Ordinal Regression for Difficulty Estimation of StepMania Levels
arXiv (Cornell University)(2023)
摘要
StepMania is a popular open-source clone of a rhythm-based video game. As is common in popular games, there is a large number of community-designed levels. It is often difficult for players and level authors to determine the difficulty level of such community contributions. In this work, we formalize and analyze the difficulty prediction task on StepMania levels as an ordinal regression (OR) task. We standardize a more extensive and diverse selection of this data resulting in five data sets, two of which are extensions of previous work. We evaluate many competitive OR and non-OR models, demonstrating that neural network-based models significantly outperform the state of the art and that StepMania-level data makes for an excellent test bed for deep OR models. We conclude with a user experiment showing our trained models' superiority over human labeling.
更多查看译文
关键词
stepmania levels,difficulty estimation,ordinal regression
AI 理解论文
溯源树
样例
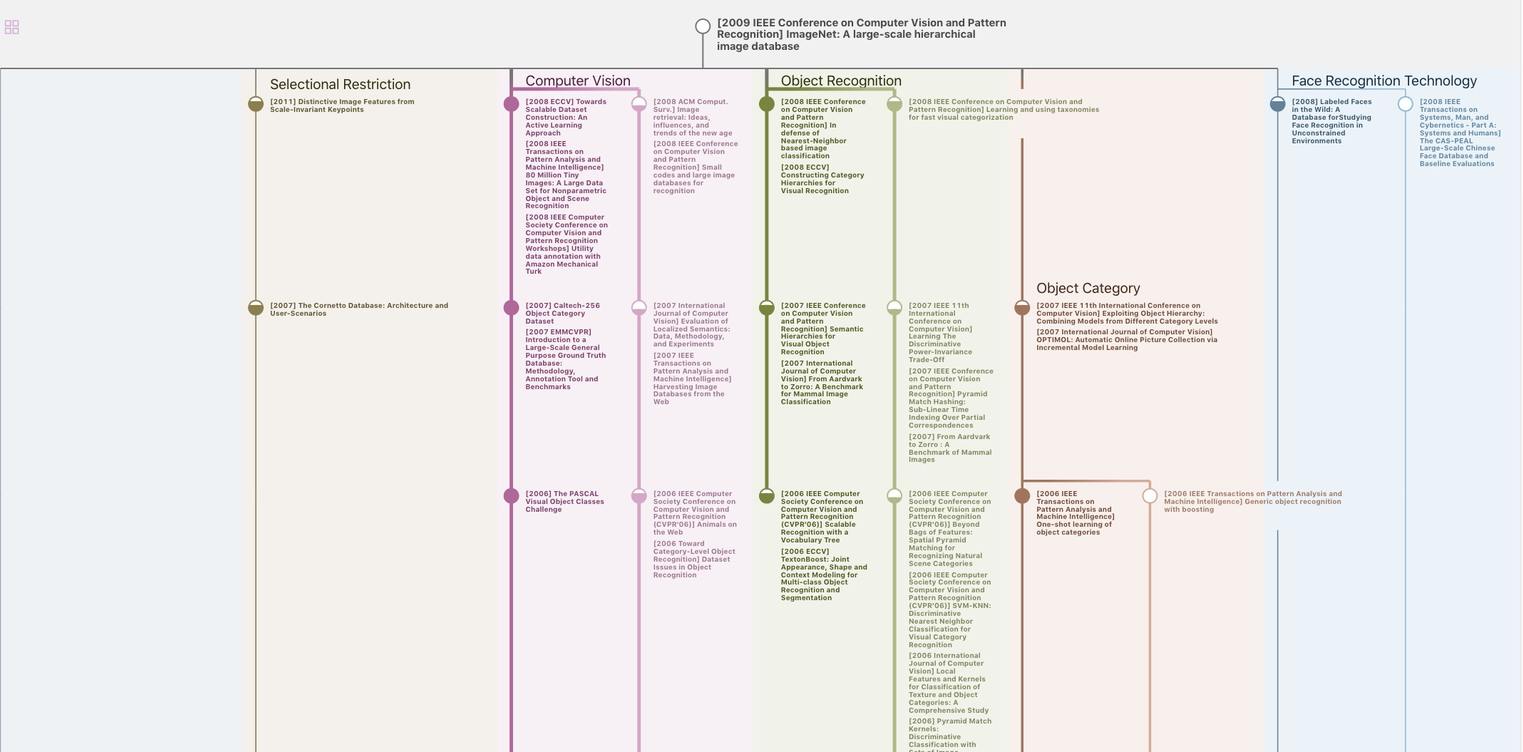
生成溯源树,研究论文发展脉络
Chat Paper
正在生成论文摘要