Machine Learning-Based Control of Electric Vehicle Charging for Practical Distributions Systems With Solar Generation
IEEE Transactions on Smart Grid(2023)
摘要
The adoption of Electric Vehicles (EVs) and solar Photovoltaic (PV) generation by households is rapidly and significantly increasing. Utilities are facing the challenge of efficiently managing EV and PV resources to help mitigate the undesirable effects on grid operation. Existing approaches to solve these issues depend on accurate but hard to predict behavior of EVs and PVs, detailed knowledge of customers, and grid infrastructure, all of which complicate the effective deployment of these resources. Motivated by these practical challenges and in collaboration with industry partners working on addressing these issues, this paper proposes a two-level data-driven smart controller for EV charging in distribution systems. The controller is modeled as a Deep Reinforcement Learning (DRL) agent, which coordinates the charging rates of multiple EVs connected to a realistic residential feeder with high penetration of PV generation. The first level coordinates the aggregated EV load at distribution Medium Voltage (MV) level to provide Demand Response (DR) services; at the Low Voltage (LV) level it aims to maximize the EVs’ state of charge at departure while avoiding the overloading of the MV/LV distribution transformers. The controller is verified through simulations on an actual utility grid facing the aforementioned challenges, demonstrating the effectiveness and practicality of the proposed DRL-based smart charging approach.
更多查看译文
关键词
Deep deterministic policy gradient,deep reinforcement learning,demand response,distribution system,electric vehicle,machine learning,photovoltaic
AI 理解论文
溯源树
样例
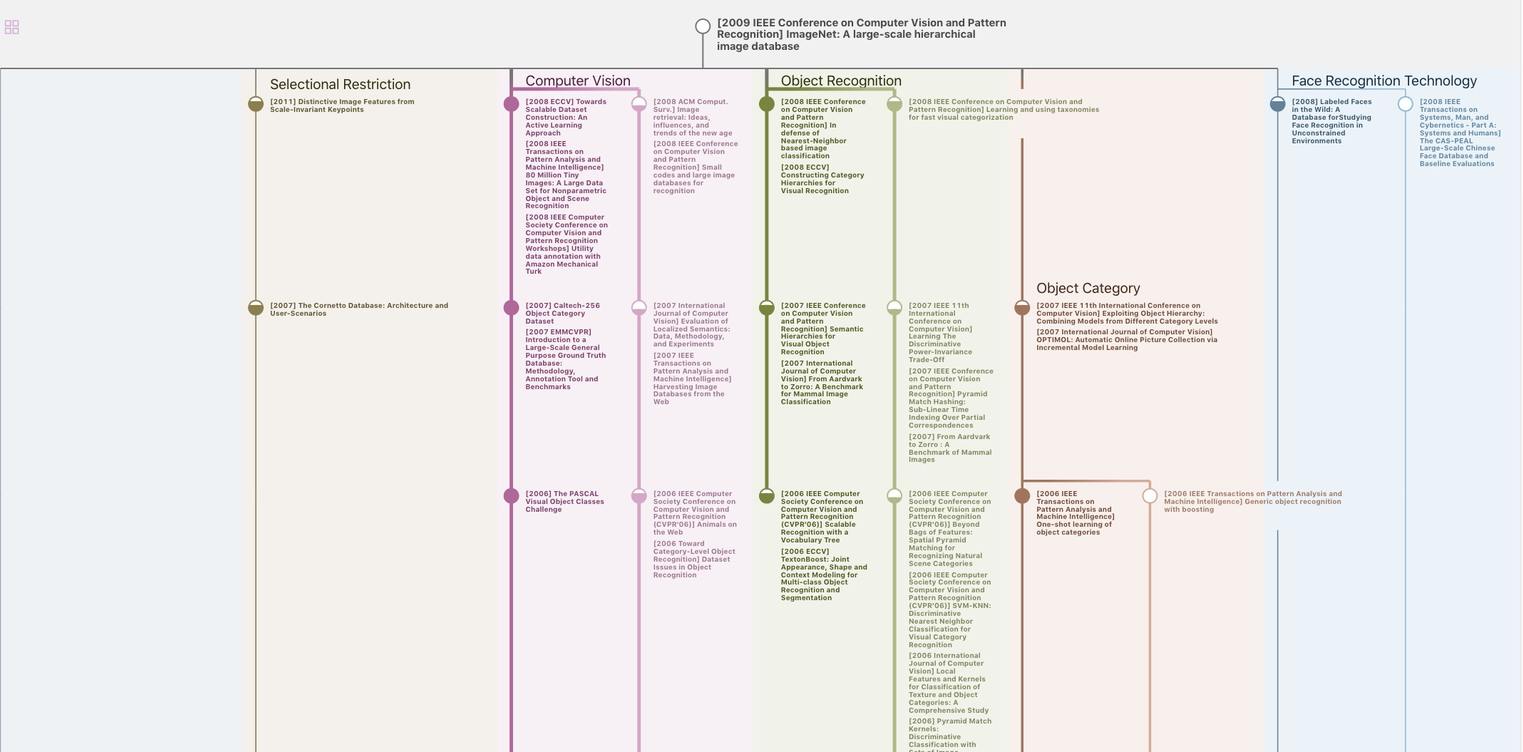
生成溯源树,研究论文发展脉络
Chat Paper
正在生成论文摘要