Multi-Agent Deep Reinforcement Learning Based UAV Trajectory Optimization for Differentiated Services.
IEEE Trans. Mob. Comput.(2024)
摘要
Driven by the increasing computational demand of real-time mobile applications, Unmanned Aerial Vehicle (UAV) assisted Multi-access Edge Computing (MEC) has been envisioned as a promising paradigm for pushing computational resources to network edges and constructing high-throughput line-of-sight links for ground users. Most exsiting studies consider simplified scenarios, such as a single UAV, Service Provider (SP) or service type, and centralized UAV trajectory control. In order to be more in line with real-world cases, we intend to achieve distributed trajectory control of multiple UAVs in UAV-assisted MEC networks with multiple SPs providing differentiated services. Our objective is to minimize the short-term computational costs of ground users and the long-term computational cost of UAVs, simultaneously based on incomplete information. We first solve the formulated problem by reaching the Nash Equilibrium (NE) of the game among SPs based on complete information. We further formulate a Markov game model and propose a Deep Reinforcement Learning (DRL)-based UAV trajectory optimization algorithm, where only local observations of each UAV are required for each SP's flying action execution. Theoretical analysis and performance evaluation demonstrate the convergence, efficiency, scalability, and robustness of our algorithm compared with other representative algorithms.
更多查看译文
关键词
Multi-access edge computing,UAV-assisted communications,game theory,multi-agent DRL
AI 理解论文
溯源树
样例
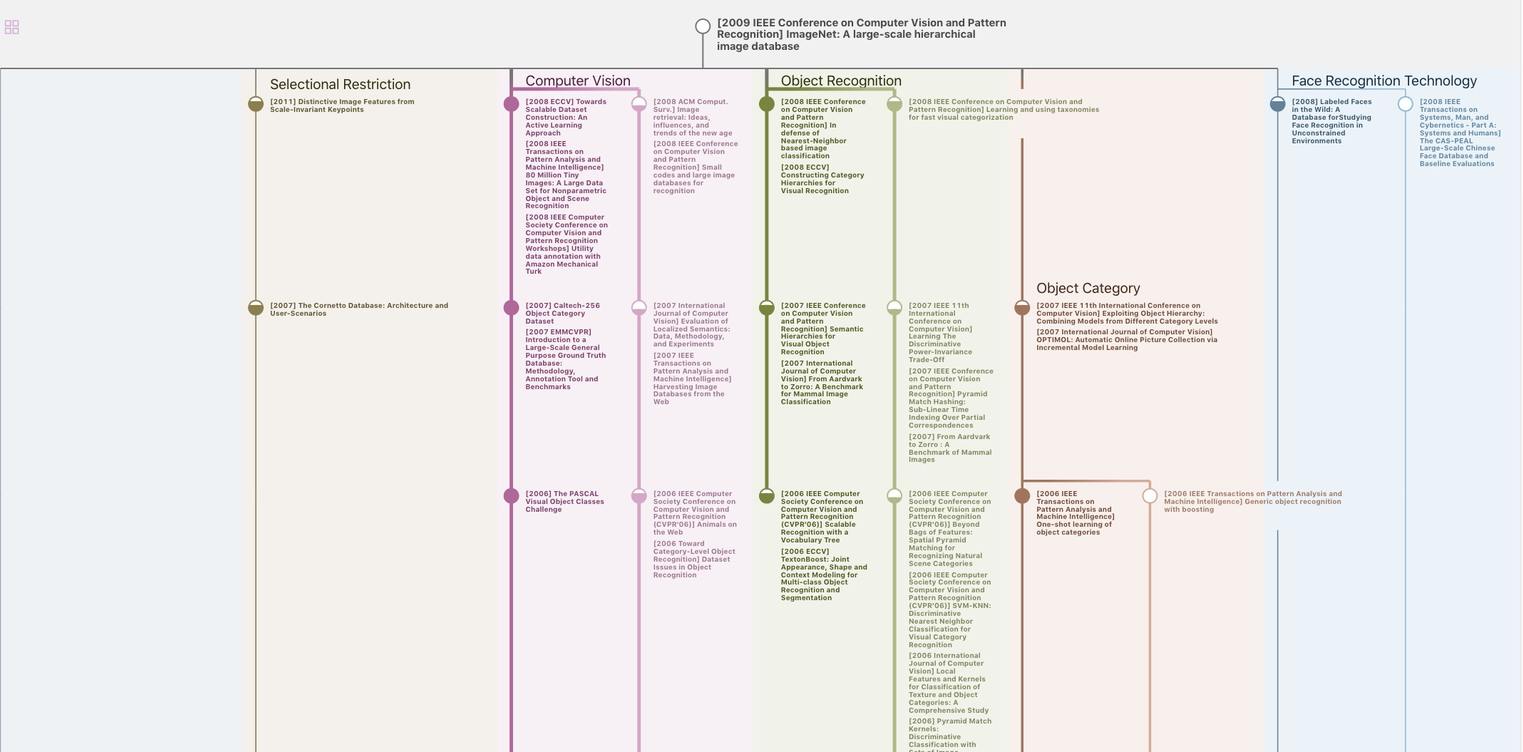
生成溯源树,研究论文发展脉络
Chat Paper
正在生成论文摘要