Physics-informed Graphical Learning and Bayesian Averaging for Robust Distribution State Estimation
IEEE Transactions on Power Systems(2023)
摘要
This paper proposes a robust topology change-aware distribution system state estimation (DSSE) method based on a physics-informed graph neural network and Bayesian Probability Weighted Averaging (BPWA). A general state estimator is first built utilizing a graph attention network to learn the nonlinear mapping functions under different distribution network topologies. During this stage, the topology information is embedded in the neural network and the attention mechanism is employed to capture collaborative signals and discriminate the importance of neighboring buses. Then, the BPWA method allows assigning proper weights for the state estimation results under different topologies, which finally yields a single consensus solution via the sparse training samples under the new topology. The physics-informed mechanism enables the proposed method to embed the topology knowledge in the neural network while fully exploiting the value of historical data. Robustness to anomalous measurements is achieved through the embedding of physics knowledge. The application of the BPWA method further allows the proposed method to achieve faster adaptation to topology change and quantification of the estimation uncertainties by measurement errors. MATLAB and Python are used to carry out the comparative tests to evaluate the performance of the proposed method.
更多查看译文
关键词
Distribution system state estimation,physics-informed learning,anomalous measurements,topology change
AI 理解论文
溯源树
样例
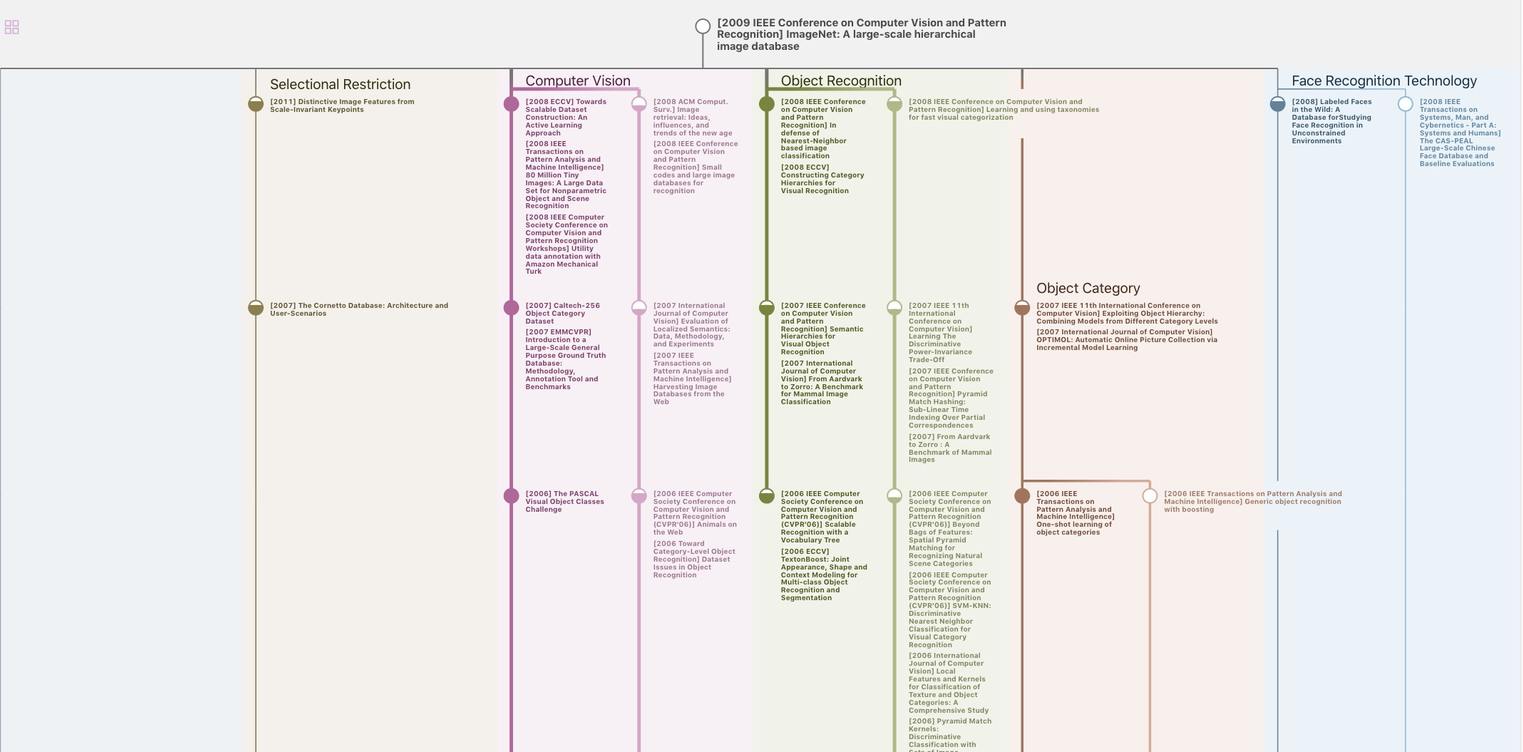
生成溯源树,研究论文发展脉络
Chat Paper
正在生成论文摘要