Balanced segmentation of CNNs for multi-TPU inference
Research Square (Research Square)(2023)
摘要
Abstract In this paper, we propose different alternatives for CNN (Convolutional Neural Networks) segmentation, addressing inference processes on computing architectures composed by multiple Edge TPUs. Specifically, we compare the inference performance for a number of state-of-the-art CNN models taking as a reference inference times on one TPU and a compiler-based pipelined inference implementation as provided by the Google's Edge TPU compiler. Departing from a profiled-based segmentation strategy, we provide further refinements to balance the workload across multiple TPUs, leveraging their co-operative computing power, reducing work imbalance and alleviating the memory access bottleneck due to the limited amount of on-chip memory per TPU. The observed performance results compared with a single TPU yield super-linear speedups and accelerations up to 2.60x compared with the segmentation offered by the compiler targeting multiple TPUs.
更多查看译文
关键词
balanced segmentation,cnns,multi-tpu
AI 理解论文
溯源树
样例
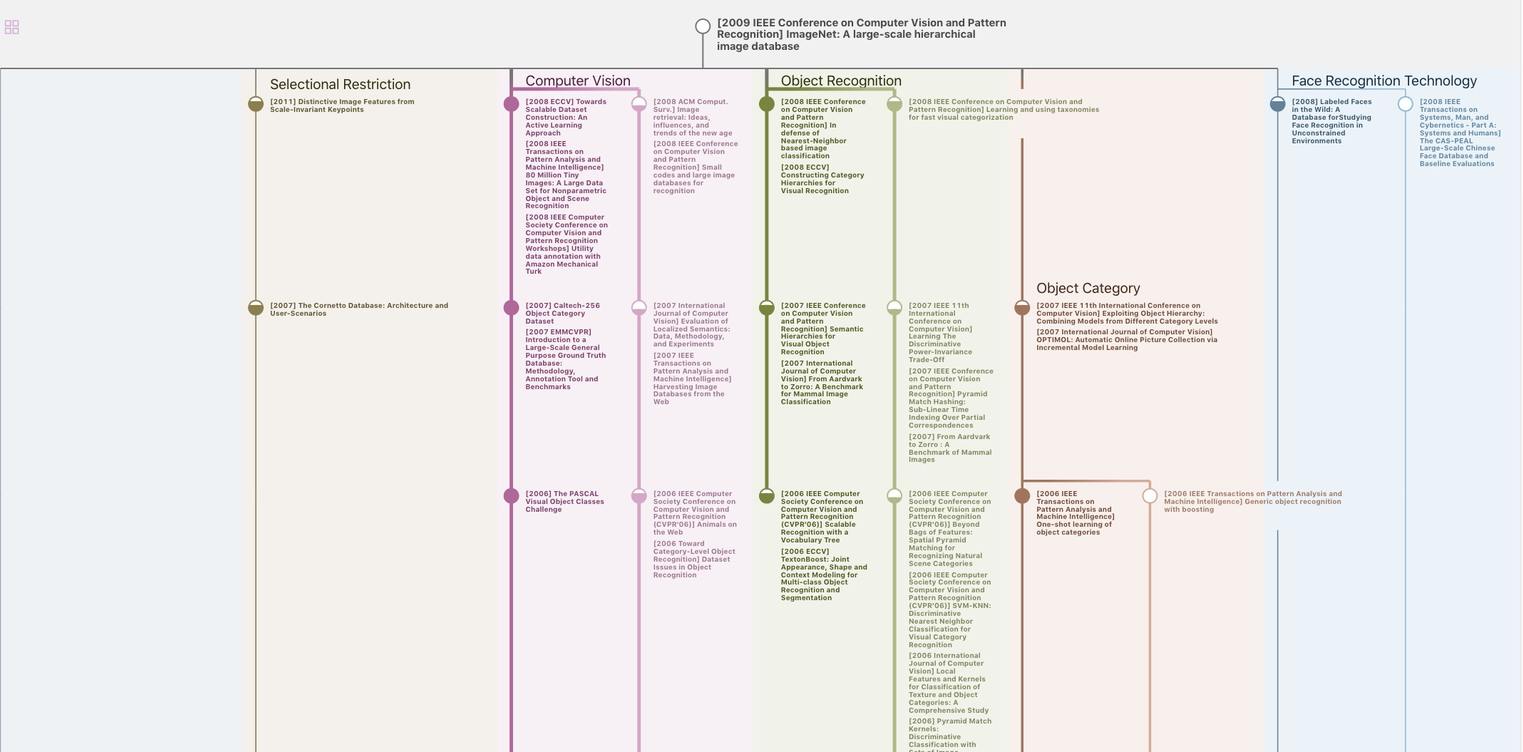
生成溯源树,研究论文发展脉络
Chat Paper
正在生成论文摘要