Coreset Learning-Based Sparse Black-Box Adversarial Attack for Video Recognition
IEEE TRANSACTIONS ON INFORMATION FORENSICS AND SECURITY(2024)
摘要
In recent years, researchers have explored the use of sparse black-box video adversarial attacks, which involve selecting keyframes to reduce computational complexity and improve efficiency in generating perturbations. However, the current sparse strategy is not optimized for attack and detection steps, resulting in inaccurate frame selection. Some researchers have used reinforcement learning to train an agent to select keyframes, but this method requires additional training. To address these challenges, we propose a plug-and-play black-box sparse attack algorithm called CLVA based on the coreset concept of active learning. Our algorithm treats a video as a mini-dataset and employs the K-Center-Greedy algorithm to compute the distances between frames. We then select the frame that meets the distance condition as the key frame. We conducted extensive experiments using two attack algorithms on five mainstream recognition models and three video recognition datasets. Our results demonstrate that CLVA significantly accelerates the black-box video attack algorithm while achieving state-of-the-art performance in sparsity, time, and success rate compared to recent sparse attack algorithms.
更多查看译文
关键词
Adversarial attack,video recognition,sparse attack,active learning,coreset learning
AI 理解论文
溯源树
样例
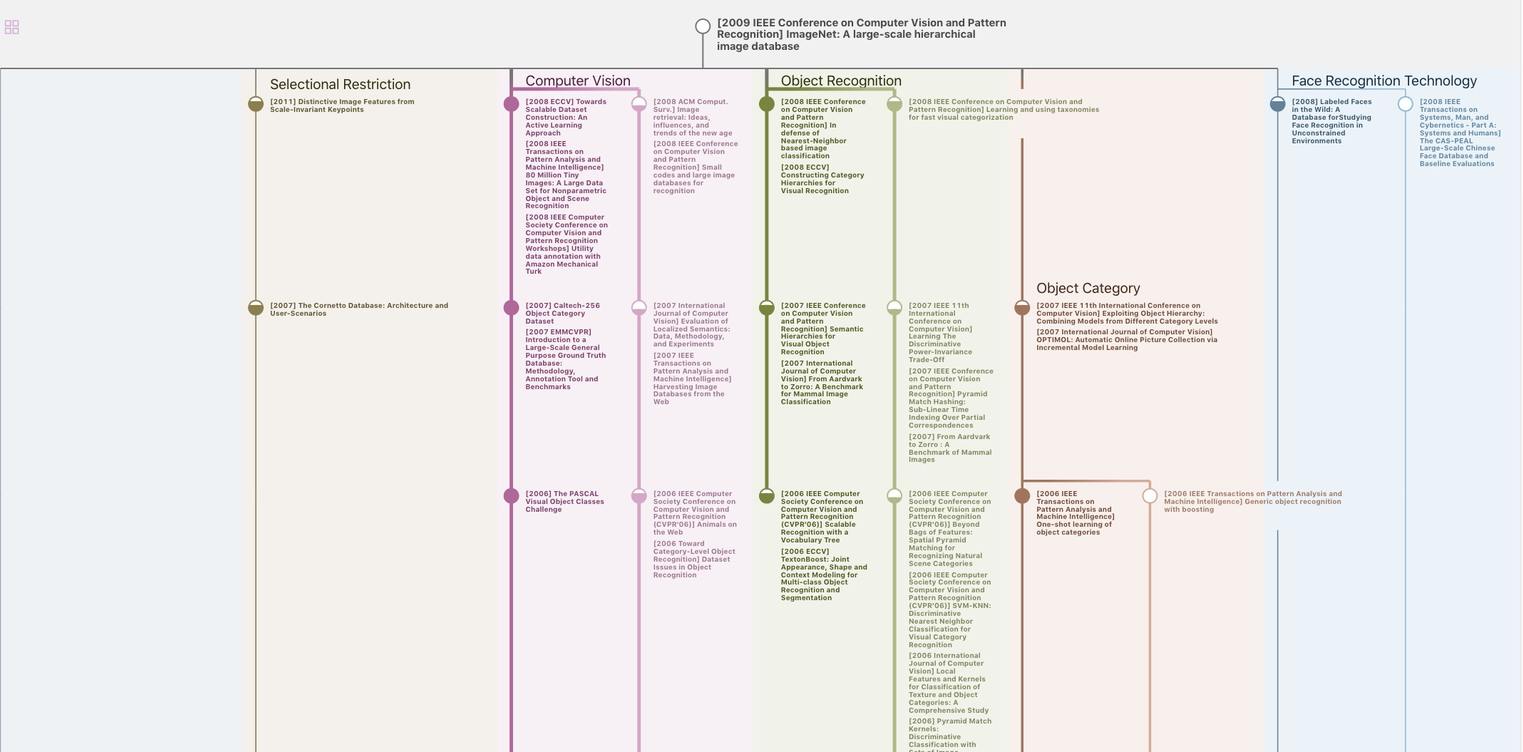
生成溯源树,研究论文发展脉络
Chat Paper
正在生成论文摘要