Tight Bounds for Machine Unlearning via Differential Privacy
arXiv (Cornell University)(2023)
摘要
We consider the formulation of "machine unlearning" of Sekhari, Acharya, Kamath, and Suresh (NeurIPS 2021), which formalizes the so-called "right to be forgotten" by requiring that a trained model, upon request, should be able to "unlearn" a number of points from the training data, as if they had never been included in the first place. Sekhari et al. established some positive and negative results about the number of data points that can be successfully unlearnt by a trained model without impacting the model's accuracy (the "deletion capacity"), showing that machine unlearning could be achieved by using differentially private (DP) algorithms. However, their results left open a gap between upper and lower bounds on the deletion capacity of these algorithms: our work fully closes this gap, obtaining tight bounds on the deletion capacity achievable by DP-based machine unlearning algorithms.
更多查看译文
关键词
privacy,machine unlearning,tight bounds
AI 理解论文
溯源树
样例
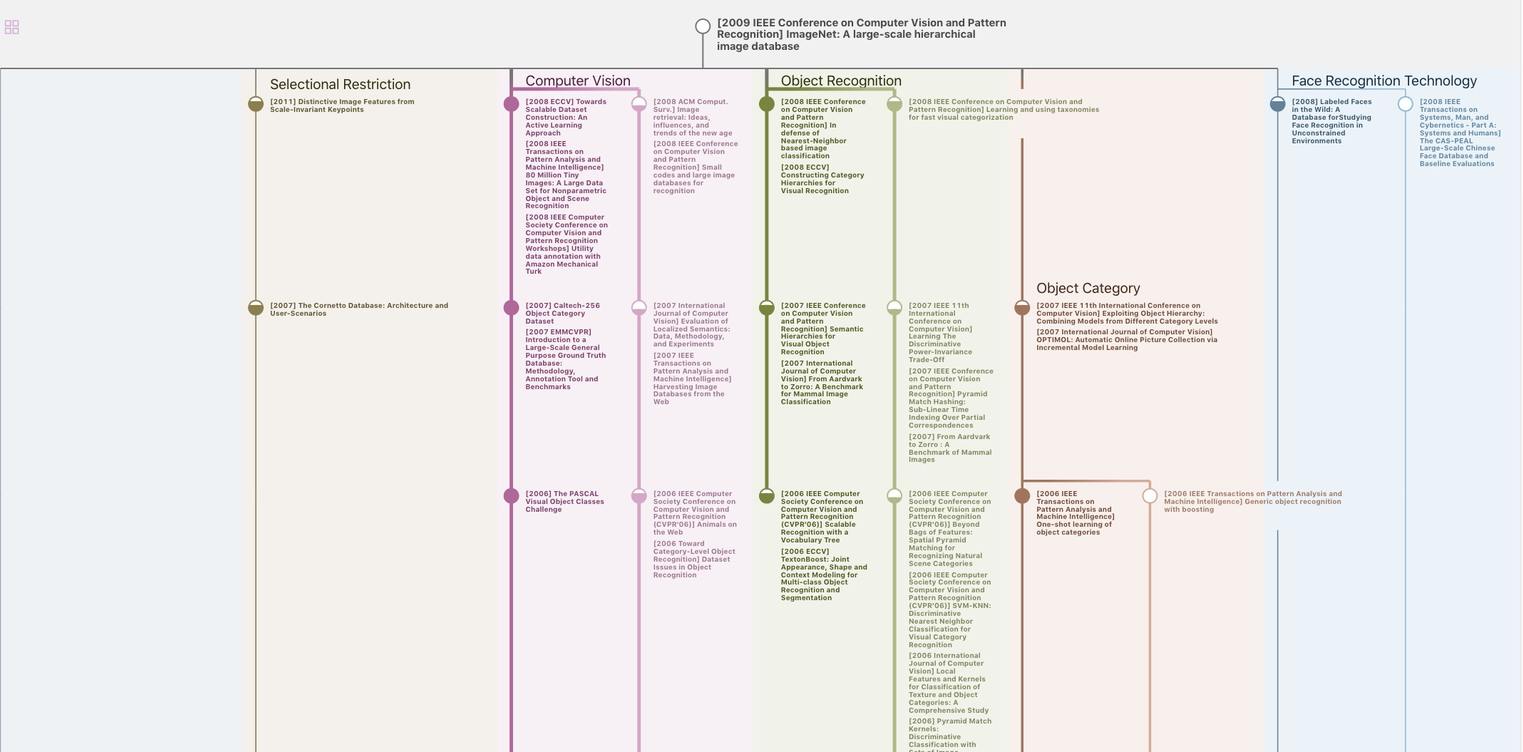
生成溯源树,研究论文发展脉络
Chat Paper
正在生成论文摘要