Population data-based federated machine-learning improves automated echocardiographic quantification of cardiac structure and function – the AVE project
European heart journal(2023)
摘要
Abstract Background Machine-learning (ML)-based automated measurement of echocardiography images emerged as an option to reduce observer variability. Objectives To improve the accuracy of a pre-existing automated reading tool (“original detector”) by federated ML-based re-training. Methods AVE (Automatisierte Vermessung der Echokardiographie) was based on the echocardiography images of n = 4,965 participants of the population-based Characteristics and Course of Heart Failure Stages A-B and Determinants of Progression Cohort Study. We implemented federated ML: echocardiography images were read by the Academic CoreLab Ultrasound-based Cardiovascular Imaging at the University Hospital Würzburg (UKW). A random algorithm selected 3,226 participants for re-training of the original detector. According to data protection rules, generation of ground truth and ML training cycles took place within the UKW network. Only non-personal training weights were exchanged with the external cooperation partner for refinement of ML algorithms. Results Both the original detector as the re-trained detector were then applied to the echocardiograms of n = 563 participants not used for training. With regards to the human referent the re-trained detector revealed 1) superior accuracy when contrasted with the original detector´s performance as it arrived at significantly smaller mean differences in all but one parameter, and 2) smaller absolute difference between measurements when compared to a group of different human observers. Conclusions Population data based ML in a federated ML set-up was feasible. The re-trained detector exhibited a much lower measurement variability than human readers. This gain in accuracy and precision strengthens the confidence into automated echocardiographic readings, which carries large potential for applications in various settings.
更多查看译文
AI 理解论文
溯源树
样例
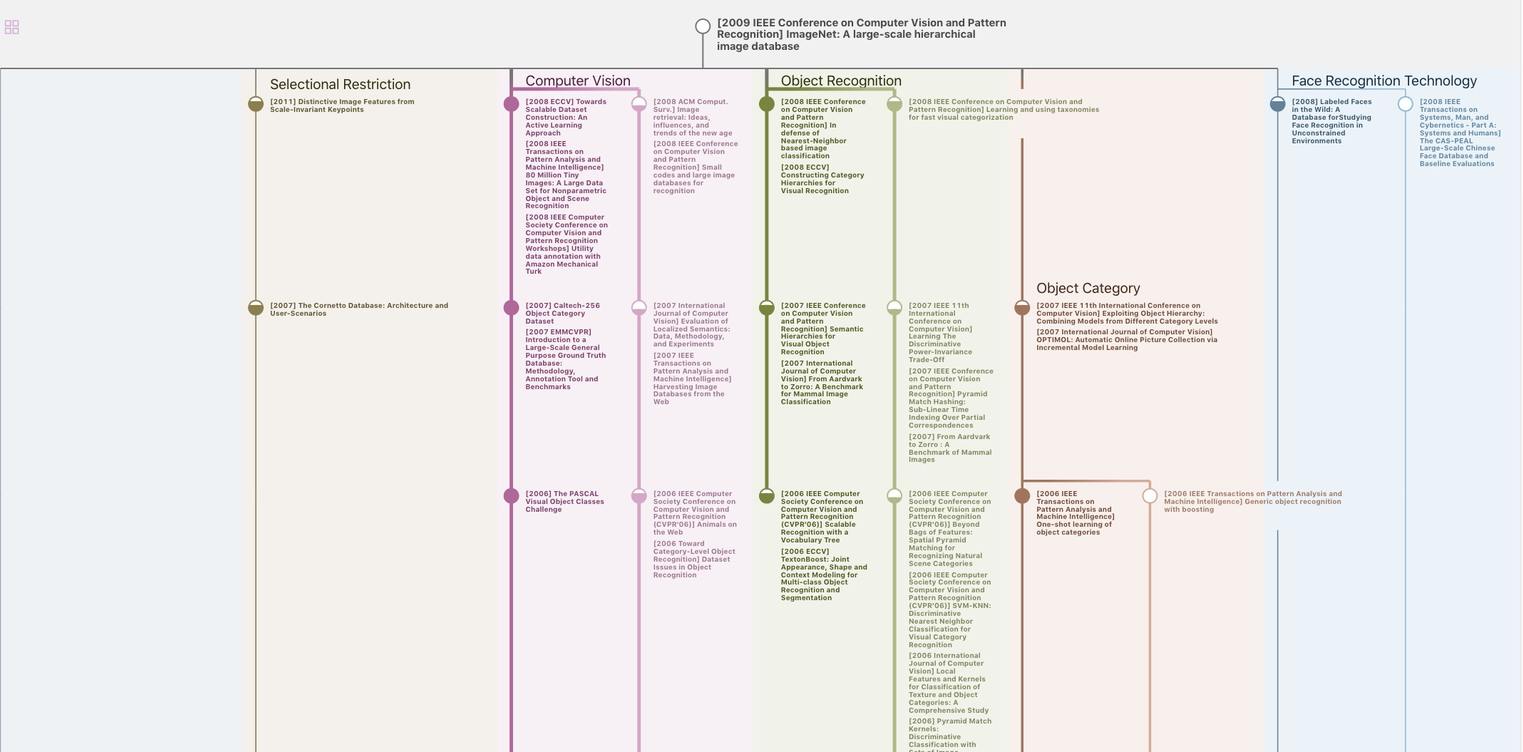
生成溯源树,研究论文发展脉络
Chat Paper
正在生成论文摘要