Sparsity-Oriented MRAM-Centric Computing for Efficient Neural Network Inference
IEEE TRANSACTIONS ON EMERGING TOPICS IN COMPUTING(2024)
摘要
Near-memory computing (NMC) and in- memory computing (IMC) paradigms show great importance in non-von Neumann architecture. Spin-transfer torque magnetic random access memory (STT-MRAM) is considered as a promising candidate to realize both NMC and IMC for resource-constrained applications. In this work, two MRAM-centric computing frameworks are proposed: triple-skipping NMC (TS-NMC) and analog-multi-bit-sparsity IMC (AMS-IMC). The TS-NMC exploits the sparsity of activations and weights to implement a write-read-calculation triple skipping computing scheme by utilizing a sparse flag generator. The AMS-IMC with reconfigured computing bit-cell and flag generator accommodate bit-level activation sparsity in the computing. STT-MRAM array and its peripheral circuits are implemented with an industrial 28-nm CMOS design-kit and an MTJ compact model. The triple-skipping scheme can reduce memory access energy consumption by 51.5x when processing zero vectors, compared to processing non-zero vectors. The energy efficiency of AMS-IMC is improved by 5.9x and 1.5x (with 75% input sparsity) as compared to the conventional NMC framework and existing analog IMC framework. Verification results show that TS-NMC and AMS-IMC achieved 98.6% and 97.5% inference accuracy in MNIST classification, with energy consumption of 14.2 nJ/pattern and 12.7 nJ/pattern, respectively.
更多查看译文
关键词
In-memory computing,near-memory computing,neural network,STT-MRAM,sparsity vector and matrix
AI 理解论文
溯源树
样例
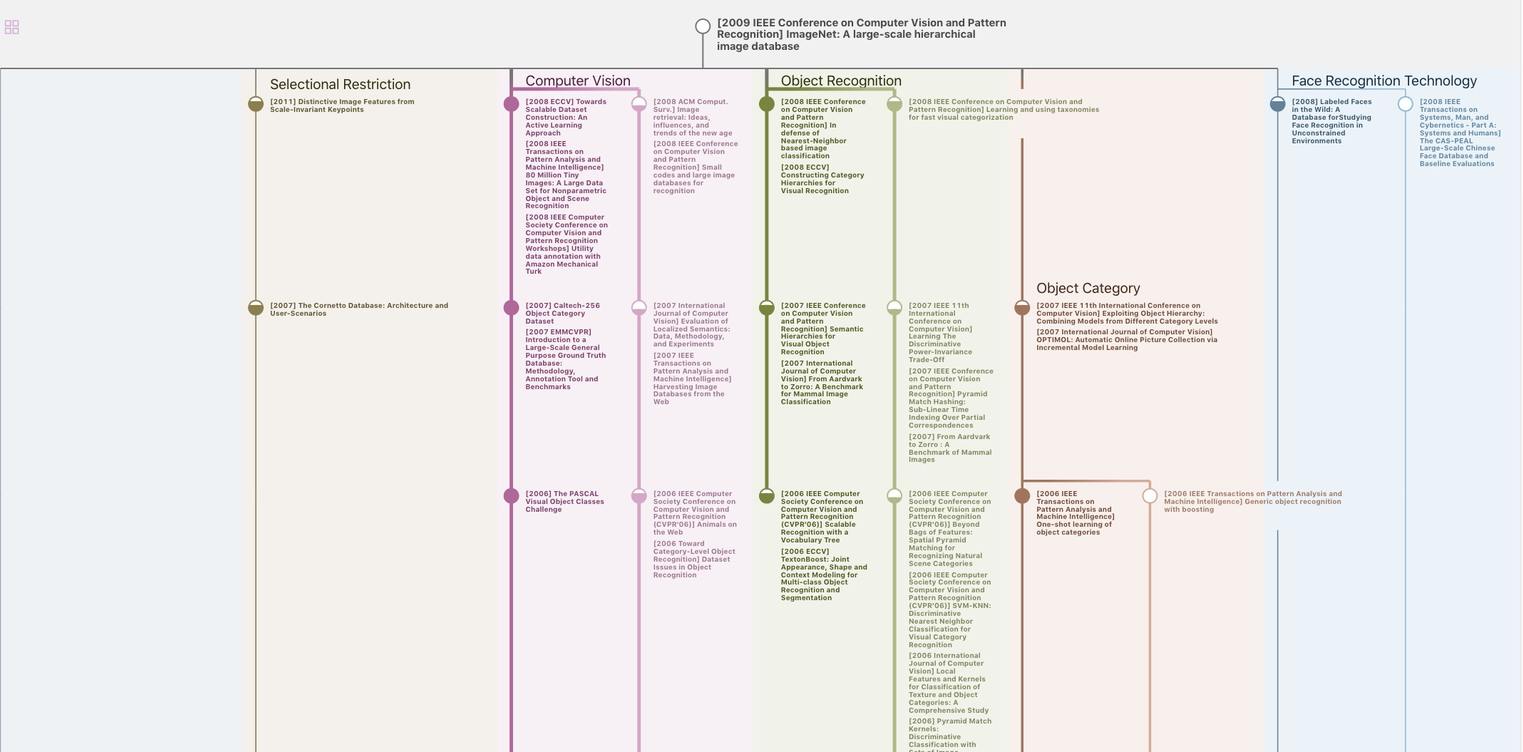
生成溯源树,研究论文发展脉络
Chat Paper
正在生成论文摘要