A Novel Small-Signal Ferroelectric Capacitance based Content Addressable Memory for Area-and Energy-Efficient Lifelong Learning
IEEE Electron Device Letters(2023)
摘要
In this work, for the first time, a novel energy- and area-efficient ferroelectric (FE) small-signal capacitance based content addressable memory (CAM) design is proposed and experimentally demonstrated. It can accurately perform distance metric in high linearity for feature storage and retrieval of memory-augmented neural network (MANN). By utilizing the non-monotonic FE small-signal capacitance, the linearly non-separable comparison operation of CAM can be experimentally implemented in only one FE capacitor, without the need of twin complementary branches, along with the benefits of high endurance (>10 9 cycles), good retention (10 years) and excellent consistency. Furthermore, based on the proposed searching method by accumulating the charge in search phase of CAM cells, the experimental results show that the CAM architecture based on FE capacitive crossbar array can perform distance metric with ideally high linearity. Array-level HSPICE simulation further shows that the search energy consumption of proposed CAM design is the lowest compared with the other emerging memories based CAM designs. Based on the proposed design, high-accuracy (96%) of MANN based few-shot learning is demonstrated, providing a promising approach for energy-efficient lifelong learning at the edge.
更多查看译文
AI 理解论文
溯源树
样例
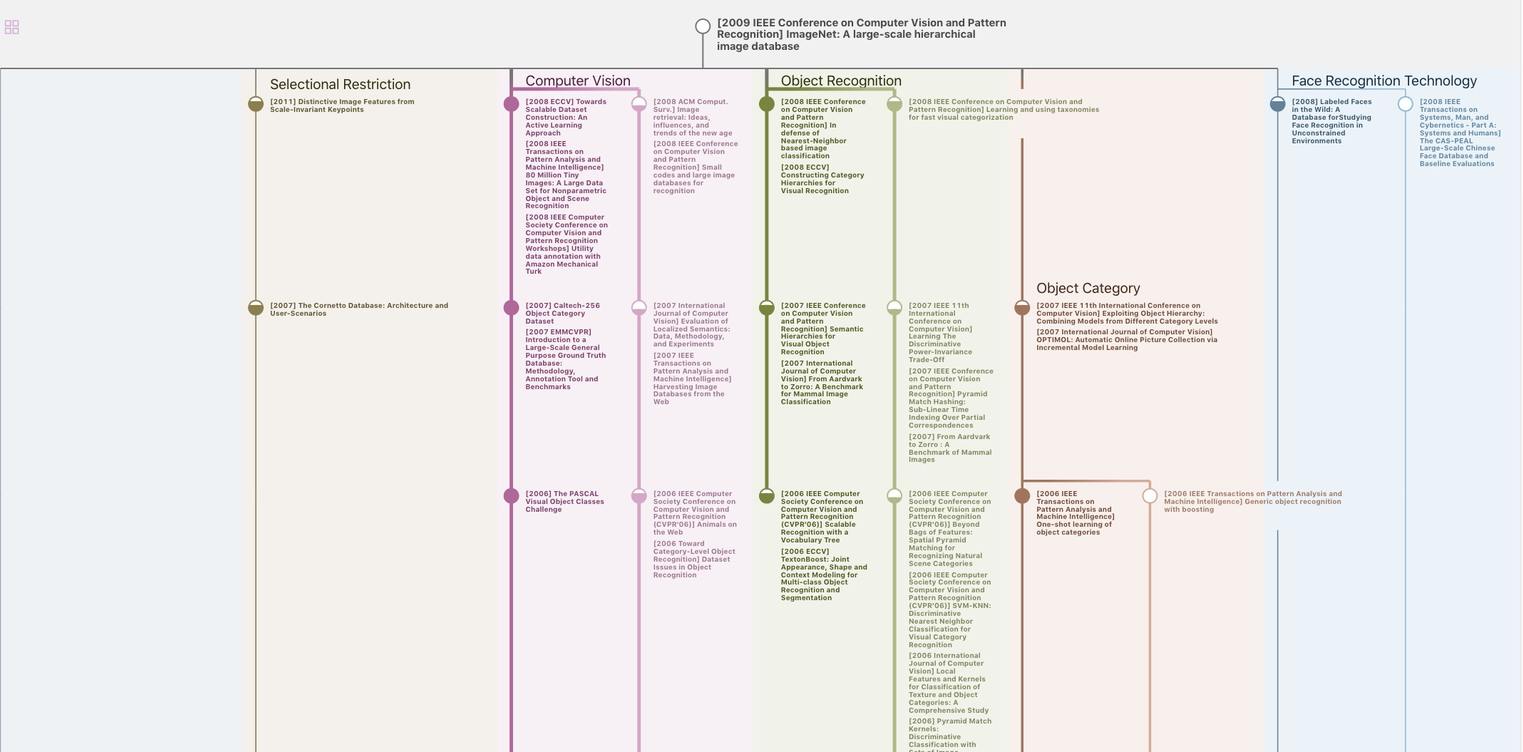
生成溯源树,研究论文发展脉络
Chat Paper
正在生成论文摘要