Accurate Knowledge Distillation with n-best Reranking
arXiv (Cornell University)(2023)
摘要
We propose utilizing n-best reranking to enhance the Sequence-Level Knowledge Distillation (Kim and Rush, 2016) where we explore hypotheses beyond the top-1 to acquire more accurate pseudo-labels. To accomplish this, we leverage a diverse set of models with different inductive biases, objective functions or architectures, including publicly-available large pretrained models. The effectiveness of our proposal is validated through experiments on the WMT'21 German-English and Chinese-English translation tasks. Our results demonstrate that utilizing the pseudo-labels generated by our n-best reranker leads to a significantly more accurate student model. In fact, our best student model achieves comparable accuracy to a large translation model from (Tran et al., 2021) with 4.7 billion parameters, while having two orders of magnitude fewer parameters.
更多查看译文
关键词
distillation,knowledge,n-best
AI 理解论文
溯源树
样例
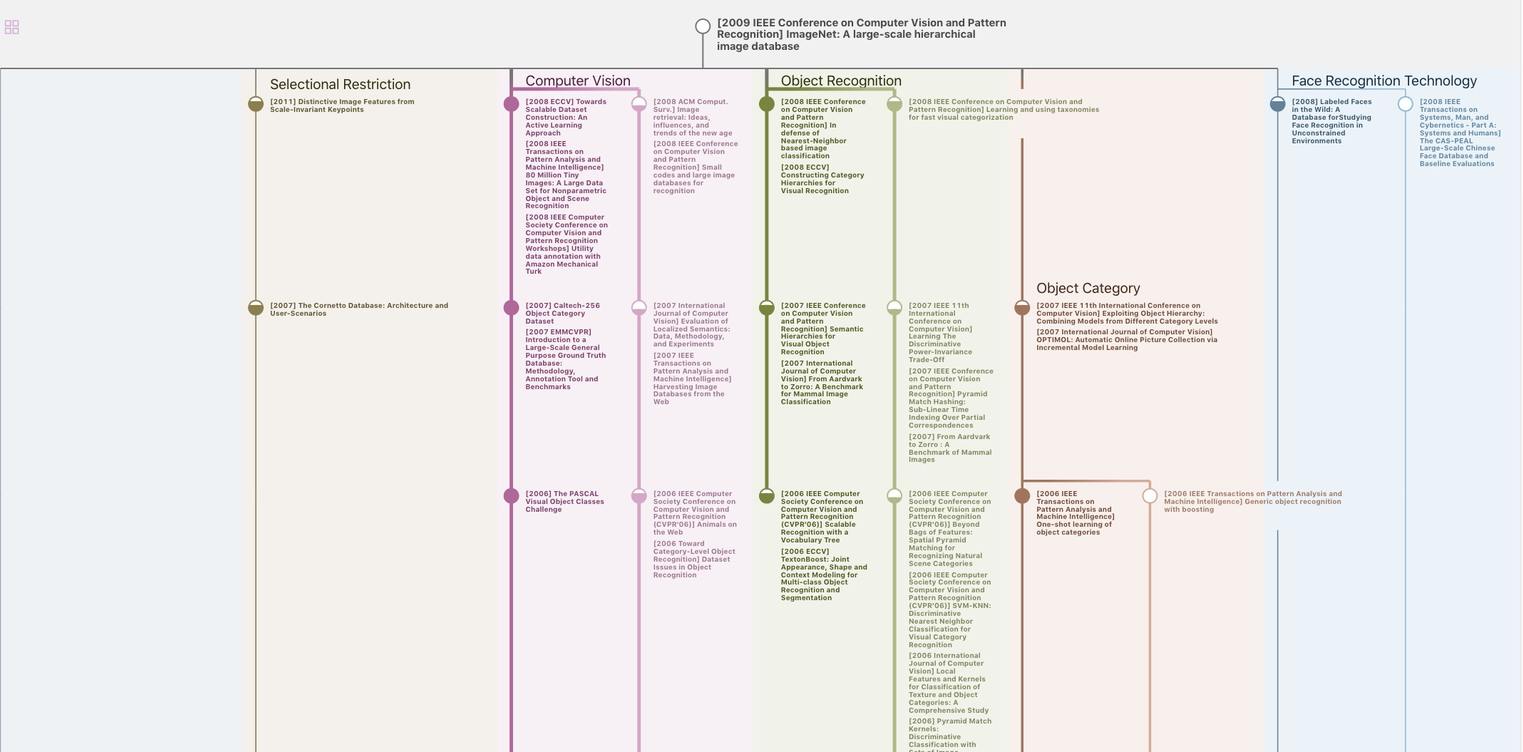
生成溯源树,研究论文发展脉络
Chat Paper
正在生成论文摘要