EqBal-RS: Mitigating popularity bias in recommender systems
Journal of Intelligent Information Systems(2023)
摘要
Recommender systems are deployed heavily by many online platforms for better user engagement and providing recommendations. Despite being so popular, several works have shown the existence of popularity bias due to the non-random nature of missing data. Popularity bias leads to the recommendation of only a few popular items causing starvation of many non-popular items. This paper considers an easy-to-understand metric to evaluate the popularity bias as the difference between mean squared error on popular and non-popular items. Then, we propose EqBal-RS, a novel re-weighting technique that updates the weights of popular and non-popular items. Re-weighting ensures that both item sets are equally balanced during training using a trade-off function between overall loss and popularity bias. Our experiments on real-world datasets show that EqBal-RS outperforms the existing state-of-art algorithms in terms of accuracy, quality, and fairness. EqBal-RS works well on the proposed and existing popularity bias metrics and has significantly reduced runtime. The code is publicly available at https://github.com/eqbalrs/EqBalRS
更多查看译文
关键词
Recommender system,Matrix factorization,Popularity bias,Fairness
AI 理解论文
溯源树
样例
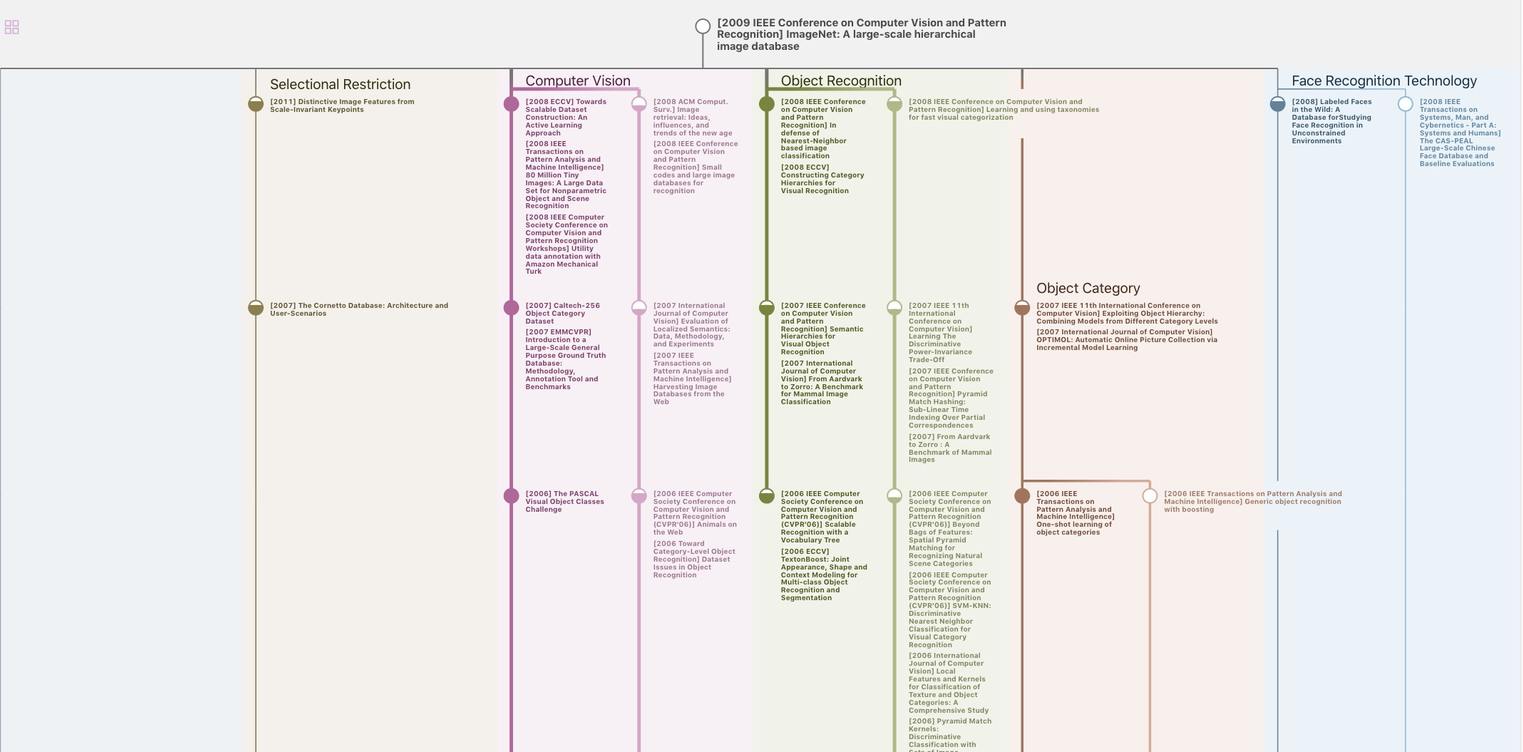
生成溯源树,研究论文发展脉络
Chat Paper
正在生成论文摘要