Hybrid Learning: When Centralized Learning Meets Federated Learning in the Mobile Edge Computing Systems
IEEE TRANSACTIONS ON COMMUNICATIONS(2023)
摘要
Federated learning is a new artificial intelligence technology with which an edge server can orchestrate with multiple end users to train a global model collaboratively. Under this setting, users only upload the locally trained parameters instead of their local data, substantially reducing communication costs and boosting data privacy. Nonetheless, federated learning mainly relies on users' local training, overlooking the abundant computing resources owned by the edge server. To exploit the edge server's processing power, we propose a hybrid learning paradigm that consists of centralized and federated learning components. This scheme uploads a portion of users' data for centralized learning when the local model is trained under federated learning. We derive a theoretical upper bound for the model accuracy, which can be used to assess the performance of the proposed new learning paradigm. To balance the computation and communication resources for a good model accuracy performance, we establish a joint optimization problem of model accuracy, latency, and energy consumption. We also devise the corresponding joint optimization algorithm to solve the problem. Experiment results show that compared with centralized and federated learning, the proposed hybrid learning algorithm can effectively improve the model accuracy and significantly reduce computation and communication resources.
更多查看译文
关键词
Federated learning,hybrid learning,edge computing,resource allocation,optimization design
AI 理解论文
溯源树
样例
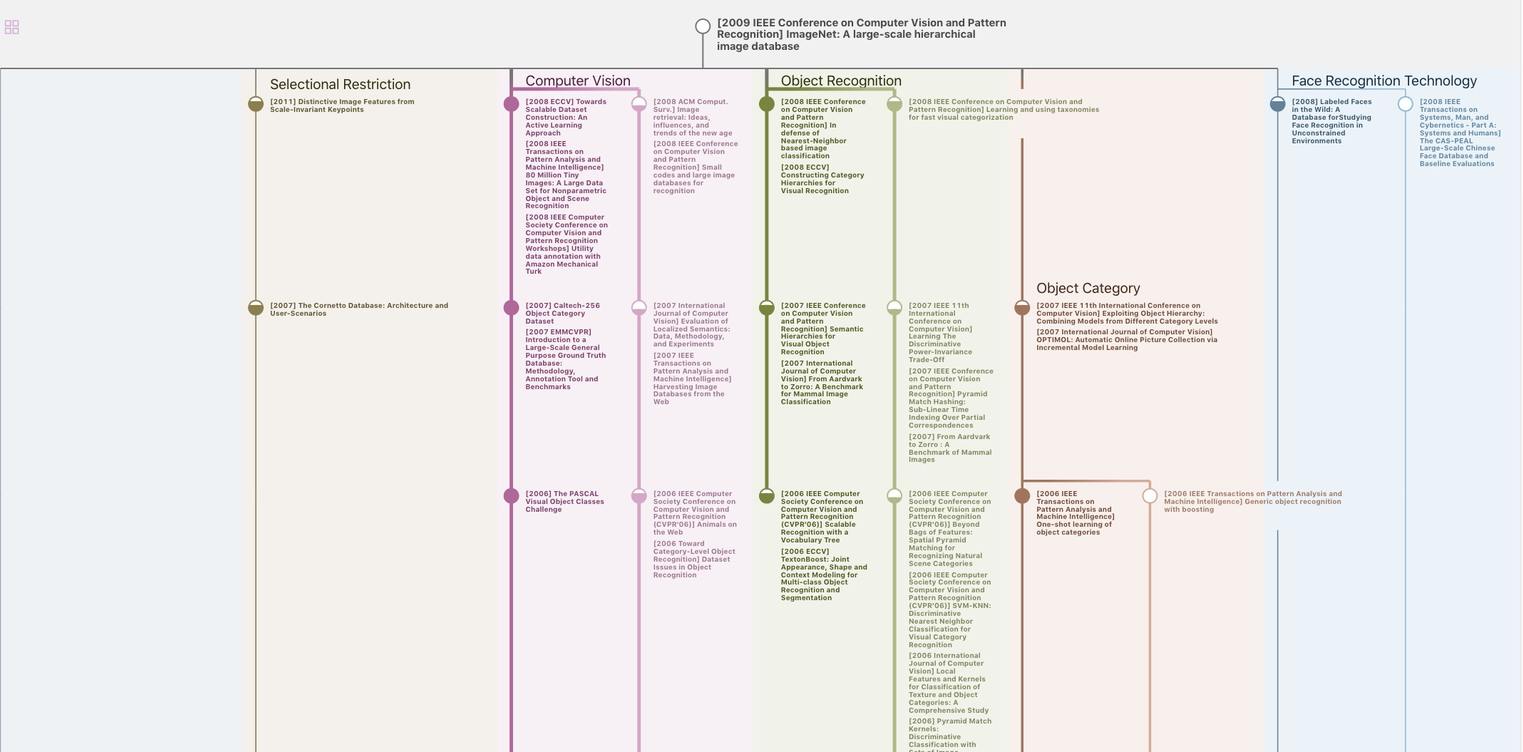
生成溯源树,研究论文发展脉络
Chat Paper
正在生成论文摘要