LOTUS: Continual Imitation Learning for Robot Manipulation Through Unsupervised Skill Discovery
arXiv (Cornell University)(2023)
摘要
We introduce LOTUS, a continual imitation learning algorithm that empowers a physical robot to continuously and efficiently learn to solve new manipulation tasks throughout its lifespan. The core idea behind LOTUS is constructing an ever-growing skill library from a sequence of new tasks with a small number of human demonstrations. LOTUS starts with a continual skill discovery process using an open-vocabulary vision model, which extracts skills as recurring patterns presented in unsegmented demonstrations. Continual skill discovery updates existing skills to avoid catastrophic forgetting of previous tasks and adds new skills to solve novel tasks. LOTUS trains a meta-controller that flexibly composes various skills to tackle vision-based manipulation tasks in the lifelong learning process. Our comprehensive experiments show that LOTUS outperforms state-of-the-art baselines by over 11% in success rate, showing its superior knowledge transfer ability compared to prior methods. More results and videos can be found on the project website: https://ut-austin-rpl.github.io/Lotus/.
更多查看译文
关键词
continual imitation learning,robot manipulation through
AI 理解论文
溯源树
样例
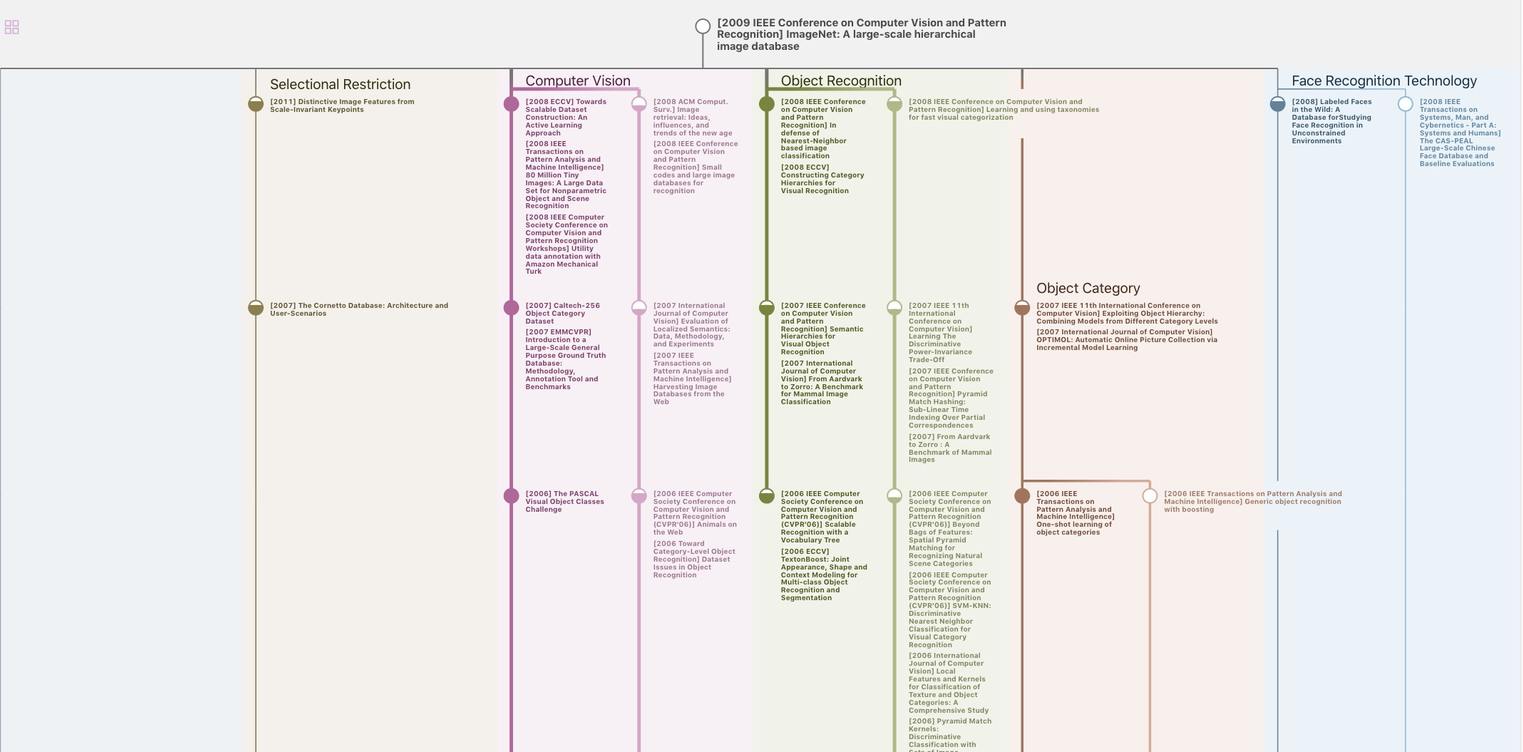
生成溯源树,研究论文发展脉络
Chat Paper
正在生成论文摘要