Predicting Radiologists' Gaze With Computational Saliency Models in Mammogram Reading
IEEE TRANSACTIONS ON MULTIMEDIA(2024)
摘要
Previous studies have shown that there is a strong correlation between radiologists' diagnoses and their gaze when reading medical images. The extent to which gaze is attracted by content in a visual scene can be characterised as visual saliency. There is a potential for the use of visual saliency in computer-aided diagnosis in radiology. However, little is known about what methods are effective for diagnostic images, and how these methods could be adapted to address specific applications in diagnostic imaging. In this study, we investigate 20 state-of-the-art saliency models including 10 traditional models and 10 deep learning-based models in predicting radiologists' visual attention while reading 196 mammograms. We found that deep learning-based models represent the most effective type of methods for predicting radiologists' gaze in mammogram reading; and that the performance of these saliency models can be significantly improved by transfer learning. In particular, an enhanced model can be achieved by pre-training the model on a large-scale natural image saliency dataset and then fine-tuning it on the target medical image dataset. In addition, based on a systematic selection of backbone networks and network architectures, we proposed a parallel multi-stream encoded model which outperforms the state-of-the-art approaches for predicting saliency of mammograms.
更多查看译文
关键词
Deep learning,mammograms,radiology,saliency,transfer learning
AI 理解论文
溯源树
样例
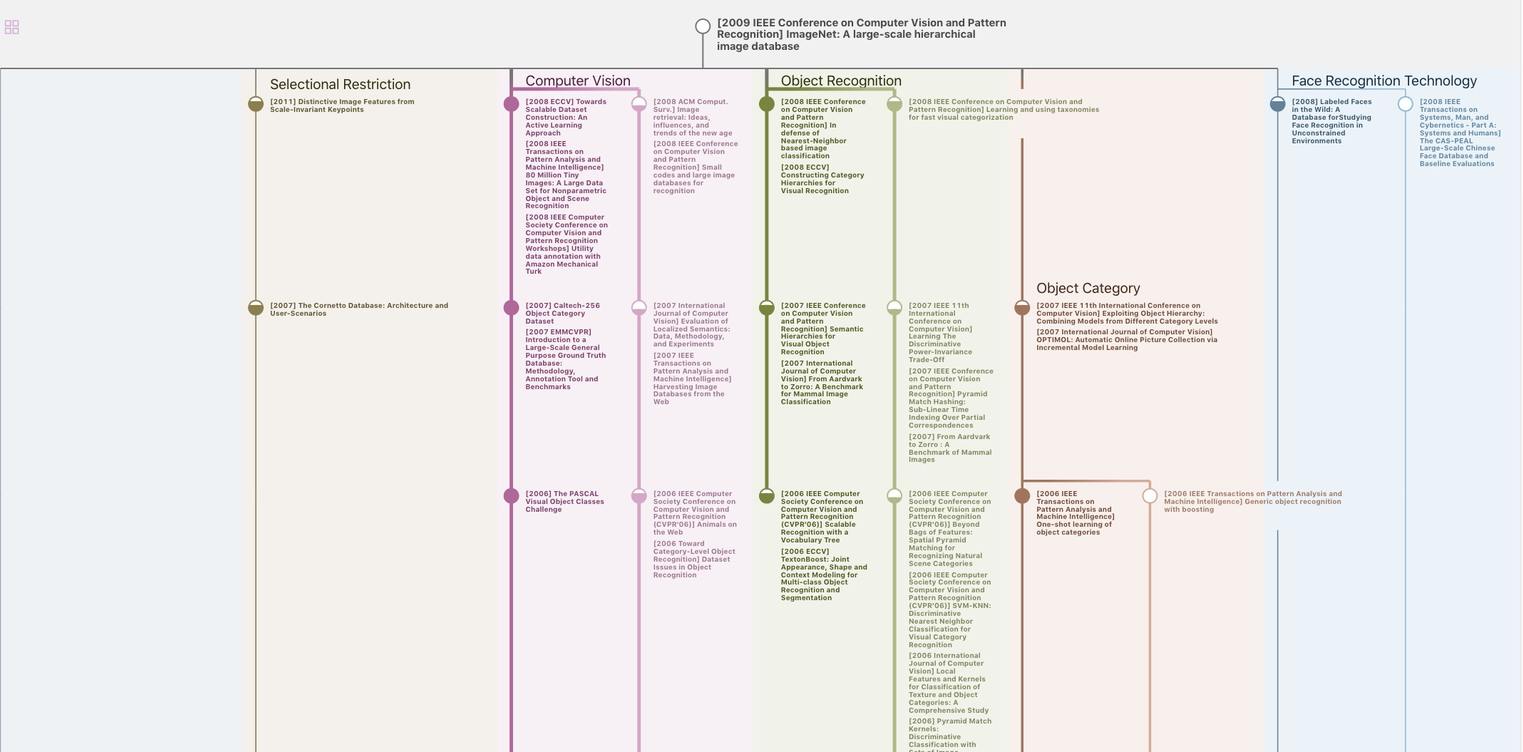
生成溯源树,研究论文发展脉络
Chat Paper
正在生成论文摘要