Geospatial Tessellation in the Agent-In-Cell Model: A Framework for Agent-Based Modeling of Pandemic
arXiv (Cornell University)(2023)
摘要
Agent-based simulation, a powerful tool for analyzing complex systems, faces challenges when integrating geographic elements due to increased computational demands. This study introduces a series of 'agent-in-the-cell' Agent-Based Models to simulate COVID spread in a city, utilizing geographical features and real-world mobility data from Safegraph. We depart from traditional aggregated transmission probabilities, focusing on direct person-to-person contact probabilities, informed by physics-based transmission studies. Our approach addresses computational complexities through innovative strategies. Agents, termed 'meta-agents', are linked to specific home cells in a city's tessellation. We explore various tessellations and agent densities, finding that Voronoi Diagram tessellations, based on specific street network locations, outperform Census Block Group tessellations in preserving dynamics. Additionally, a hybrid tessellation combining Voronoi Diagrams and Census Block Groups proves effective with fewer meta-agents, maintaining an accurate representation of city dynamics. Our analysis covers diverse city sizes in the U.S., offering insights into agent count reduction effects, sensitivity metrics, and city-specific factors. We benchmark our model against an existing ABM, focusing on runtime and reduced agent count implications. Key optimizations include meta-agent usage, advanced tessellation methods, and parallelization techniques. This study's findings contribute to the field of agent-based modeling, especially in scenarios requiring geographic specificity and high computational efficiency.
更多查看译文
关键词
agent-in-cell agent-based modeling
AI 理解论文
溯源树
样例
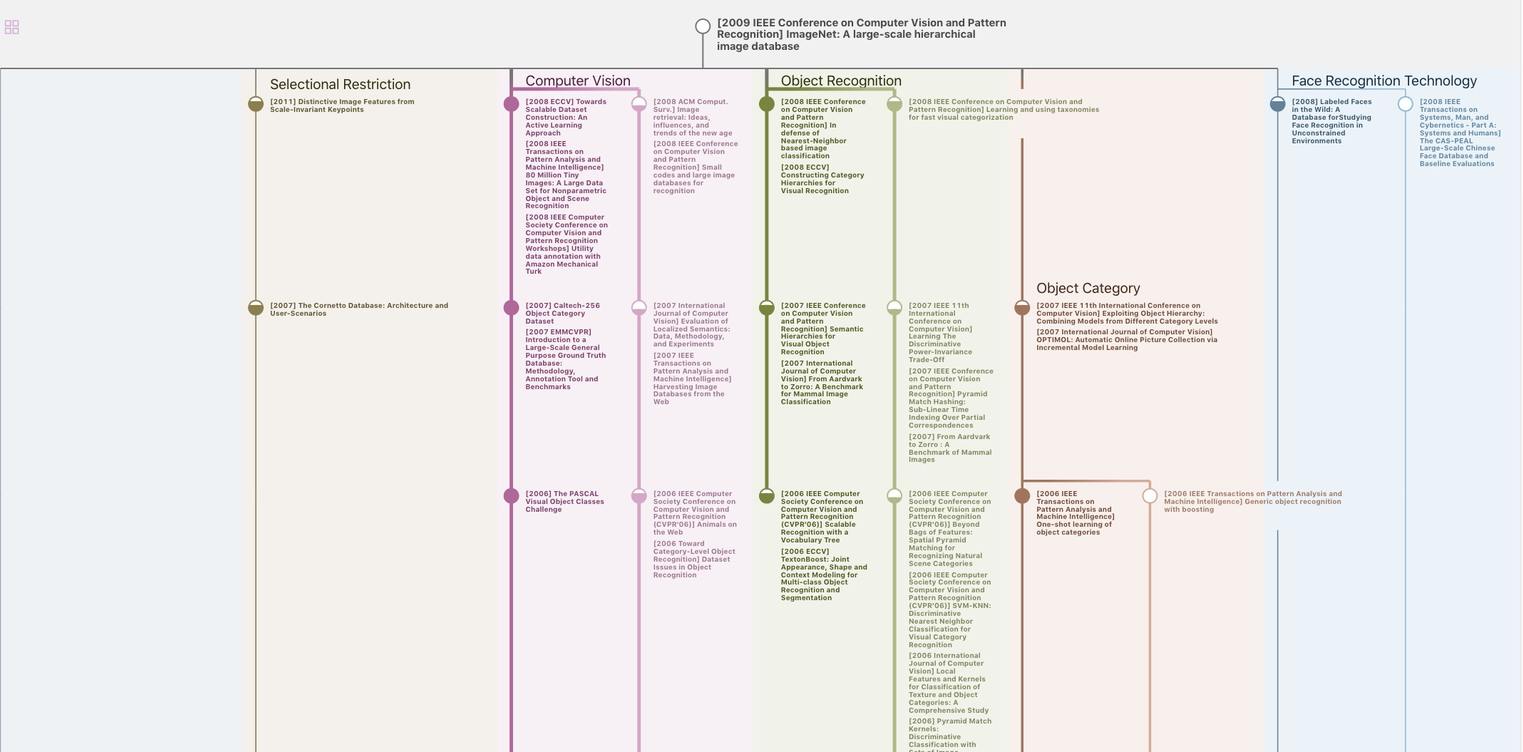
生成溯源树,研究论文发展脉络
Chat Paper
正在生成论文摘要