Discouraging posterior collapse in hierarchical Variational Autoencoders using context
arXiv (Cornell University)(2023)
摘要
Hierarchical Variational Autoencoders (VAEs) are among the most popular likelihood-based generative models. There is a consensus that the top-down hierarchical VAEs allow effective learning of deep latent structures and avoid problems like posterior collapse. Here, we show that this is not necessarily the case, and the problem of collapsing posteriors remains. To discourage this issue, we propose a deep hierarchical VAE with a context on top. Specifically, we use a Discrete Cosine Transform to obtain the last latent variable. In a series of experiments, we observe that the proposed modification allows us to achieve better utilization of the latent space and does not harm the model's generative abilities.
更多查看译文
关键词
hierarchical variational autoencoders,posterior collapse,context
AI 理解论文
溯源树
样例
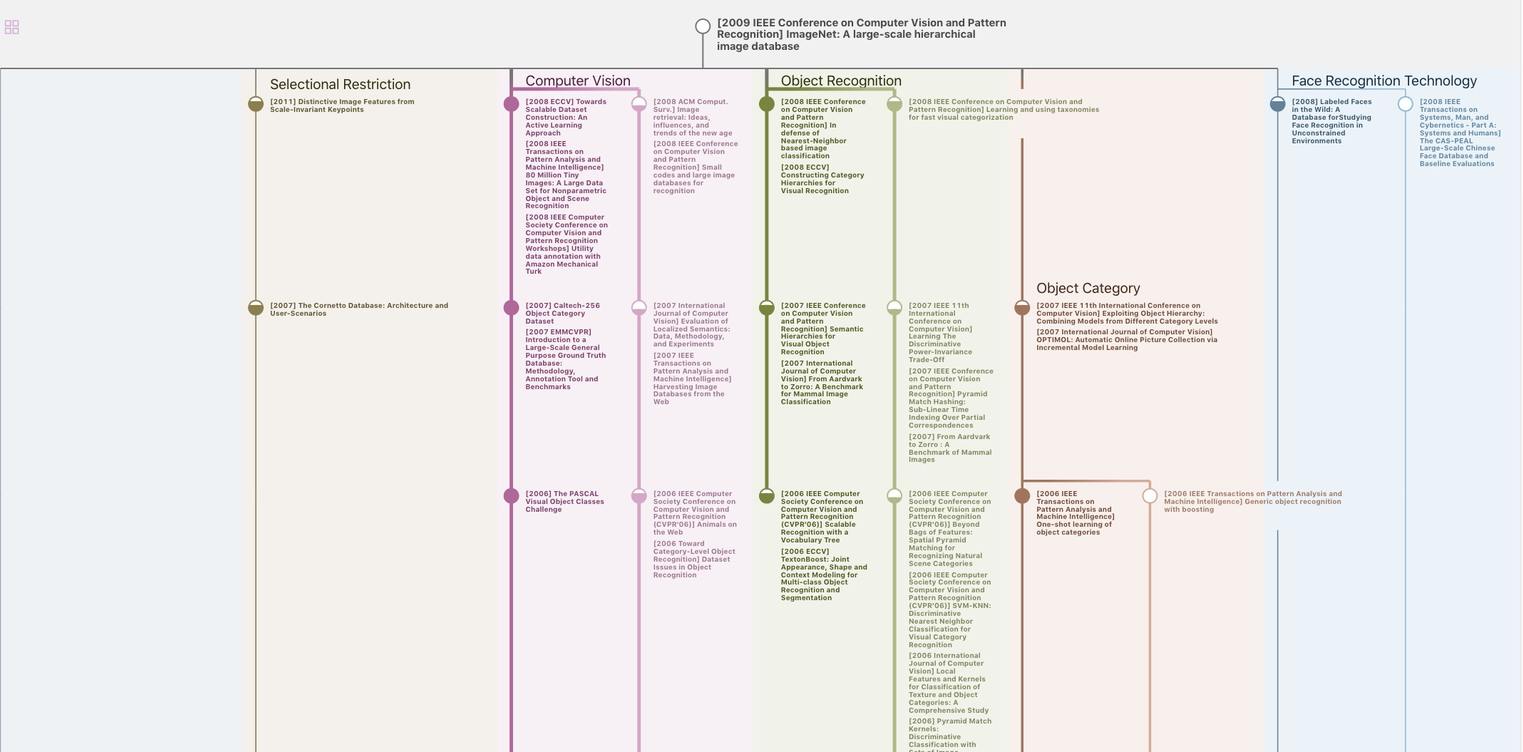
生成溯源树,研究论文发展脉络
Chat Paper
正在生成论文摘要