Trimap-guided feature mining and fusion network for natural image matting
Computer Vision and Image Understanding(2023)
摘要
Mining interesting objects with trimap guidance and fusing multi-level features are two important issues for trimap-based matting. For feature mining, existing methods simply feed trimaps to an encoder network and mine features evenly in different regions with a decoding module, which lacks an efficient and effective way to mine interesting objects pointed out by trimaps. For emerging content-based feature fusion, most existing matting methods only focus on local features which lack the guidance of a global feature with strong semantic information related to the interesting object. In this paper, we propose a trimap-guided feature mining and fusion network consisting of our trimap-guided non-background multi-scale pooling (TMP) module and global-local context-aware fusion (GLF) modules. Considering that trimap provides strong semantic guidance, our TMP module focuses effective feature mining on interesting objects under the guidance of trimap without extra parameters. Furthermore, our GLF modules use global semantic information of interesting objects mined by our TMP module to guide an effective global-local context-aware multi-level feature fusion. In addition, we build a common interesting object matting (CIOM) dataset to advance high-quality image matting. Experimental results on the Composition-1k test set, Alphamatting benchmark, and our CIOM test set demonstrate that our method outperforms state-of-the-art approaches. Codes and models will be publicly available at https://github.com/Serge-weihao/TMF-Matting.
更多查看译文
关键词
natural image,fusion network,trimap-guided
AI 理解论文
溯源树
样例
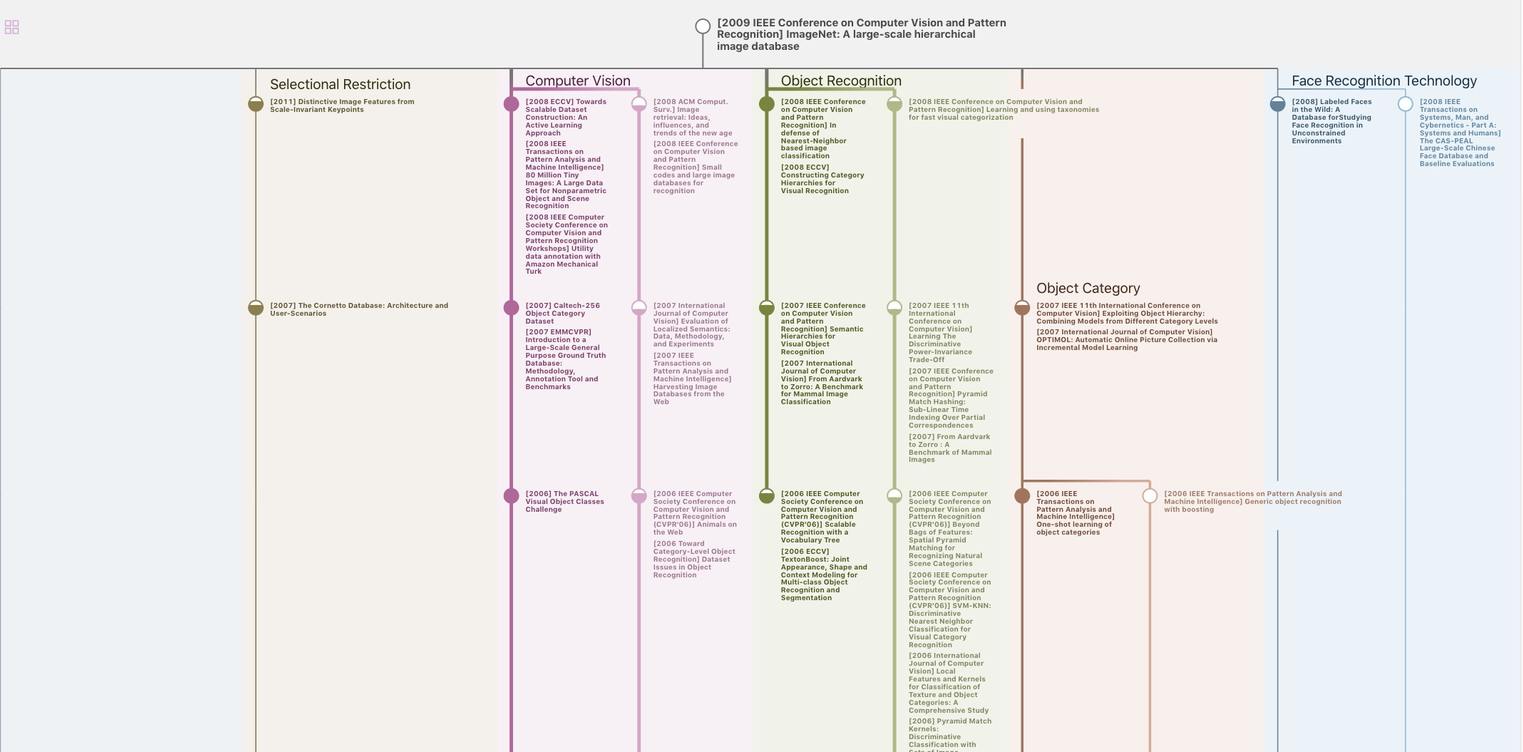
生成溯源树,研究论文发展脉络
Chat Paper
正在生成论文摘要