Label-Weighted Graph-Based Learning for Semi-Supervised Classification Under Label Noise
IEEE TRANSACTIONS ON BIG DATA(2024)
摘要
Graph-based semi-supervised learning (GSSL) is a quite important technology due to its effectiveness in practice. Existing GSSL works often treat the given labels equally and ignore the unbalance importance of labels. In some inaccurate systems, the collected labels usually contain noise (noisy labels) and the methods treating labels equally suffer from the label noise. In this article, we propose a novel label-weighted learning method on graph for semi-supervised classification under label noise, which allows considering the contribution differences of labels. In particular, the label dependency of data is revealed by graph constraints. With the help of this label dependency, the proposed method develops the strategy of adaptive label weight, where label weights are assigned to labels adaptively. Accordingly, an efficient algorithm is developed to solve the proposed optimization objective, where each subproblem has a closed-form solution. Experimental results on a synthetic dataset and several real-world datasets show the advantage of the proposed method, compared to the state-of-the-art methods.
更多查看译文
关键词
Noise measurement,Synthetic data,Robustness,Big Data,Adaptation models,Optimization,Deep learning,Graph-based learning,semi-supervised classification,label noise,adaptive label weight
AI 理解论文
溯源树
样例
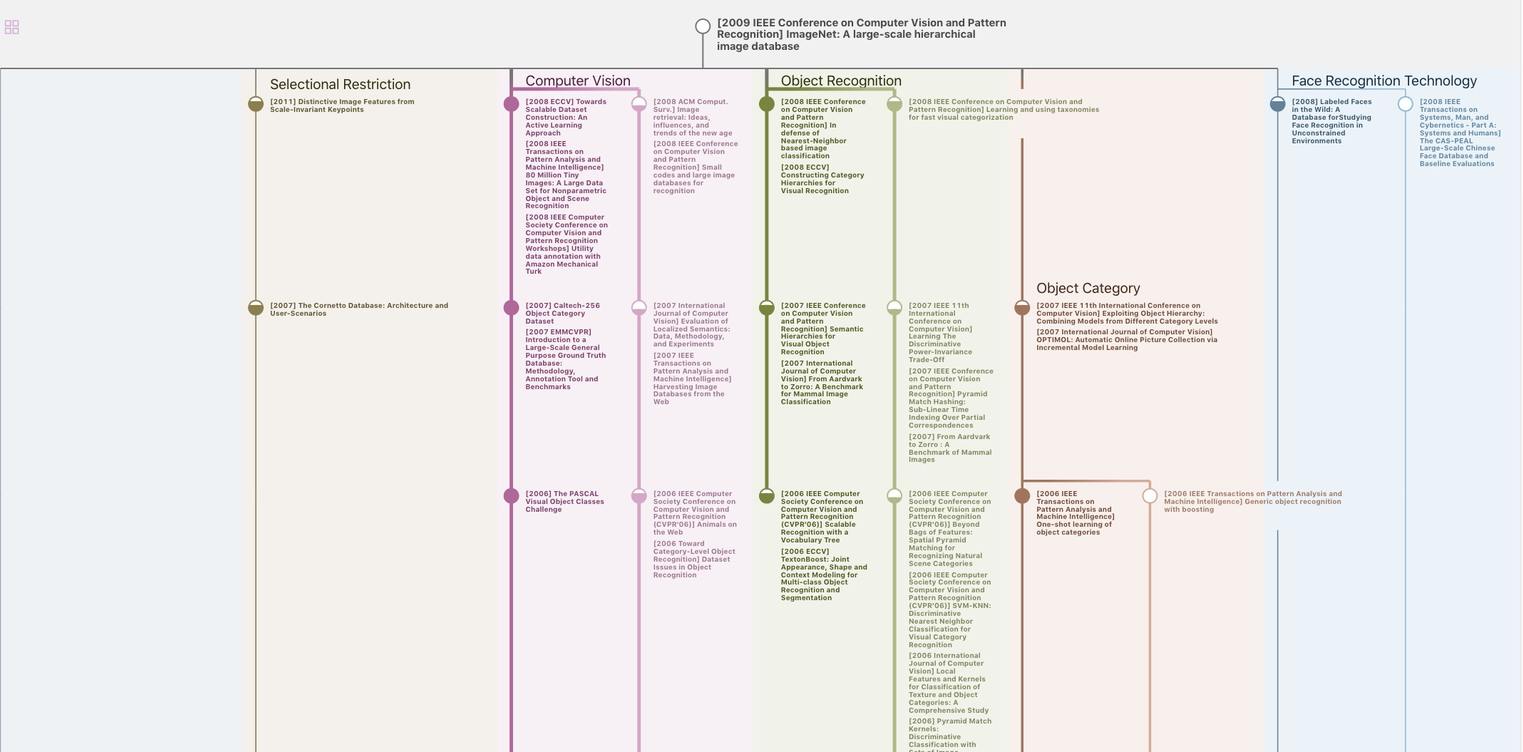
生成溯源树,研究论文发展脉络
Chat Paper
正在生成论文摘要