Credible Remote Sensing Scene Classification Using Evidential Fusion on Aerial-Ground Dual-view Images
arXiv (Cornell University)(2023)
摘要
Due to their ability to offer more comprehensive information than data from a single view, multi-view (multi-source, multi-modal, multi-perspective, etc.) data are being used more frequently in remote sensing tasks. However, as the number of views grows, the issue of data quality becomes more apparent, limiting the potential benefits of multi-view data. Although recent deep neural network (DNN) based models can learn the weight of data adaptively, a lack of research on explicitly quantifying the data quality of each view when fusing them renders these models inexplicable, performing unsatisfactorily and inflexible in downstream remote sensing tasks. To fill this gap, in this paper, evidential deep learning is introduced to the task of aerial-ground dual-view remote sensing scene classification to model the credibility of each view. Specifically, the theory of evidence is used to calculate an uncertainty value which describes the decision-making risk of each view. Based on this uncertainty, a novel decision-level fusion strategy is proposed to ensure that the view with lower risk obtains more weight, making the classification more credible. On two well-known, publicly available datasets of aerial-ground dual-view remote sensing images, the proposed approach achieves state-of-the-art results, demonstrating its effectiveness. The code and datasets of this article are available at the following address: https://github.com/gaopiaoliang/Evidential.
更多查看译文
关键词
remote sensing,evidential fusion,classification,aerial-ground,dual-view
AI 理解论文
溯源树
样例
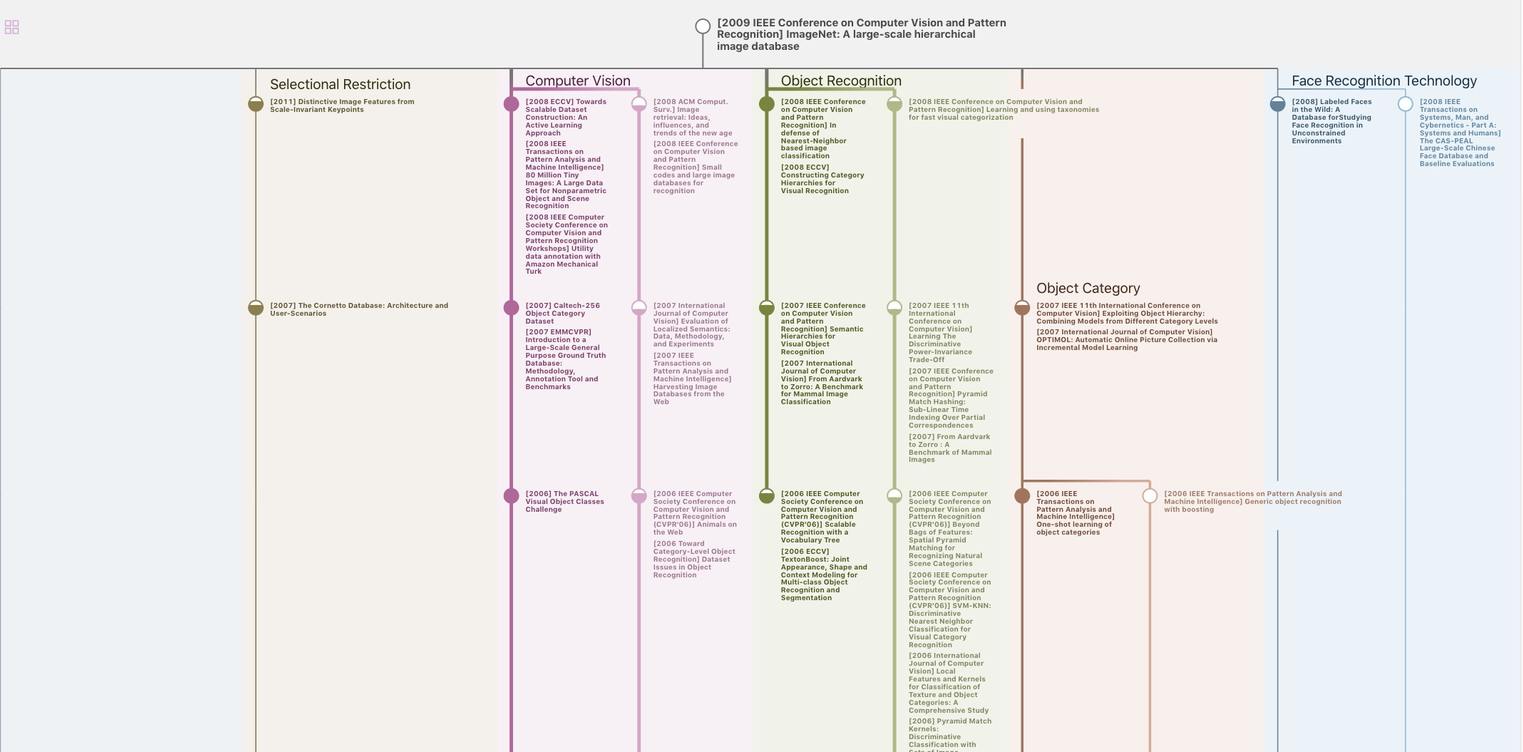
生成溯源树,研究论文发展脉络
Chat Paper
正在生成论文摘要