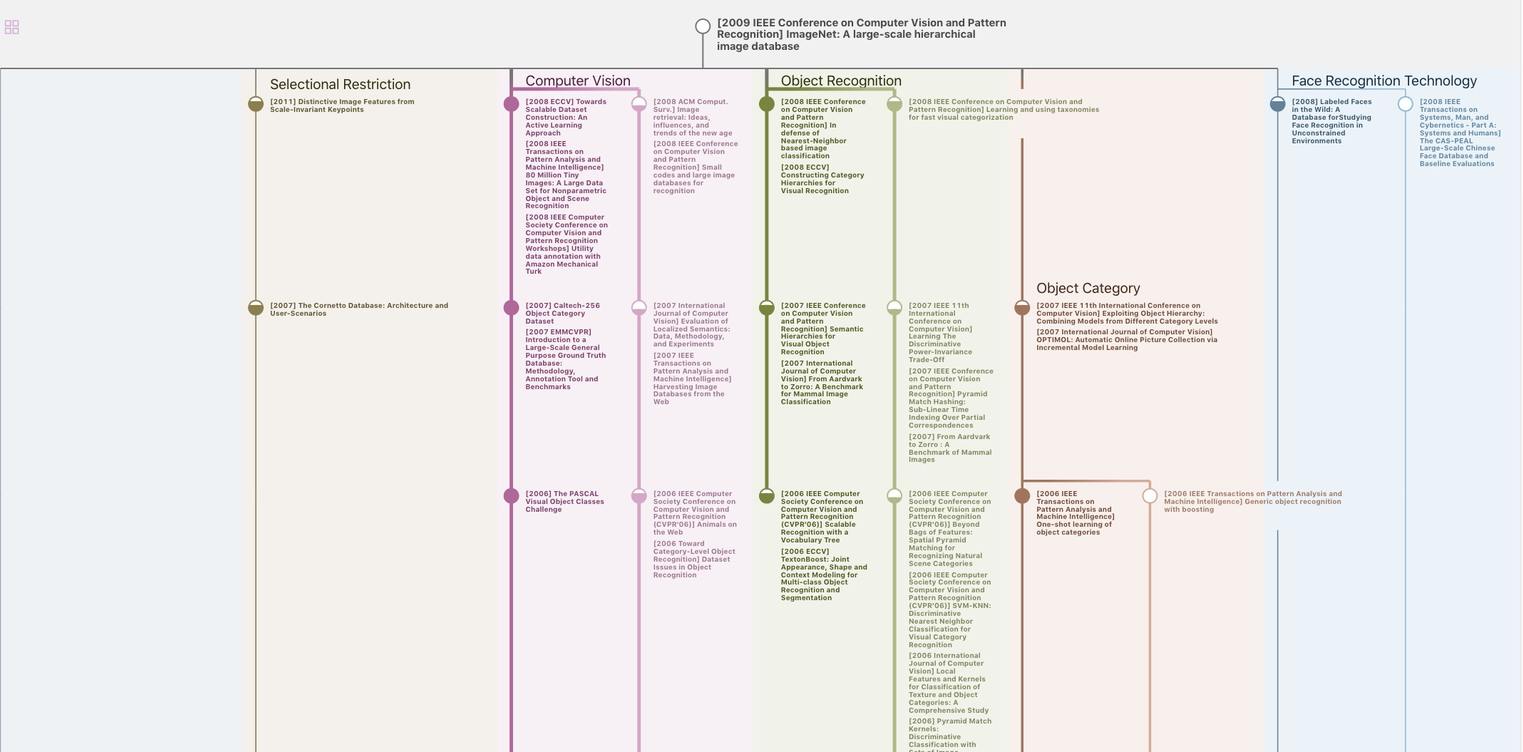
生成溯源树,研究论文发展脉络
Chat Paper
正在生成论文摘要