A Subgraph-Based Hierarchical Q-Learning Approach to Optimal Resource Scheduling for Complex Industrial Networks
IEEE TRANSACTIONS ON CIRCUITS AND SYSTEMS I-REGULAR PAPERS(2023)
摘要
This paper proposes a subgraph-based hierarchical Q-learning network (SgHQN) approach to solve the optimal resource scheduling problem for complex industrial networks. In the industrial network, each connection between two individual stations has limited communication bandwidth, while each station has limited computing and storage capability and is only accessible to its local information. The resource packages flowing within the industrial network are treated as agents that have different sizes and different levels of decision-making priority. This makes the industrial resource scheduling problem on the industrial network a multi-level decision-making problem with information asymmetry. Specifically, the resource packages with lower decision-making priority have knowledge of the decisions made by those with higher priority, but not vice versa. To solve this resource scheduling problem with information asymmetry, an SgHQN model is developed by exploiting partial observations. It is found that the proposed SgHQN can be used to solve resource scheduling problems for general industrial networks. Numerical experiments simulating industrial scheduling scenarios demonstrate the effectiveness and advantages of our method.
更多查看译文
关键词
Job shop scheduling,Decision making,Q-learning,Task analysis,Optimization,Games,Processor scheduling,Complex industrial networks,deep Q-learning,information asymmetry,resource scheduling
AI 理解论文
溯源树
样例
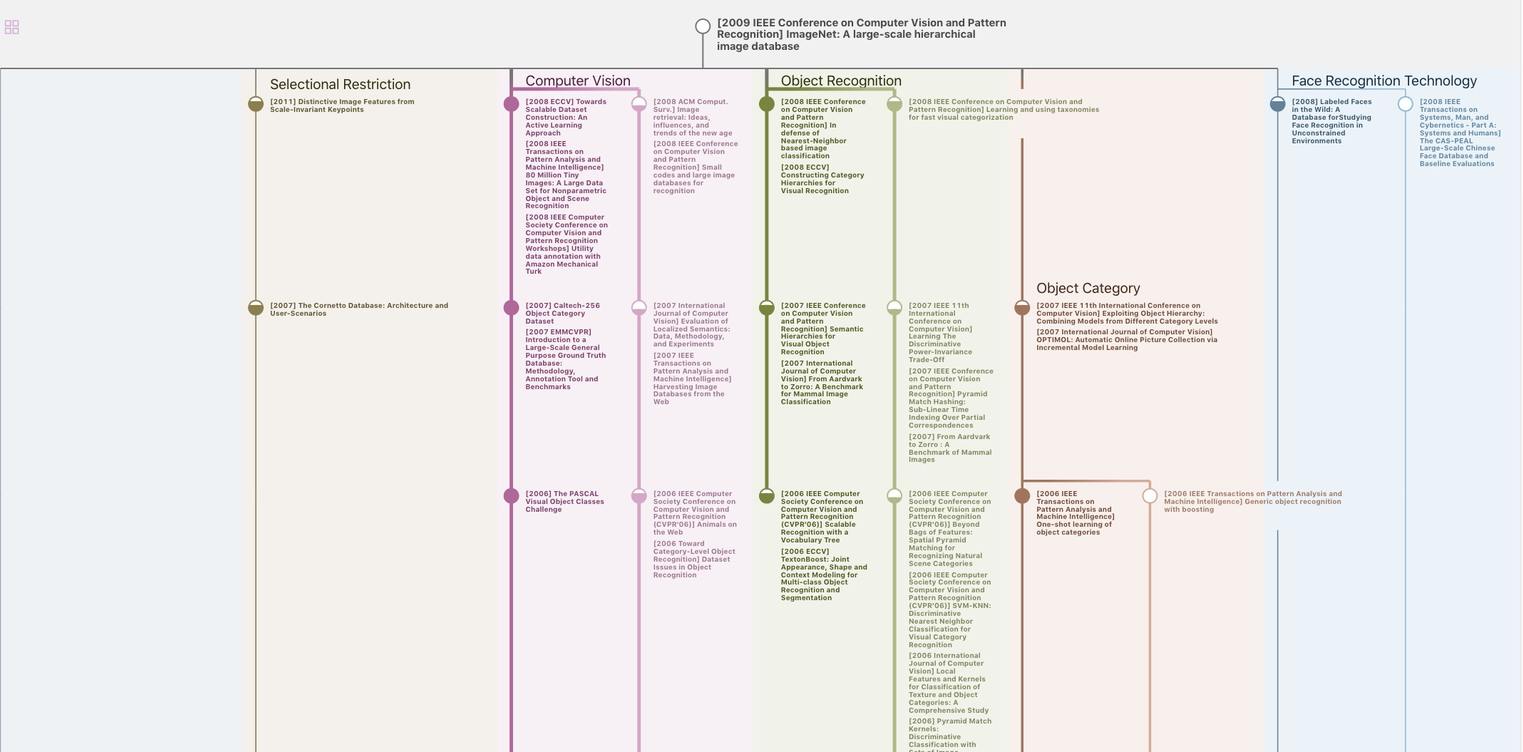
生成溯源树,研究论文发展脉络
Chat Paper
正在生成论文摘要