MRI Reconstruction with Side Information using Diffusion Models.
Asilomar Conference on Signals, Systems and Computers(2023)
摘要
Magnetic resonance imaging (MRI) exam protocols consist of multiple contrast-weighted images of the same anatomy to emphasize different tissue properties. Due to the long acquisition times required to collect fully sampled k-space measurements, it is common to only collect a fraction of k-space for each scan and subsequently solve independent inverse problems for each image contrast. Recently, there has been a push to further accelerate MRI exams using data-driven priors, and generative models in particular, to regularize the ill-posed inverse problem of image reconstruction. These methods have shown promising improvements over classical methods. However, many of the approaches neglect the additional information present in a clinical MRI exam like the multi-contrast nature of the data and treat each scan as an independent reconstruction. In this work we show that by learning a joint Bayesian prior over multi-contrast data with a score-based generative model we are able to leverage the underlying structure between random variables related to a given imaging problem. This leads to an improvement in image reconstruction fidelity over generative models that rely only on a marginal prior over the image contrast of interest.
更多查看译文
关键词
Magnetic Resonance Imaging (MRI),Multi-Contrast,Compressed sensing,Generative Models,Bayesian reconstruction
AI 理解论文
溯源树
样例
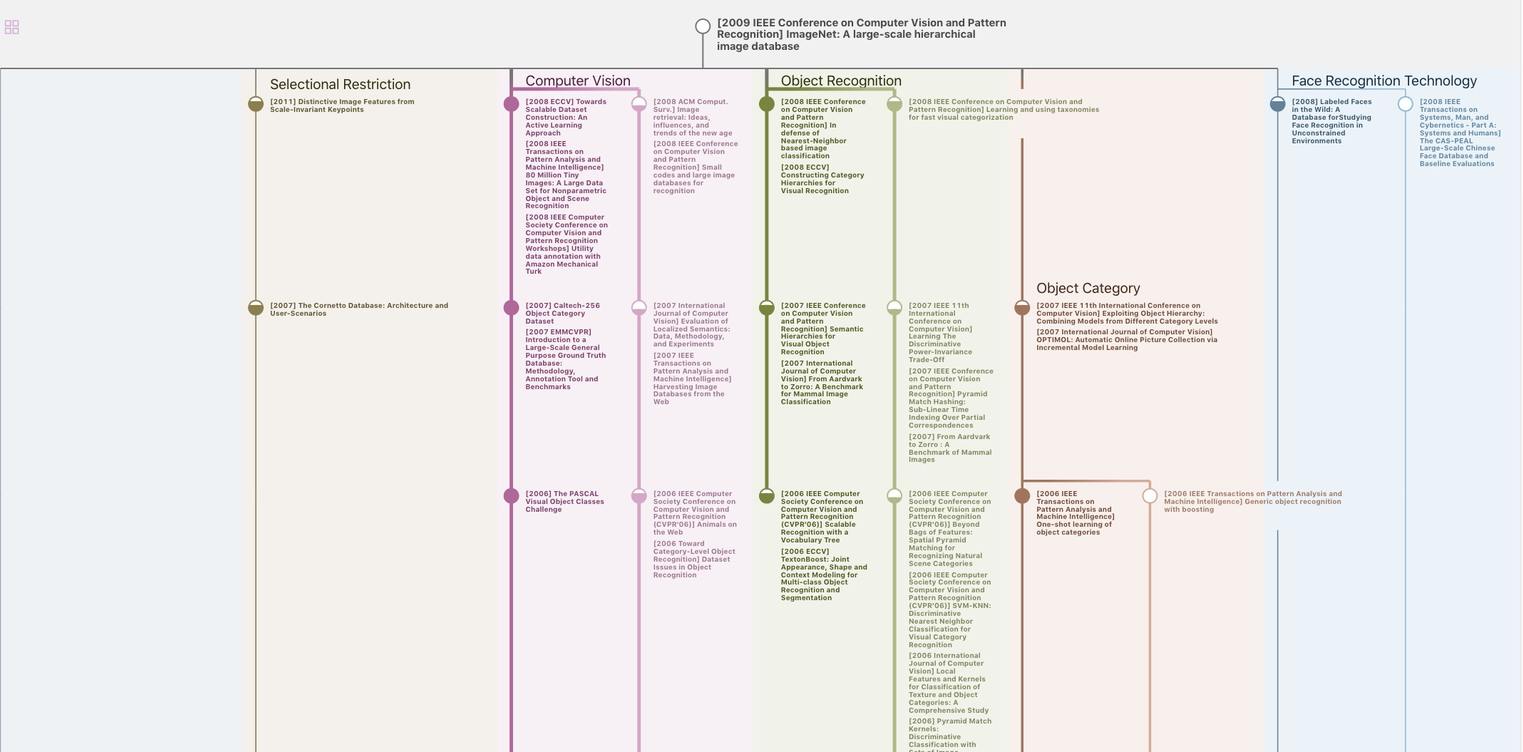
生成溯源树,研究论文发展脉络
Chat Paper
正在生成论文摘要