Abstract 4257: Using Multilevel Regression and Poststratification (MRP) to inform estimates of biomarker prevalence for target populations of interest using linked clinico-genomic databases
Cancer Research(2023)
摘要
Abstract Background Contemporary evidence on the size of biomarker-defined cancer populations is crucial for clinical and economic healthcare decision makers. Estimates can be derived from real-world data (RWD), but may be affected by biases stemming from differences in observed populations vs. general populations of patients under the purview of decision makers. These differences may be driven by patient or clinical characteristics, as well as differences in assays used to assess biomarker status. This study demonstrates the applicability of an emerging method in survey science (Multilevel Regression and Poststratification (MRP)), which leverages common covariates across source data and larger, more representative set of observations to inform estimates of biomarker prevalence for target populations of interest. Methods In disease-agnostic cohorts of patients with solid-tumor cancers from two, large clinico-genomic cancer databases (AACR GENIE 12.0, and nationwide (US-based) de-identified Flatiron Health - Foundation Medicine Clinico-Genomic Database(CGDB)), we used covariate-adjusted regression (age, sex, race, stage at diagnosis (for CGDB patients), and cancer type) to predict the probability of a patient having an NTRK fusion and/or a BRAF V600E mutation detected in their sequenced cancer tissue. AACR Project GENIE (n = 118,649) is a cancer registry of real-world data assembled through data sharing between 19 international cancer centers. The de-identified data in the CGDB (n = 91,719) originated from approximately 280 US cancer clinics (~800 sites of care).We then implemented post-stratification adjustments (Gelman, 2006; Downes 2018) using the Surveillance, Epidemiology, and End Results (SEER) 17 2022 cancer registry to estimate prevalence of the alterations in the large, population-based registry. Results Prevalence estimates were generally consistent between sources. Adjusted prevalence (%) estimates for NTRK Fusion (AACR: 0.25% (raw), adjusted to 0.21% (0.01%-0.48%) (MRP-adjusted mean % (95% quantile intervals)); CGDB: 0.29, adjusted to 0.26 (0.02-0.63), and for BRAF V600E (AACR: 2.19, adjusted to 1.72 (0.77-2.66); CGDB: 2.44, adjusted to 2.68 (1.50-3.89)) reflected differential patient characteristics (predominantly tumor type) in these sources compared to SEER. MRP also enabled creation, from posterior distributions, of tumor-level estimates, along with credible interval bounds. Conclusion While both clinico-genomic databases offered generally consistent estimates of biomarker prevalence, representativeness methods that are used in the survey literature may be suited for addressing biases in RWD sources to improve biomarker prevalence estimates of target populations of patients with cancer. Citation Format: Jeremy W. Snider, Brennan Beal, Lilia Bouzit, Cheryl Cho-Phan, Leah Comment, Garrett Frampton, Tamara Snow. Using Multilevel Regression and Poststratification (MRP) to inform estimates of biomarker prevalence for target populations of interest using linked clinico-genomic databases. [abstract]. In: Proceedings of the American Association for Cancer Research Annual Meeting 2023; Part 1 (Regular and Invited Abstracts); 2023 Apr 14-19; Orlando, FL. Philadelphia (PA): AACR; Cancer Res 2023;83(7_Suppl):Abstract nr 4257.
更多查看译文
关键词
biomarker prevalence,multilevel regression,target populations,clinico-genomic
AI 理解论文
溯源树
样例
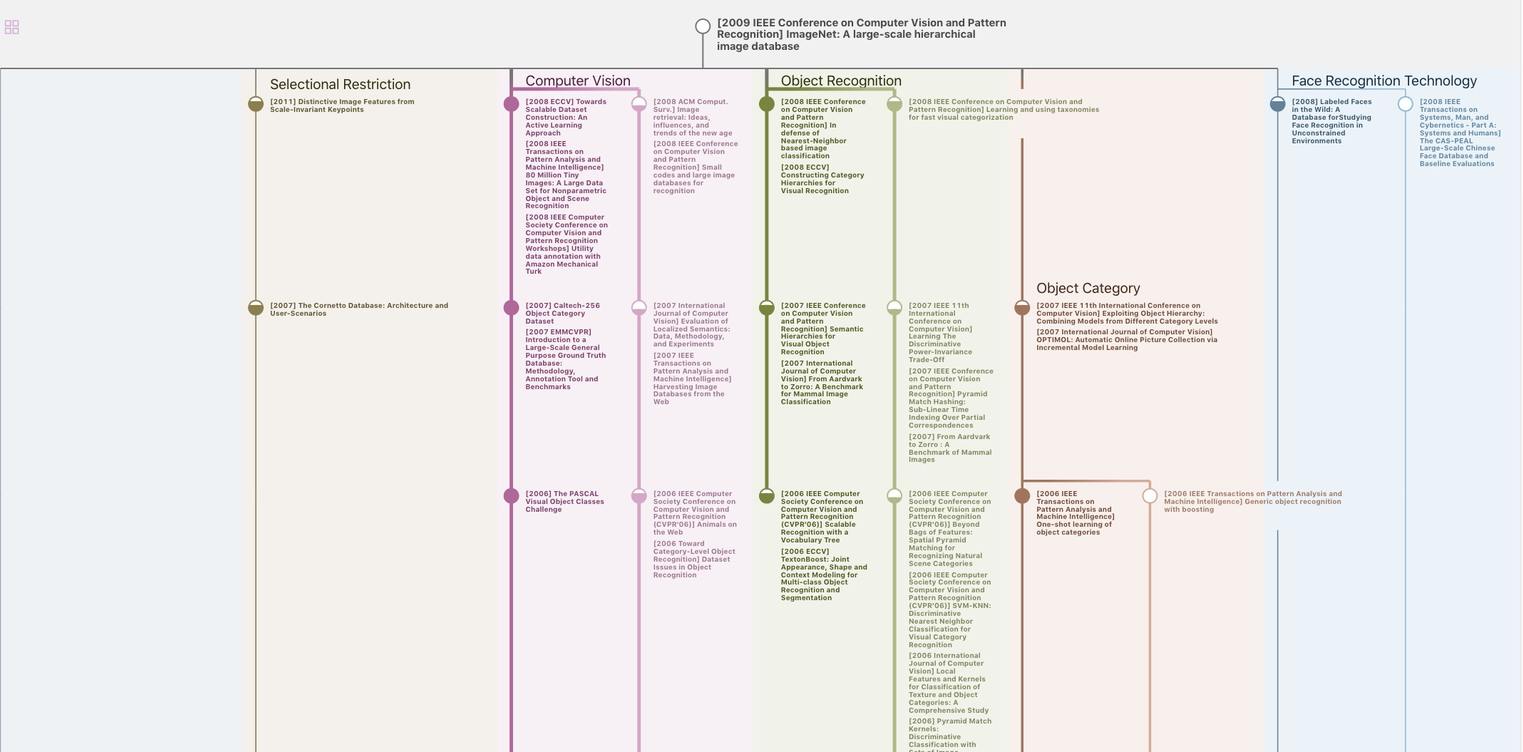
生成溯源树,研究论文发展脉络
Chat Paper
正在生成论文摘要