Machine learning-based prediction and interpretation of decomposition temperatures of energetic materials
ENERGETIC MATERIALS FRONTIERS(2023)
摘要
Exploring the application of machine learning (ML) in energetic materials (EMs) has been a hot research topic. Accordingly, the prediction of the detonation properties of EMs using ML methods has attracted much attention. However, the predictive models for the thermal decomposition temperatures (T-d) of EMs have been scarcely reported. Furthermore, the small datasets used in these reports lead to a weak generalization ability of the predictive models. This study created a dataset containing 1022 energetic molecules with T-d values of 38-425 degrees C and determined an optimal predictive model through training. The gradient boost machine for regression (GBR) model yielded a coefficient of determination (R-2) of 0.65 and a mean absolute error (MAE) of 27.7 for the test set. This study further explored critical features, determining that the prediction accuracy of the models was significantly influenced by descriptors representing molecular bond stability (i.e., the BCUT metrics) and atomic composition (i.e., the Molecular ID). Finally, the analysis of the outlier structure indicated that the model accuracy can be further improved by incorporating features related to molecular interactions. The results of this study help gain a deep understanding of the application of ML in the prediction of EM properties, particularly in dataset construction and feature selection.
更多查看译文
关键词
Machine learning,Energetic materials,Property prediction,Decomposition temperature
AI 理解论文
溯源树
样例
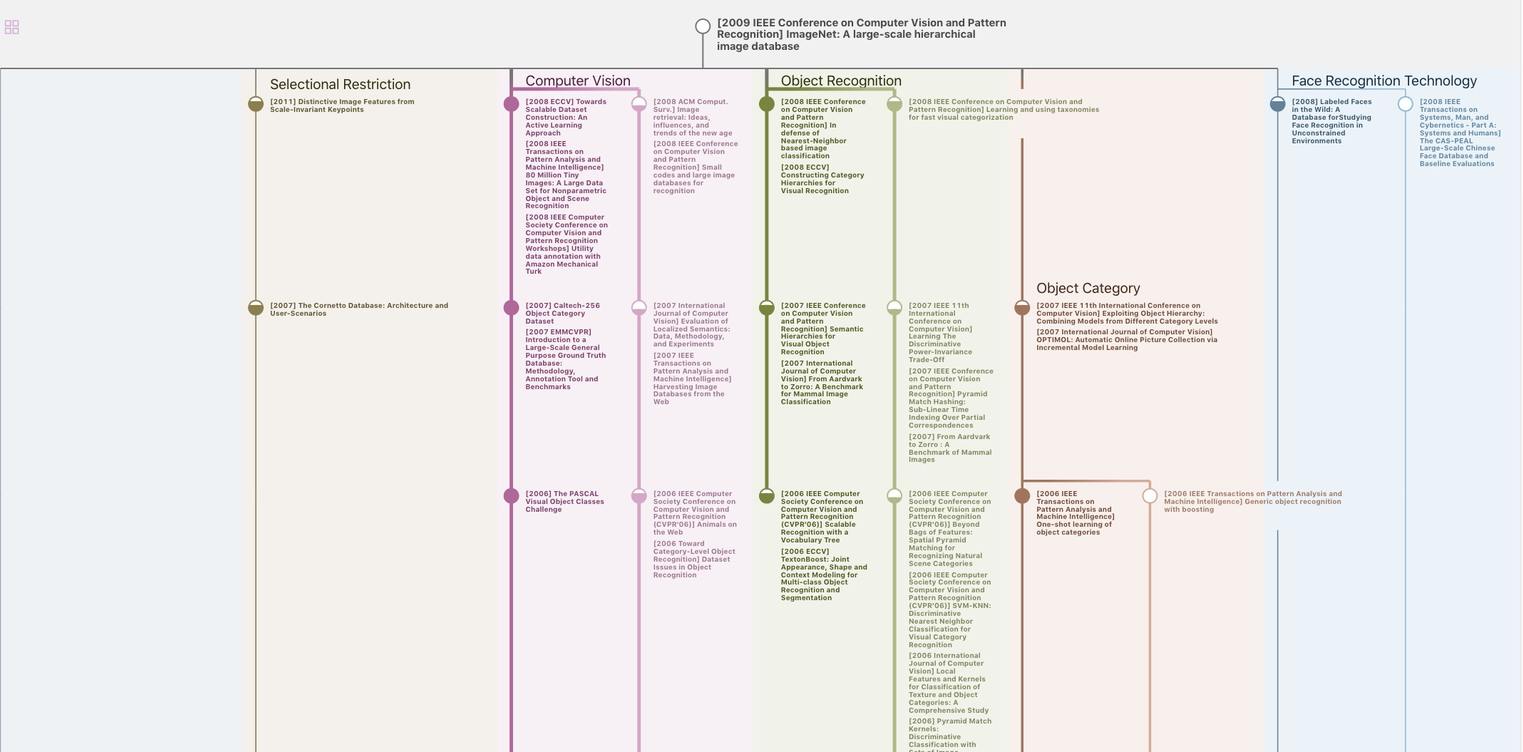
生成溯源树,研究论文发展脉络
Chat Paper
正在生成论文摘要